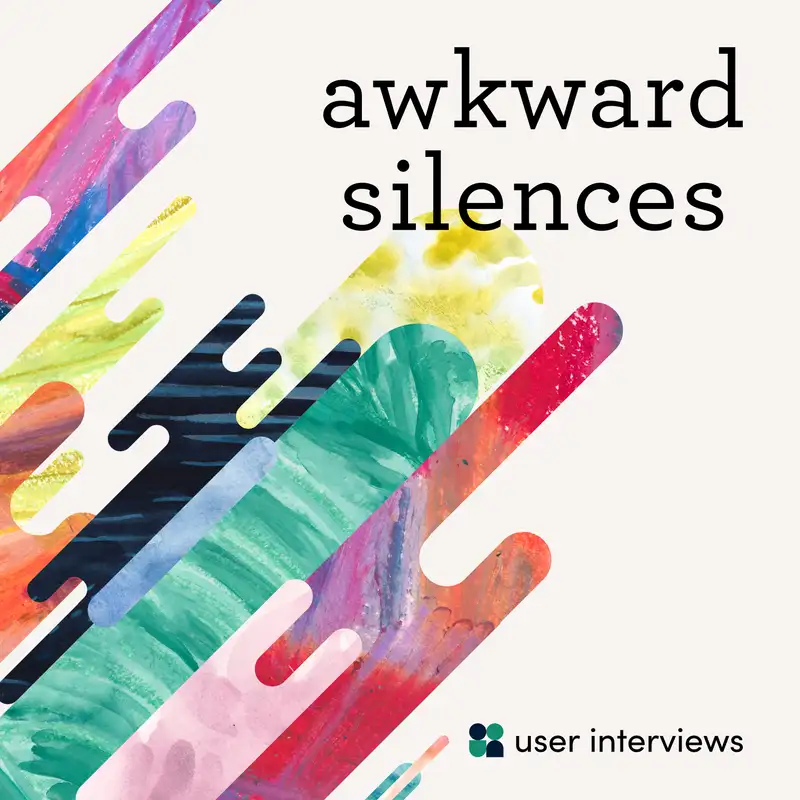
#126 - AI Anxiety: The Dangers (and Opportunities) of AI in Research with Mike Adams of Grain
Erin - 00:00:43: Hello everybody and welcome back to Awkward Silences. Today we're here with Mike Adams, who is the CEO and Co-founder of Grain. Really excited to have you here today to talk about AI and research, a very hot topic.
Mike - 00:00:56: Awesome. I'm excited to be here.
Erin - 00:00:43: We got JH here too.
JH - 00:00:58: Yeah, excited. We love Grain. We use it a lot on our team, so that's always fun. And then we've always said, Erin, I think that we try to stay away from topical things. But I think this topic is here to stay, so this will, I think, become an Evergreen episode even though it's having a bit of a moment.
00:01:13 - Erin: Yeah, for sure. Evergreen sort of can start out with a topical, and this has been an interesting one where I can remember my last, my first sort of B2B job. There were these waves of the hot tech topics, and it was like AR, AI, ML, all these acronyms, right? And it was kind of like the future promise that wasn't being delivered. And it feels like recently with ChatGPT, it was like that was the moment, fever pitch AI is here and kind of past the hype stage, and who knows what will happen, but seeing real use cases and enthusiasm. Mike, are you seeing that too, and how do you think about it in the context of research?
Mike - 00:01:50: Yeah, absolutely, and I think this conversation will be probably interesting in that none of us are professing to be AI experts, right? I think we're all kind of experiencing this moment in time, and in particular, at Grain, we've been keeping an eye on these technologies because they provide very obvious solutions in theory to problems that our user base experiences every single day around trying to categorize information or synthesize it or summarize it. And all of a sudden it went from this kind of, as you mentioned, Erin, like hypothetical fever dream to like, “Oh wow, it can do that.” And I feel like your average person is probably very limited in their exposure to what these large language models can seemingly suddenly do, even though they've actually been able to do this for a little while. I think it's just ChatGPT that moved it into the consumer mind, and that application of the large language model of GPT-3 is really just like one kind of nichey application of it, and there's so much more power that is really there at our fingertips, and it's just a matter now of kind of like applying it to aid and augment the work that we do every day.
