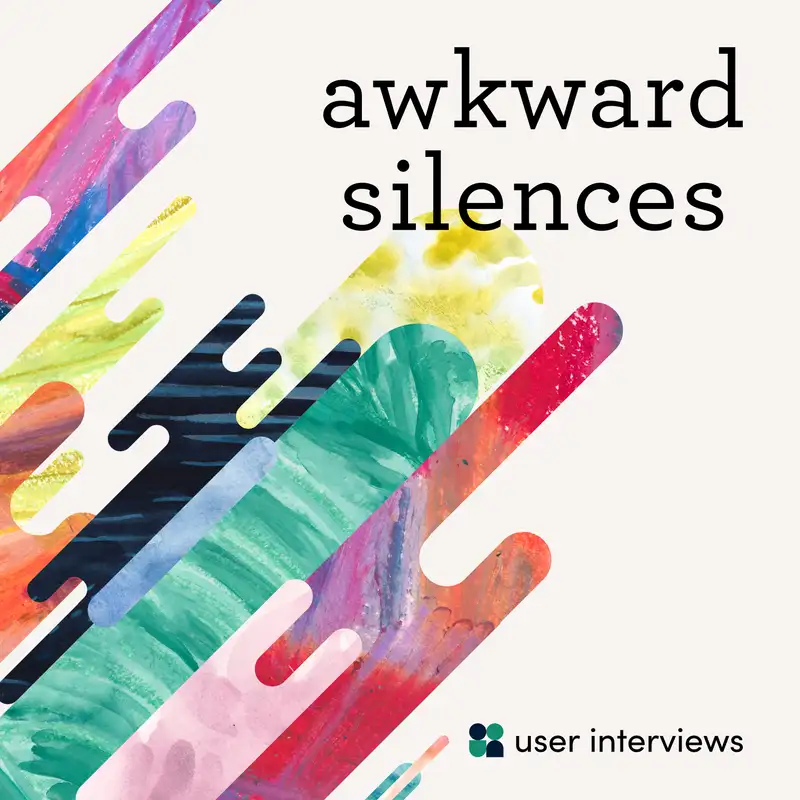
#123 - The Power of Atomic Research with Daniel Pidcock of Glean.ly
Erin - 00:00:13: This is Erin May.
JH - 00:00:15: I'm John-Henry Forster. And this is Awkward Silences.
Erin - 00:00:26: Hello everybody, and welcome back to Awkward Silences. Today we're here with Daniel Pidcock, who is the Co-founder of Glean.ly and the creator of Atomic Research. Really excited to have you here today to talk about, of course, atomic research and where it came from and where it is now. JH is here, too.
JH - 00:00:46: Yeah, excited for this one. I feel like one of the first concepts I got exposed to when I was doing kind of repository stuff was Atomic Research a couple of years back. And excited to explore it.
Daniel - 00:00:55: Thank you so much, and I'm really honored to be here. So thank you so much for inviting me. And I've been listening to a lot of the podcasts, and there are some real favorites in there. So enjoying it.
Erin - 00:01:04: Yeah. Thanks so much for joining. It's actually funny you mentioned that, JH. When I joined Q4 2017 User Interviews just a few months after JH joined, one of the first things you shared with me, JH, was this article on atomic research all those years ago. So cool to have you on now and to dig into it.
Daniel - 00:01:24: Fantastic. Thank you.
Erin - 00:01:25: So I imagine we have some listeners who are pretty familiar with atomic research, and others who maybe are not at all familiar. So let's start with the beginning. What is atomic research?
Daniel - 00:01:35: Well, it's really simple. It's basically the process of taking a piece of knowledge and breaking it down into its atomic parts. So we define as four distinct parts: the experiment, which is the source of the learning, wherever it came from. And experiment sounds a bit formal. It doesn't need to be in a laboratory or anything like that. It could even just be something you heard on the bus, but it's where the information, the data came from. And then the next one is a fact. So that's what we learned as factually as possible. And the reason we call it a fact rather than the finding is just to remind people that it's not our opinion of what we've seen or what we've heard or what the data says. It's what the data says or what the person has said if we're interviewing someone. Whereas the next item, the next atomic element, so to speak, is an insight. And that is our opportunity to say, "Right, this is our opinion on the data. This is what we think is the cause of it, or the effect, what it means to us." And then finally, we have recommendation, which is what we're going to do about it. And the great thing about recommendations is they tend to be testable, so they come back round to experiments. So that really simple process of breaking knowledge down I think becomes really powerful into, first of all, understanding your research. But the reason we kind of created it was actually to be able to create a kind of a scalable knowledge repository with these nodes of knowledge that could interconnect. And it's that connection, being able to bring different elements, different knowledge from around an organization and bring them together to come up with ideas, or have lots of different ideas about the same knowledge as well and kind of explore what it means to us. That's where it gets really exciting and really fun.