JH - 00:03:08: Totally. Have you gotten a sense from your user base within your team? Is this something that user researchers are kind of more excited about or maybe a little bit more fearful of? Is it the “it's coming for our jobs” thing, or are people like, “Oh, this is gonna help us so much.” Any read on that?
Mike - 00:03:21: Yeah. It's a good question, and I think it's worth clarifying that at Grain, we have a lot of user researchers that love our product and use our product, but we're not like a tool for user researchers. We're definitely more like your kind of founder that's trying to do qualitative research and conversational research, or your kind of smaller UXR teams. Or there’s some larger UXR teams that have embraced Grain over more purpose-built tools because of the simplicity of being able to pull out, select some text on a transcript, turn it into a clip, and embed it everywhere to distribute that voice to the customer. So I think that's just an important caveat first that I think the point of view I have is probably more like people who do research as Kate talks about from Atlassian, more so than like the proper research as a function. But I would say that the kind of more informal side of research, there's been a lot of enthusiasm I've been seeing around just the idea of automating the busy work that I would normally have to do myself or pay an intern or pay some MTurk type of situation to go through and perform a transform on conversation that was had and make it actually into an output that's useful. I mean, we're really not that far removed from when transcription used to be this exact same process, and I think transcription is how ubiquitous and how useful and how helpful it is, is a representation of kind of 10 years ahead of where these large language models are in terms of the type of value that you can get from something you used to have to just manually do. Super painful, it was really expensive to do, and so it's that sort of kind of monotonous, busy work that in the line of work you end up having to do because there isn't really anybody else to do it and there's not an automated way to do it. That I think is the most exciting part of the first wave of these large language models, being able to kind of automate away some of that.
Erin - 00:05:18: What applications are you most excited about as you think about the most viral posts I've ever put on LinkedIn was sort of poking fun at all the ChatGPT speculation, right? And like everyone's an expert on this, but I think if we bring it down to earth and really talk about how, in the near term, we're actually gonna see this technology applied not just with ChatGPT, but with actual applied AI in the context of Grain and research, what are you most excited about? We talked about saving time, getting rid of tests that could be automated.
Mike - 00:05:49: Yeah, totally. So I guess a quick kind of preamble that is, like, my favorite thing is to watch Bing go crazy right now, like all upset and like, "You're a bad user, I'm a good Bing." And so I think we're all on the same page around how limited and unrealistic it is to think that these models are going to come straight out the gate and act in a way that we would expect them to. But that doesn't mean that we're not seeing immediate applications that are actually game-changing around the work we do on a daily basis. So at Grain, we just opened up our beta for Grain AI, where we have, I would say, three main features that we're doing, using large language models, in particular using GPT-3 on the back end. But one is just like summarizing the meeting down into its core moments, and that idea has been there in similar products to Grain for decades, and we've never really bit the bait of the hype train because it doesn't really work. And now, all of a sudden, it does, and it's pretty incredible, and it's massively time-saving. To be able to take a 30-minute meeting, see the six to seven bullet points that are most representative of the main ideas, kind of scan through it, and in the context of our product in the beta, you can kind of click on the summary and then go to the actual underlying text and see what the substances that the summary is kind of paraphrasing. And I've found so far that it kind of takes that motion. I'm not yet able to really just take the summary at surface level and be like, "Yeah, this is now the replacement to sitting in the meeting for 30 minutes or re-watching it at two times speed." But it becomes like markers to saving a huge amount of time around trying to get the essence or just go to the moments that I particularly find the most interesting or valuable. So that's like one application. The other one is like question and answer detection. And so that's a pretty common application at the large language models, not just OpenAI models, but Google has this and a bunch of other API-level services have built like question-answer detection. But what I find particularly really interesting is not just the question and answer detection, but the question detection and the answer summarization. And that becomes huge time savings because, again, we are really limited in the day how we spend our day. And nobody wants to sit there and re-watch a thing they were a part of or maybe even weren't a part of. They're trying to catch up. And what you really want is to be able to just kind of dive in and get as close to the true essence of what occurred or what was said as possible without having to spend that entire time. So the question and answer detection becomes a really, I would say, question and answer summarization becomes a huge time savings to doing the sort of synthesis analysis work that you need to do, as opposed to and it just is removing some of the monotonous labor of having to kind of just read the entire thing from in its raw format and just kind of start your consumption in a more summarized place to dive into the right spot.