JH - 00:03:13: The thing that always comes to mind for me is you're in a moderated user interview call, you're speaking to somebody, and you ask them a question, and they just kind of come back with all of this different stuff, right? So I was trying to do this and I got confused by that, and then this and that. And you might have it as, like, a notetaker, handful of things, but there's probably a couple of atomic insights in there. Is that right? Oh, they were anxious about this. Is that, like, how granular you're getting when you say this stuff?
Daniel - 00:03:39: Yeah, I mean, I always try and say there's no right or wrong way to do these things, and it's really dependent on the type of research you're doing, the organization you are or anything like that. But one of the things we have on Glean.ly, which is the tool we built around the process to try and aid the process, is one thing we were really clear about is we kept all items without a fact and insight recommendation really short. So we have a limited 255 characters, which isn't all that much, and every time someone's come to me and said oh, I need more characters, we've looked at it and it's either been the case that they're going into too much detail and it just could be simplified down, or the alternative is, yeah, they're talking a lot about the subject but there's lots of points there and they should be broken out as individual things. And the whole point of this breaking it down is that someone can come in and glance at what they at the different items, the different molecules, or different atoms, I should say, and just be able to understand them almost at a glance. They don't have to spend ages reading something to understand why it's being connected to this or what the relevance is.
Erin - 00:04:48: Maybe we could use an example of what one of these atoms or atomic units is in terms of a fact, an insight and a recommendation?
Daniel - 00:04:57: Yeah, so I think the really useful thing to do is try and think about why we're ever doing any research and why a repository, for example, exists, and that is to make decisions. Right, so sometimes it could be the researchers themselves making those decisions, but quite often we're delivering them to a stakeholder. So it's actually sometimes really useful to think of it conceptually from the first point, which is the last point in the journey, I suppose, which is the recommendation. So the way that it works is, I see a recommendation. I think we should do this. We should build this feature or we should change this thing, or whatever it may be. And then working backwards, we can see the insights, which is like the thinking behind that idea, why we think we should do that, why has this idea come about? And then each one of those insights would also then be connected to the evidence. So you say that this is the case, but why do you think that? Well, we spoke to these people over here. We've got that data from over there, and that's really important. We can bring different data points and different types of evidence, different types of research from anywhere. So that could even be internal. It could be that we've spoken to someone internally. They go, "We really need a new app." Why do you say that? Well, because of this. I think it's because of this that's internal evidence. We're probably not going to build an entire feature just because someone internally said so. Especially like you get the highest-paid person's opinion. Right, okay, they might actually have a point. As humans, we can be very intuitive, can't we? So yeah, okay, that's a data source. As long as we're aware that that has come from internally, it's not a customer speaking, or even if it is a customer speaking, that is one person or several people, it isn't necessarily reflective of the whole all of our customers, all of our users. So actually, now we've got this data over here, it's more quantitative. We can bring all these elements together till we get the confidence to be able to make that decision. And importantly, once we've made that decision, we're going to try this thing. Hopefully, it's a bit smaller than building a whole app, but making a change or building a feature, I think very digitally. So excuse me with that, but I always tend to work within the digital sphere. We've even got people who've used this process and our product for things like investments. So it was one of the first ones that I came across where they were using it outside of the UX field. And it really surprised me that I'd always been thinking it as a UX process and Glean.ly as a UX product. And then there was this team that were making decisions whether they're going to buy a company. So it was a big European company looking to spend like €50 million on buying this small startup, and they were showing how they're bringing all of this evidence together. Right, and from industry-wide data on what's the industry going to, to internal documents and financials and interviews with shareholders and things like that. They're able to bring this all together to make a decision. The weirdest one I've ever seen is an organization that studies murders. So they were actually trying to solve murders, and the problem they had was that they have all of these different types of evidence, from interview to very scientific, like blood splatter evidence, and there isn't really a very good way of bringing that all together. So they found that atomic really helped them because it's completely agnostic on where that information has come from. We can say, right, we know that this happened because of that blood splatter. We know that this person was in the area, or whatever it may be. We can bring all of these elements together in a really useful way. And importantly, we can also do it negatively. So if we've got evidence that actually pushes away for something, we can join that up just as equally as evidence for something, so we can really have a nice, balanced, holistic view.