Erin - 00:08:51: Yeah, and we know from our research, researchers don't like doing that work, which is intuitive, but it's the most painful part of the process. And to your point, you could imagine the AI robots can do this well, whereas, No participant wants to sit in a user interview with a robot, right? That's a good one for humans to probably stick with for a while.
Mike - 00:09:11: Yeah, I think the interviewer's job is going to be safe from robots.
Erin - 00:09:16: Yeah, I think so. Yeah.
JH - 00:09:19: There's an example that I know was popularized in some books, and I forget where else I've seen it, but when some of this stuff years ago was starting to come along, and people were trying to make computers really good at learning chess, the thing that came out very early on was it's really hard to get a human to become an expert level chess player. Takes a lot of practice and skill, also very hard. Back then when things were a little bit more limited to get a computer to be at that sort of grand master level as well. But what they found along that journey was that if you pair like a novice human player with kind of a somewhat sophisticated computer model, you really quickly actually could have through that hybrid a really powerful competitive player. And I feel like this is kind of in a similar spot where I think if you try to outsource your synthesis and insight analysis fully to the models, there's going to be some gaps or some things that maybe you can't fully trust just yet. But if you're not leveraging them at all, like it's, you're not taking advantage of the time savings that could be really helpful and kind of getting that pairing where it's pointing you and doing some of the 80-20 work but still need some human review and like cleanup on top of it. I don't know if that kind of tracks with what you were saying, Mike.
Mike - 00:10:15: Yeah, a hundred percent. So one area where I think folks that are just exposed at like the ChatGPT level and haven't done any like prompt engineering in, so for example, you can go to the OpenAI playground and you can say really simple. I would actually recommend people to do this, grab some transcription or some other texts that you want to find some information inside of and say, "Here is some text. Paste your text in the playground. Here is the prompt," and then write your prompt below it and say, "Find all of the concerns," or I even had one the other day, which was “Estimate the user's NPS on this transcript and explain why." And so the output of that prompt was actually pretty phenomenal. And it was like, "The estimated eNPS is 8 out of 10, and here are the reasons why." And then I went back to the transcript to try to kind of trace the conclusion of like an 8 out of 10 versus a prompt detractor, like a 2 out of 10. And it actually tracks really well, but it's like identify the feature requests of the users or the bugs that are mentioned and those types of moments are actually able to be identified automatically in a way that can save the actual research or the person doing the research a huge amount of time of just that like busy work of having to kind of go through and find it. And so there's kind of those two layers on an individual, like an interview where it's either like, "Save me time by pulling out the things I'm interested in so I don't have to stand through it," or "Perform a cognitive kind of evaluation on what could be the case, like that eNPS, like what's your estimation or sentiment?" Both of those are pretty, I would say, powerful and realistic current applications technology. But right now it's kind of limited off of your imagination of what prompts you can come up with. And then the output being right there in like a playground because there's just not that many tools yet that have been built that make doing that sort of work on a body of specific texts that you're trying to understand, like the transcript from an interview, for example. There's just not a lot of tools yet that are putting that power into an end user's hands.
JH - 00:12:36: All right, a quick awkward interruption here. It's fun to talk about user research, but you know what's really fun is doing user research, and we want to help you with that.
Erin - 00:12:44: We want to help you so much that we have created a special place. It's called userinterviews.com/awkward for you to get your first three participants free.
JH - 00:12:54: We all know we should be talking to users more. So we've went ahead and removed as many barriers as possible. It's gonna be easy. It's gonna be quick. You're gonna love it. So get over there and check it out.