Erin - 00:08:48: The murder example is interesting for a few reasons, I think. There are organizations that solve murders, I guess private and not private ones, but it's almost like you're talking about trying to really break down and diagram, maybe to use the murder example, like what's going on in the detective's head, right? Like ping, ping, ping, ping, ping. Like making these associations, but making that sort of scalable for lots of non-murderous use cases as well.
Daniel - 00:09:16: I think more than that as well, is that it may not just be a detective. It may be there is a detective in charge of it. But you've got someone who's doing the interviews. You've got someone who specializes in DNA. You've got someone who specializes in taking data from our digital spies in the corner, like Alexa and Google and such like. They can tell us a lot. So they've got all of these different specialists, and they've all got these different pieces of knowledge and things to bring to the table, and actually bringing them all together in a really useful way can be really difficult. Sorry to interrupt that.
JH - 00:09:48: Yeah, no, I feel like I'm hosting Serial right now, I’m excited.
Erin - 00:09:52: We’re going to crack this one.
JH - 00:09:55: So to build on this a little, though, to take it back to maybe a digital thing, I do a usability session, right? You probably generate a ton of facts and insights from even one session, right? Like 50, 100 as you really kind of break these down into these small atomic units. Is the team doing maybe five of these sessions in a week or whatever, just how do you deal with the volume of facts, insights, recommendations that are going to come out of that? And are you putting categories on them, or it feels like, practically speaking, it's probably a hard thing to manage?
Daniel - 00:10:24: I mean, this is where we started. I was actually working for Just Eat, which is justeattakeaway.com now. It's a massive food takeaway, the biggest in the world, I believe. I think they own Skip the Dishes in the US, but I might be wrong. So, yeah, this is an organization that has many brands and many parts to it. And I started leading the accessibility team. I actually founded the accessibility team there. Now, the thing about accessibility is the organization had a lot of knowledge around it, but very rarely people were studying accessibility. It's more they were working on something and there's an accessibility part. They learn something, there's a useful either insight or at least a bit of data that would form an insight. So we were having to go through these piles and piles and piles of research reports, just like tons and tons of them, to find these little kind of nuggets of juicy goodness in there. And it was so frustrating. How can we pull insights out of a research report without using the important context that the report gives? And this is how we kind of approach this. So I need to be really clear, I get the credit of being the creator of Atomic, but actually it was a process that was developed alongside many people, especially within Just Eat. And outside we work with Monzo and printing.com, which you would know in the UK, probably not outside of the UK, and a good few other companies as well. So, yeah, that was where we started with, was we have all of this part of the knowledge and it's really hard to keep hold of it and this way of being able to break things down, kind of give insights and recommendations their own lives outside of that individual research report. That particular study is what allowed them to be able to expand and grow. We see them kind of ebbing and flowing. There's more evidence at the moment that this is true, actually, this is changing now there's more evidence that isn't true anymore. So people aren't leaving the house because of COVID things have changed massively. We've actually recently started working with a UK bank. We work with a few financial institutes around the world, but there's one in particular that we started working with recently. And it was interesting when they said, like, they were saying people think of banking as being quite traditional and slow to move. And at the time I was speaking to them, they said the Prime Minister, we had one of the three we had last year, had just made an announcement that had basically destroyed the economy overnight and loads of people, millions of people, lost their mortgage applications and things like that. So they said, right, literally something happened yesterday which is affecting us today, and we have to react to that. People want to know what's the status of their mortgage? What's going to happen? They're coming up to renewal, they need to deal with that. So even in kind of very what people might think of as quite slow-moving organizations, things are happening all the time, all of these things moving and changing. And the great thing about Atomic is, unlike traditional research reports that tend to be kind of carved in stone and it's very easy to pick an old report and just assume that it's true, with this, because we connect all of these different parts from even not just across the organization, but even outside the organization, we can bring them together and really help us understand what is true at the moment and what's changing, where the patterns lying. And that can be really interesting.
Erin - 00:13:40: Awesome. So I want to go back to you've got the three parts. There's the fact, the insight and the recommendation. Is that right?
Daniel - 00:13:47: So there's the experiment as well. You could see that as more of a context for the facts. So we always say facts belong inside of an experiment, but we find that experiments have what you might call a source. The thing that we did to find this information that has a lot of its own kind of its own information. So we kind of treat that separately.
Erin - 00:14:10: And so, is this like all one unit, the fact, the insight and recommendation? Or can facts map to multiple insights, which map to multiple recommendations? And what's the shape of all these things?