Erin - 00:13:04: And then when you're done with that, go on over to your favorite podcasting app and leave us a review, please.
JH - 00:13:13: Yeah, I like the call-out on the prompt engineering, because I do think that's such an important part of it that is not that familiarized for people yet, or not that common. It's like you give somebody a spreadsheet, an Excel file, or whatever; you can use it in all these powerful ways, but you have to kind of know how to lay out the data or what functions or things are in there. And it's not the exact same, but you do kind of need to learn how to use the tool to maximize the benefit from it. And that's probably something, to your point, that people should start to find kind of cheap and clever ways to play with, so they can start to internalize how you can take advantage of this and where you can maybe deploy it to save yourself some time going forward.
Mike - 00:13:44: I think prompt engineering is going to be like one of the core skill sets that everybody who does like cognition-related work is going to have to get pretty good at because, you know, it's garbage in, garbage out, and the garbage in is the quality of the extra adding in and the quality of your prompts.
Erin - 00:14:00: And it is just like learning how we all learned how to Google search, right? You can kind of do the easy way, which most people do. I forget, what's it like, 10 or 20% of Google searches are unique and never been performed before, but you can do all the advanced operators and get sophisticated with it. Same thing, it's a new sort of model of interacting with outputs and the dialogue back and forth.
JH - 00:14:21: Put Reddit at the end of your query. It's the best running shoes, Reddit, and needs to get to those. Yeah.
Erin - 00:14:26: Pro tip. Yeah. If you want to really go down a rabbit hole
Mike: 00:14:29: I'll pull reddit.com; we'll dramatically improve the likelihood of getting a good answer.
Erin - 00:14:33: But to your point, you were talking about using these sort of API protocols and pulling in AI that others had done. I'm curious just to go below the hood a little bit, and when you think about building versus buying AI with your own product development, how do you think about that? As the AI has come far enough, how do you make those decisions?
Mike - 00:14:52: Yeah, so OpenAI, and I think there's Cohere, and obviously Google and others that are the actual API providers of these large language models, they're in a pretty good spot. I liken it to, I think about it like kind of AWS almost, where yeah, you could manage all your own servers and you could do all of that work on your own. There's like ways to do it. But it's just so much easier, and the economies of scale make it such that like you're never gonna want to manage your own server farm. Just use Amazon web services or some other cloud server. And that's kind of where we're at on these large language models. Is that what you're. I think there is kind of basically a skip function that's occurred around doing custom bespoke machine learning in the application or context of a product or a UI that all of a sudden now is just leapfrogged by proper prompt engineering and kind of systems design. Such that you can kind of feed specific information to one of these large language models with a really good prompt and then get something out that is just dramatically better in terms of a machine learning prediction result than you could if you were trying to train a bespoke model on your own. And so there's also, that's specifically in the context of generative AI where you're trying to get the large language model to produce either text or results. There's a ton of other applications around classification and embedding around identifying patterns, which is a kind of totally different beast from what I'm just mentioning. But it's still in the same boat of it being valuable to kind of leverage some of these just large language models and OpenAI protocols with that are actually going to be significantly better than trying to build it, kind of bespoke in-house. And so I kind of think about it similar to that kind of move to the cloud that happened a decade ago.
Erin - 00:16:48: Yeah, I'm just playing out the ethics and the sort of multiplier effects here because if you have these two or three main APIs that become embedded in every technology we're using, we'd better hope they have good training models, right? We've talked a lot about the ethics of research that goes into it. Who do you talk to to develop what become the predominant patterns and things like this? So yeah, I'm curious to see how that all plays out, hopefully for the better and better all the time.
Mike - 00:17:15: And the nature of the problem is that these are all based on neural nets where the output is feeding back in and becomes input to the next iteration of the output, and so it's kind of constantly going to be evolving, and changing. So there's both the component of the arbitrariness of the models, but then also there's this unpredicted nature of the fact that as we use these models, it feeds back in and it kind of changes the nature of them.
JH - 00:17:42: Yeah, it gets a little circular. We were talking, talking about this - something you've kind of ramped up on and developed a better understanding as kind of hype has been proven out. Any ideas for how people who are maybe seeing these headlines but haven't played with the tools firsthand or just don't feel familiar? Like what are good ways to get started and kind of cut your teeth on what these things can do and how to inform yourself a little bit more?
Mike - 00:18:01: Yeah, really good question. So my background's in education, and I love visual learning. That's how I like to learn; it's kind of my style. And so you mentioned Mid Journey, the kind of image-based AI applications. There's a bunch of them, but the one I've been playing around with is Mid Journey. I actually think just like joining the Mid Journey community and getting in those discords and seeing what people are doing and following like the Mid Journey subreddit and seeing those outputs, that for me has been super interesting. Because usually, there's a tie between the prompt that was written and the output of that prompt. And for me, it's just a matter of kind of training my brain around like as a prompt engineer, as a, not to get too fancy, but like you are the writer of the prompt to get the output and the best outputs and these incredible images that are being generated using AI. Are really a function of the quality of both the input. Sometimes you'll feed it like a base image to do things on top of, and so you can do like a transform on top of like a photo you took or a drawing or a sketch that you have and then turn it into something truly incredible. If you kind of know the sophisticated prompts and what is available in terms of what the model can understand and then apply to get the output that you'd like. So I would recommend spending some time as weird as it would seem to like screw around with prompt engineering for imagery. It might not have a direct application, but it is one of the best, I think, ways of being able to really understand how we will be working with these models. And even though right now there's just kind of a lot of abstraction in terms of knowing even, what are the parameters you could put into the prompts that you're writing? To get that output. One quick example of this that kind of I slept on is I used to teach at a coding boot camp when it was brand new and I would, one of our student's projects was like AI images that they were outputting. And I remember thinking it was so silly, but they were so excited about it. They were like this random smudgy compilation of pixels was generated by AI, and they were so excited about it and they wanted to kind of create a consumer application so everybody could make their AI images. And obviously, they were just eight to, you know, seven to eight years too early. But that was the kind of same basis that now has a pretty substantial following. And so that's like suggestion number one is to start to just kind of like learn how prompt engineering works. And then I think suggestion number two is what I mentioned a little bit earlier of like, just get into OpenAI's playground. It's just playground.openai.com, and like I mentioned, you can, there's a bunch of different examples out there, but it's just like, here is some text. Here is put the checks in there. Here is a prompt, and just play around with what questions you might have of the thing that you gave it that it can give you in response. And obviously, these are all examples of kind of the generative AI application that is just one of the elements of everything that's going on right now, but I think it's kind of the tip of the iceberg and the most exciting way to explore right now.
JH - 00:21:03: Totally, the community piece. I think the prompt engineering is really important because I do think even if you're very creative and you're playing with these things kind of in isolation, after like half an hour, almost even, you're gonna like start to hit the limits on the ways that you think to poke it and throw prompts at the model. And then if you see other people, it just continues to unlock of like, oh, I didn't realize it could put that kind of modifier on there or do this. I think you just need to kind of build that web. And so I think being in a community is a really good idea. The thing I think has been really interesting, and maybe it'd be a good one for researchers, is I think it's really obvious to go to the synthesis idea of like, here's a transcript, boil this down. And certainly would encourage people to play with that. But I know, I think Erin, you were playing with this on some other aspects of research, like write me a screener survey for this type of profile, and maybe you'll come up with some questions you wouldn't have thought of that you could weave into your own or ask it to describe like the persona that you're talking to and where can I find those people? Like where can I find machine learning, like surface communities you don't know about to help with your recruiting or whatever. So I think there are lots of adjacent things within research that people should also kind of poke on to see what's possible. And again, you probably won't use it a hundred percent the way it is outputted, but it might unlock some stuff for you as well.