Daniel - 00:14:24: That's where it gets really powerful. You see, just breaking it down helps you understand it. So for instance, one of the most common things I hear from researchers using the process for the first time is deliberately separating the evidence, the kind of understanding, and the decision really helps them. Let me put this a different way, “it's very easy for us to say the user said this or the user did this, so we're going to do that. But actually defining why those two things connect is really powerful. It's really simple, but actually really powerful." And so often people come to me and say, "I thought I understood my research till I started using that process, and then I started thinking about it in a different way." But anyway, I've slightly gone off track there. So yeah, being able to connect it to multiple things, an insight doesn't belong to an experiment. It doesn't belong to how we learned it. It's connected to the fact. So we can connect lots of facts from different parts inside and outside the organization to really see, "Do we know this or not?" An insight. But also we can have one fact. If a customer said this or the customer did this, we may disagree on what that means, and we don't need to argue about it. We can just have two different insights, right? Or we might have an insight we're really excited about, might have two different ideas on how to approach it and what we should do next. Well, that's great. Let's test it, let's see what works and what doesn't work. So it actually really helps that exploration and kind of broadening our ideas of what to do next. And yeah, I find that's something that a lot of people really appreciate about the process.
JH - 00:15:59: You'd mentioned research reports at some point, and I'm curious, do you see that teams who adopt a more atomic approach, they just start using the atomic insights for everything and research reports kind of go by the wayside? Or do they coexist like you atomicize all this stuff and categorize it, but you still write a report to maybe give it the high-level summary for folks?
Daniel - 00:16:18: We did a survey with our customers about it's probably over a year now; it might be even like back year and a half. So it's maybe a little bit out of date. But what we found at that point is customers, especially we're kind of specialized at the moment, at least more with internal teams, kind of works almost better for internal teams. So I think it is different if you've got external stakeholders. I think you're almost definitely going to need to deliver something like almost like a brochure at the end of it. This is what we did for you, but for internal teams, yeah, it's really common now to say, right, I've got this recommendation, share it with a colleague, or oh, you're working on that, I've got an insight that will help you. Here you go. And the great thing there is that really massively reduces reporting. And when we did that survey, we found that it reduced it by between about 70 and 80%. So there's still about 30 and 20% of times where a report was needed. But one of the benefits, especially of being able to share a recommendation or insight as an atomic unit, is people can explore out from there. So for instance, you may send me a recommendation, and I'll look at it and go, great, yeah, I trust JH with this. It looks like he's done his research, he knows what he's talking about. Cool, off you go. Especially if it's a fairly minor change. Right. But if it's a really big decision, I need to have a certain amount of confidence. It doesn't necessarily mean a lot of evidence, but I need to have really good confidence in what we're seeing here. I'll probably read every single little bit, right? I can choose my own adventure, which is really great. So when you've got different stakeholders and decision-makers that have different levels or different approaches to what they need, they can explore, as I say, choose their own adventure. And we find that people sometimes it's a bit like that thing that happens on Wikipedia. There's a little bit of a rabbit hole. It's like, "Oh, we did this experiment. Did we? That's interesting. I want to read more about that. Oh, I didn't realize we did this as well." Because everything's connected, you can end up kind of going exploring and get lost in the data, and it gets really rich. The other thing that I think is quite interesting as well is I talk quite a lot about kind of starting with the experiment, all the facts, and then synthesizing into insights and recommendations. But quite often, that happens the other way around as well. So it might be someone comes along and says, "I think we should do this." Well, we can treat that as a hypothesis and start with a recommendation and go backwards and say, "Do we have any data that supports this?" And either very quickly go, "That's a terrible idea," or go actually, "Yeah, there's some value here," and that means we're investing in things that we already have good evidence for, and we already know there's some value there. Now, of course, you can do that with research reports, but you've got a lot of processes. It's like going to a physical library. Imagine we still had to do that. People do still do that, but it takes a long time. It's a very dedicated, difficult, long process, and sometimes it's easier just to go, "Yeah, well, let's just give it a go," right? With an atomic or an insights-based repository, you can just go in there and go, "What do we know about this subject? Have we got anything to back this up?" and make not necessarily solid decisions but at least have a finger in the air and go, "Yeah, this has got some legs, let's maybe invest a little bit into this, let's do a bit of research." At least we can see where the gaps in knowledge are as well.
Erin - 00:19:35: And practically, how do you go about finding that evidence? So let's just say, like just to be meta because it's fun. I have a hypothesis that User Interviews should build a research repository. I want to go and find evidence to support or not support that this is a good idea, is it based on tags or how is this information sort of like fitting together to then go find that?