Mike - 00:22:05: I think that's another really good suggestion is this almost kind of, remember the “Ask Jeeves" It's like ChatGPT is actually the manifestation of this problem - app.com or "Ask Jeeves" where you're like, "Hey, Jeeves, could you go tell me where all of my target audience lives and what communities I should join?" And that's what, like Jeeves, even just inside of ChatGPT, it does have the limit that I believe the dataset is only up until like 2021. So it's not going to be the most up-to-speed, but I believe very soon, Bing is actually like has a much more recent dataset. But I think that's another great application. Is like just asking kind of questions related to your audience that you're trying to understand or serve. I've also had it generate a user interview guide for me for our product. And then I've also, what I like as well is it has kind of like the aggregate understanding of the internet basically in terms of even if it's a very highly specialized and technical result. It will kind of distill down the essence of common belief of an expert community, which I find to be really useful as I'm like, "What are the most common evaluative user testing questions that I should, or user interview questions I should ask?" And I've actually found that output to be pretty great, and then it can inform the interview guide I go in with.
Erin - 00:23:23: We should have it: find us podcast guests and write all the conversation. Guys, we're set. We're going to save so much time. But yeah, it occurs to me there's sort of like, how do you, now, how will you soon use AI as a researcher to make your job better, easier, more efficient, so you can focus on the stuff that humans are really good at? And how do those two come together? To your point, JH, if AI can help, how do you kind of insert yourself at the right moments? But then there's also like we were talking about, this prompt engineering for researchers to have these new ways of interacting with technology top of mind because that's going impact how you think about building great products and how you understand the changing relationship, like the sort of macro relationship of consumers to technology. Because it feels like this is going to result in sort of slowly and then all at once, kinds of changes potentially.
Mike - 00:24:17: I can't remember exactly whose tweet it was or the exact phrasing, but the gist of it was like these new models are less like one Albert Einstein and more like a hundred high schoolers. They're capable, but they're not going to make these incredible connections that you can even make. I think for a while, for a long, long while, I think that's going to be our job to do.
Erin - 00:24:41: Yeah, I like that analogy.
JH - 00:24:43: Yeah, I think it's going to be really interesting too to see how and where these things manifest in different product experiences. Like you were describing, Mike, how you all are playing with it in Grain and introducing some beta capabilities. But, kind of like what you were saying even earlier with the ChatGPT interface, it's like the underlying model had been available for a while, but putting the chat interface on top of it kind of made it click for people, and it's like, "oh, this is accessible. I can use this." And I think that'll be true for a lot of research-related products. Not just a matter of having the AI built-in and having access to language models, but it really does become about the experience of how and where you present it to people, how they're able to interact with it. And even though maybe people, everyone's using the same API behind the scenes, you can still deploy it in very different ways, right? Lots of apps are built on top of AWS, but they're all pretty different. And so I'm just very curious to see how that'll play out and just, yeah. I don't know if you've seen anything interesting within other research products or just in products in general that seems to be catching on.
Mike - 00:25:33: Yeah, to be honest, I feel like there's not a lot yet. I think you're totally right that these models have been around for a really long time. There's definitely capability improvement shifts that have happened with kind of GPT-3 to GPT-3.5. I'm super excited about GPT-4. It's unclear what sort of step changes, but it should be an improvement in terms of that accuracy. But yeah, there's definitely been the ability to do these types of things for quite a bit longer than the hype around the stuff, and it is the ChatGPT interface that I think has kind of made it accessible for everybody. And I think this year and in the next couple of years, it's going to be a really exciting time as really the innovators that are trying to solve specific user problems. Like in our case, it's this kind of meta-application of building products for people who are building products. But that is going to be, I think, a really exciting thing to watch as it happens over the next, I would say, six to nine to eighteen months. But there isn't a lot out there, and I'm watching it pretty closely, and I think there is definitely a lot of roadmap shifting that has occurred on these types of products over the last, I would say, three to four months. It definitely has shifted our roadmap, but it's, I think, going to be an interesting kind of wave of like, "Wow, that's incredible." And then that thing went from being incredible to standard table stakes that everybody has to have in order because that's the expectation of what people want because it's so powerful and useful. And so it's, I think, of right now as being very likely to be similar to the kind of 2008 when mobile was brand new, and all of a sudden you had a computer connected to the internet in your pocket with a GPS location, and it took a few years to kind of realize what that meant for food delivery or car ordering services and stuff like that. But it's going to be, I think, a pretty quick wave towards the innovators that are recognizing the opportunities that are unlocked because of how powerful these models are.