Daniel - 00:19:59: Yeah, so one thing I always try to be clear on is, the atomic process kind of starts with assuming there's a certain amount of processing of the data, right? So if you've got a repository that's rich with data, you can probably answer some questions. The better tagged it is, the better taxonomy you have, the easier that stuff is going to be to discover. One of the benefits of atomic process is, because we have these connections between things, it's a little bit more human. I often refer to as coding by stealth, right? Because we work a lot with non-researchers doing research, and I mean, I'm meant to be a professional researcher, and I hate tagging and I hate coding. It's such a boring task. By saying this fact connects to this insight, I'm connecting metadata there, and maybe those individual items are tagged as well. The way that I tend to treat it is, my aim is to give each of those elements context. If I've got an insight, I might say, "This has been tested in France, it's been tested in Germany, it's been tested in Italy," so that's great. If I came in looking for Spain, I can probably look at that insight and go, "Right, I probably want to retest it for Spain, but I can be pretty confident." But you know what? We're working in Japan, and that's such a different culture. I don't think that evidence is strong enough for us to make a decision. We need to definitely retest this, right? But at least they're still all humans at the end of the day. So there's some evidence here that this is probably going to have some value. So, yeah, good tagging, good taxonomy really helps. But the good thing, especially in organizations where there's difficulty in getting consistency or engagement with best practices, that very human way of connecting one thing to another and saying, "This is related to this, which is related to this," kind of solves it. I've seen whole, quite large repositories with almost no tagging, no taxonomy, and they work not as well as they would have done if they fully invested in that. But I often say to people that, especially with an atomic repository, quantity is more important than quality, which is really rare in this world, right? But with research, if it's not in the repository, it basically doesn't exist. People aren't going to know about it, and it will get lost, it'll get forgotten. At least if it's there, we can go and, if it's poorly coded, it may be found. If it's connected to something that's connected to something that I know I'm aware of, I'll probably find it, and then I can go, "Right, why is this not being coded? Let's get this sorted out." But at least I've got the opportunity to discover it, right?
JH - 00:22:34: Yeah, I like that framing. All right, a quick, awkward interruption here. It's fun to talk about user research, but you know what's really fun is doing user research and we want to help you with that.
Erin - 00:22:45: We want to help you so much that we have created a special place, it's called userinterviews.com/awkward, for you to get your first three participants free.
JH - 00:22:56: We all know we should be talking to users more, so we've went ahead and removed as many barriers as possible. It's going to be easy, it's going to be quick, you're going to love it. So get over there and check it out.
Erin - 00:23:05: And then when you're done with that, go on over to your favorite podcasting app and leave us a review, please.
JH - 00:23:13: You've been thinking about this and working on this stuff for a while, a number of years now. How has it changed or not from when you first started getting into this? Is it pretty similar and just evolved a little bit, or are there parts that are actually quite a bit different and have become much more sophisticated, or changed your thinking on it?
Daniel - 00:23:29: That's a really good question. I think it's mostly stayed similar, and obviously, there is a certain amount that is kind of difficult to change because there are lots of organizations using it, and it needs to almost maintain the standard. So one of the things that, especially as UX people being involved, we love to talk about terminology and get really fussy around terminology, myself included. And yeah, I think when I started this, maybe through a lack of confidence in it or wanting to make it sound slightly more official than I maybe needed to, I used kind of scientific terminology. We had "experiment" rather than "study" or "source" or something like that. That's the most commonly changed terminology I see on Glean.ly. Facts I quite support. I think "insights" can be confusing because a lot of people think of insights as what we might call a fact. So that's always an interesting conversation. A recommendation we actually used to call "conclusion." So when we first started this process, it was actually called "conclusion." If you see my first medium article, there are still some references to it in there, but that was definitely wrong because "conclusion" sounds like it's final, right where it's just the beginning of the journey. So terminology has definitely been one that comes up again and again. I see quite often that people sometimes add other layers to it, so either a layer maybe in the middle or layer at the end or something. I'm trying my best to think of an example completely coming up cold. I'm so sorry, but I've always been interested in that, and there have been a couple of times I've been tempted to officially kind of bring it in and not that I'm like the gatekeeper of this, but being able to say, "Yeah, actually, we really recommend that you add this extra section on that could be quite interesting." Every time I've looked at it, I've always felt, "Yeah, it's right for that organization. I can understand why they've chosen to do it, but I think it makes it slightly more complicated than it needs to for most people, and simplicity is part of the power, right? It needs to be accessible by everybody, especially non-researchers doing research. That's such an important place, but even more than that, are the stakeholders. And this is the thing we have at the front of mind whenever we're designing around this product, around this process, is who are we doing it for? It's the people making decisions, and as I said earlier, sometimes they're researchers, but quite often they're not, and they need to be able to come in without any idea of what it is. They don't need like an hour's training or going to a boot camp or something like that. They need to be able to come in and understand that data straight away and be able to make good quality decisions straight away. So that's always a focus on what we're doing it for.
Erin - 00:26:08: You've talked a bit about non-researchers doing research throughout so far, and that's definitely something we're thinking about a lot. Sure, you hear about Democratization talking about that for a while, but the point is non-researchers are doing more research from what we can tell than ever before. And so I'm curious how that fits with Atomic research to you. Are you finding that this just clicks with non-researchers, or that it needs to be adapted? What about there's this sort of, if you think about the library, the repository kind of putting the insight in and coding it up and then there's the pulling it out of the library and using it - who's finding this to be something that works for their workflow? More or less researchers, non-researchers? And how is everybody playing together around this system?
Daniel - 00:26:55: So we tend to find that the people in the organization that are bringing a product like Glean.ly in or aware of the process of Atomic tend to be researchers, like professional researchers. But I would say maybe three out of four clients that I speak to, one of the first things they're saying in their objectives, not just in their objectives, but probably one of the first primary objectives is desiloing knowledge, right? And so by that very definition, that means it's going to be across different disciplines. So I'm seeing a lot of companies where there are research teams managing non-researchers. So more and more like UX researchers, their entire job is not to do research but to manage the process of it reops, I suppose, research ops that's getting more and more common. But when we're desiloing, I made this mistake when I started with this process thinking this was a UX process and knowledge management was a uniquely UX thing and of course it is and that was so naive of me. Marketing has loads of knowledge, sales have loads of knowledge, especially sales, right? They tend to be on the front lines and customer service even more so. But it's interesting in our organization, we even have the developers using the repository because if I'm designing a product, one of the limitations is what can the software do, what can the technology do or some of the opportunities I might not even be aware of. So I'm really keen when I do research to bring a cross-functional team in. So if I've got a database analyst or someone like that on processing the research, likelihood is they're going to be aware of a possibility that wouldn't even come into my world because I'm so much so far away from database management. I come from a UI background. So yeah, I find that Atomic is a really good framework for non-researchers because it gives that kind of lightweight, lightweight let me try that again. It gives that lightweight structure to the research they're doing and the knowledge they're trying to form and keeps a certain consistency as well. So we talked about tagging earlier. One of the tools that we have in Glean.ly we create like what we call custom filters, but they're categorized tags, I think any research would know them as. And the benefit of that is it gives a consistency of coding. You've got this consistency of structure around, we've got this evidence, this is where it came from. Separately, this is our opinion on that. So we're not getting those two muddled up and bringing in bias and then separately what we're going to do about it, which allows us to kind of bring new evidence to bear and change our mind and encourages retesting as well. These things are being drummed into us as researchers, but may not come so naturally to other people that are really excited about their new idea and want to just build it. They don't care about necessarily the evidence behind it. So yeah, generally we're seeing a lot of I would say there's more non-researchers that have really been helpless than researchers, but where it's really helping the researchers is being able to scale their work.
JH- 00:30:06: That's really useful.
Erin - 00:30:07: Yeah, you talked about quantity over quality, which I love because no one ever says that, but it's a nice.
Daniel - 00:30:14: I can’t think of any other part of life where quantity rather than quality is.
Erin - 00:30:21: No, but I like that a lot and I think it's approachable for non-researchers as well. Is there like a guideline you have in terms of if you're doing a 30-minute to 60-minute user interview, some kind of moderated research? Like what's a fact or not a fact? How big should the fact be? I think when we think about volume, obviously, and you talked about what, 255 character limit, right? They should be small, I suppose, in a relative scale. But if you already have this fact, for example, in your repository well-represented, do you need to sort of add it again, or are you looking in a half-hour conversation to get five facts, ten facts, or a hundred facts, or is that the wrong way to think about it? How do you encourage particularly non-researchers?
Daniel - 00:31:05: I think it could be the wrong way to think about it. Yeah, it should be “what's useful?” An atom is a useful piece of knowledge that guides us, right? And that may not be straight away. One of the first organizations outside of Just Eat that I worked with of this process. One of the things we did is we went through some of their legacy research, and we noticed the same pattern came up several times. And they had made a note of it, but they didn't really think it important because it wasn't what they were researching. It was a different department that was responsible for that thing. But because we were creating this atomic process, we're bringing all of these things together, this was building up more and more evidence. It was coming up in about one in every three or four experiments. So we actually took that to that department and said, oh, by the way, just to let you know, we've been finding this information. It might be useful for you. And they were like, oh my God, this is really serious? Really? Okay. They said, yeah, we're coming up to our biggest trading period of the year, like where 70% of our revenue comes from, and we won't be able to properly trade unless we sort this out. Now, luckily, it was actually quite a simple thing to sort. They had about two months to go, and it only took them about two weeks to get it sorted, but they weren't aware of it. The company was aware of it, they just didn't know they were. So actually being able to bring all these things together was really useful. And so in that regard, there seemed to be something to record there. And I can't really define it much more than that because sometimes it may be that you have an interview with someone and yeah, things are coming out, it's just pure gold, and sometimes it's just like checking off some boxes. Yeah, they can do this. Yes, they can do that. So in that regard, quite often people ask, okay, so I've had three people do the same thing. Do I create three different facts? Or can I say three out of five people did this? And once again, there is no right or wrong way, and it really depends on what's right for you guys and what's right for the people doing it. The useful thing, if you've got it three times, it shows that kind of quantity. But just because a lot is happening to a lot of people doesn't mean something that's only happening to one person isn't important, because that could be really serious. That could be a blocker, right? So that shouldn't be the be all and end all.
Erin - 00:33:14: So you're saying basically, don't force it. And if it's useful, which obviously this is something that trained researchers get better and better at over time, right, knowing what's useful and what's not. But if it's useful, it's a fact, if it's not, it's not, you got it.
Daniel - 00:33:29: Also on the point of what makes a fact and what makes an insight, that can be really interesting, and as I say, by the clues in the name, is that it has to be factual. But even then, one of my favorite occurrences is when I was actually on a call with a client and they had put some results from a survey through the process, and they had an insight, which was something along the lines of 70% of our customers prefer green clothing to other colors. And I said, "I don't think that's." So the conversation started around, is that a fact? Or an insight, but it became so much more. It was fantastic. So I said, because this was what the survey told us, what the respondents to the survey told us. It's a fact. It's not our opinion. Right. What's interesting there is why is that, first of all, is that an unusual number? Second of all, is that kind of borne out with actually other data? So luckily someone on that call had access to their sales data and was able very quickly to go, "Yeah, we're selling a lot more green clothes than any other color." Right. Okay. So we've got two points of evidence here. We have no idea why this is. We're going to have to start doing some interviews. Let's find out. But they could still start thinking about, well, maybe it's something to do with our branding. Is it to do with this? Okay. But also when we think about the insights, it's not just the cause of it, but the effect, what does this mean to us? Should we lean into this and become the green clothing company? Why are we kind of leaving out all these customers that like, prefer red clothing? Is there an opportunity here? Is this a problem? And someone said, "Right, wrong recommendations is we should probably change the hero image on the homepage." So on that call, someone was busy creating an AB test while we were discussing this. I didn't see them until about one or two months later, and I can't remember the number, but it was quite a significant rise in conversion from that thing. And they said they paid for the software like ten times over just for that one call because they discovered this thing, they could see it there. The data was there. The company had knew this stuff. It just was not really, it hadn't kind of struck a chord. It hadn't been turned into something it hadn't actually been turned into something usable and tangible before. And that was so beautiful for me to see, and quite rarely do I get to see that in action. Sometimes I hear the stories, but it was lovely to actually be a part of that and be involved with that as it happened. It was really exciting.
JH - 00:35:52: Yeah, it's nice in the feedback loops like that.
Erin - 00:35:54: Well, why, though? Why do they like the green clothing? I want to know.
Daniel - 00:35:59: Well, no, that's a really good question, because the strongest theory at the time was something to do with their branding, but their branding wasn't green.
Erin – 00:35:06: So interesting. I think green is a bright color. We all want green
JH - 00:36:10: I was waiting for you to say it was like St. Patrick's Day or something. It was like a seasonal.
Erin - 00:36:14: Or colorblind with the red and the green. I don't know. Something happening there.
Daniel - 00:36:18: So one of the things I don't know whether they did this, one of the things obviously you'd want to do there is maybe have a look at is that just a standard national representation of most people, prefer green clothing? I don't know, I'd have to check in with them because I quite often use that story because I really love it. But that's a really good question.
JH - 00:36:38: Yeah, there's been a lot of nuance we've hit on here in terms of how you do this. Do you lump the three things together, put them in three times, and some of the other things you've touched on? If a team is curious about this stuff, is atomic research something you can kind of, like, dip your toe in the water and try it here and there, or you got to kind of go all in and be like, "We're doing this. It's a new thing. Let's commit and figure it out." How do you get started?
Daniel - 00:37:00: I think it's something that you can definitely just do on a project basis just to test it out. As is this a good way to synthesize and to understand our knowledge? That's probably the best place to start, and that's certainly where we started, just with Whiteboards. I think if you're looking at it and you need to create a repository, a repository is one of those things. It's all in or all out kind of thing. So yeah, easiest way to get started is literally on Miro or something like that, literally creating facts and drawing lines to create insights. That's how we started. We then went to Airtable to try and create a more formalized thing, but very quickly we found that Airtable is a fantastic flexible product, but it couldn't scale quite how we wanted and there were particularly a couple of things we wanted to be able to do that we couldn't. So one of the things we can do on Glean.ly, for instance, is changing how we look at something. So we can see the world from the point of view of a fact or see the world from the point of view of a recommendation, which probably sounds quite weird as I put it like that, but it does make sense, I promise you. So that's one of the reasons we built software. We only built the software because we had certain things we wanted to achieve. You can absolutely do the process without the software, especially for small organizations, right? If you're a lone researcher, we have plenty of lone researchers or small organizations using the product, but they don't have to. You can get away with using anything when it comes to actually making this a formal part of how an organization works. There is a level of commitment there and that's a real difficult thing to get around. It's difficult for legacy, but I think the biggest difficulty is engagement especially. We were talking about non-researchers earlier doing research. They're the hardest people to engage in something like this. So much so, we did some research not too long ago, and it was really interesting what we found because there were a couple of things we were expecting. So first of all, they're not researchers. This isn't a part of their, this is just a part of their kind of actual role. So they're trying to get the answers for the thing they want to do, so they've got their answers that move on. Right. They don't really necessarily see the benefit in or fully understand how valuable a repository can be to them. Secondly, it's a new piece of software. They have to learn it. That's quite off-putting. But the thing that maybe should have been obvious but was a surprise to me was actually the biggest one, which was, "I'm not a researcher. I don't necessarily feel confident in my research. UX people always telling me how difficult it is and why you should earn so much money." Right. I don't feel confident. I've never had training in this. I don't want to put my research on display in front of my whole company, all of my colleagues, which was, oh, God. Yeah. It was one of those kind of sunrise moments where I was just like, of course, that makes absolute sense. How do we deal with that? Actually, we found just recognizing it, just literally calling it out and saying, "Yeah, you're probably going to feel a little bit exposed here, maybe a bit vulnerable here. That's okay. That's normal. Actually, that is enough.” Surprisingly, we'll keep on looking at that and seeing if there's even better ways and more ways. But I think even just recognizing that and rather getting frustrated with people, kind of empathizing with them, which we're good at as researchers, right? For the most part, understanding where they might feel uncomfortable, but yeah, certainly worth testing it out, giving it a go, seeing what works, what doesn't work, changing things. I think we talk about a process, and that can feel like you have to do it a certain way. There's a right way or a wrong way, and there isn't. There just isn't. There are definitely best practices and things that I've seen worked. I've got a cheat sheet, which you can probably just Google Atomic UX Research Cheat Sheet. It will probably come up, which can be useful. But there is no right or wrong way. So every organization is different, every individual is different. So, yeah, do it your way.
Erin - 00:40:53: Great. And we'll link that in the show notes, too.
Daniel - 00:40:55: Oh, please do. Yeah.
Erin - 00:40:57: Awesome. Well, thank you so much. This has been so interesting. I want to create some Atomic nuggets myself right now.
Daniel - 00:41:03: Yeah, start frying up some nuggets.
JH - 00:41:08: Thanks for hanging out.
Erin - 00:41:10: Thanks for listening to Awkward Silences, brought to you by User Interviews.
JH - 00:41:15: Theme music by Fragile Gang.
Episode Video
Creators and Guests
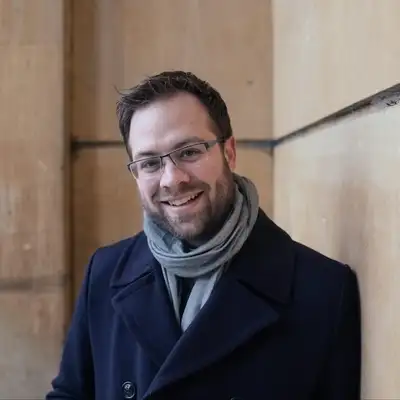