Erin - 00:27:33: You mentioned your roadmap shifting a bit. Is there anything you can tell us about that you didn't already? Any sneak peeks or anything you're excited about?
Mike 00:27:39: Yeah, I mean, I've mentioned a few of them, but I would say we're pretty early, frankly, on a lot of these applications as well, and we're just in the middle of trying to make sure that the applications of them are actually useful and not just buzzwordy for the sake of buzz. It's like, "Oh my gosh, it could do that!" and it's like, "Yeah, but why?" That's kind of the main thing I think everybody right now that's getting shifted by these, that's getting the roadmap shifted needs to focus on. And so it's very clear for us at a theoretical level that matches these problems we've known that our user base wants, solves from the beginning of grain. And so I would say there are probably two levels at which we're working on it right now. One is at the individual interview level of just trying to make those easier to parse and to share and to break down other component parts. And kind of the core value of Grain has always been this easy sniping and highlighting that you can embed anywhere that kind of unlocks these pieces to be shipped around on their own and atomized. And so that's like one part of it. And then the other part of it is kind of aggregating across many different conversations. And so whether that be a specific kind of research project where you're talking to 10 people about using the same interview guide or just more broadly, generally, the fact that sales is talking to customers and generating insights on a daily basis. Being able to actually identify those moments that are probably less interesting to the sales team and more interesting to say, the product team, and then be able to actually atomize and distribute that content to a larger group of people. But there's definitely this other kind of thing going on right now, which is about this democratization of this information and research being less of a formal process for most companies and more of an alignment exercise around the voice of the customer or the user. And so that's kind of another application that we are definitely seeing and working towards - being able to just free up, identify, free up, and distribute those moments and kind of aggregate those patterns that can ultimately inform the decision making. Because that's really what it's all about - we're trying to understand a really hairy, difficult, qualitative problem so that we can make decisions around our roadmaps or around our copy or around whatever it may be, and that those decisions are represented in rooted in reality of the people we're trying to serve.
JH - 00:30:04: Yeah, I like that. We've been on a pretty positive kick here. We think we're all optimistic about some things here, but if you are following the discourse around AI, there are some people who are less enthused and I think some real concerns. Any thoughts on like where researchers might get burned as they try to leverage some of these things? There's the whole "take our job" piece, which I don't think we think is all that likely, but there is, you know, as Erin is touching on, like maybe bias in the models that you're unaware of and it's pulling stuff out that you wouldn't have done it the same way. Or, I think what you see in a lot of the ChatGPT stuff or Bing stuff that gets kind of viral is like it's very confidently wrong, and so it seems like it knows what it's talking about. It's kinda like a bullshitter, and you're like, unless you know about the subject, you wouldn't maybe know that. And so like, where are some of the ways that maybe this could go wrongly so wrong for researchers in the short term?
Mike - 00:30:48: Yeah, I think there's definitely a lot of areas where it could be problematic. One that probably comes to the top of mind is, I'm not sure if you're familiar with the author Cathy O'Neill, but she wrote a book called Weapons of Math Destruction, sweet little play on words there. The premise of the book was a recognition that algorithmic approaches to problem-solving, while they're fantastic in a lot of ways and can really help us to solve especially scale problems, they can become destructive and problematic in themselves, largely because they are representative of the bias of the programmers and the algorithm kind of weights themselves. She goes into just like dozens of different instances around test scores or policing and using like algorithmic approaches to trying to understand where a police force should put, you know, the police in different parts of the city. And then you're like, "Yeah, but that's just reinforcing because the data is that, you're sending more there," and it's just going to increasingly send more police presence to the place where there already was police presence, as opposed to really maybe solving a problem and distributing it to solve the real underlying issue. And I think that that is absolutely a concern that there is, that we start to kind of remove some of that objectivity and just get almost immune or kind of ignoring the underlying bias that's there, and that maybe even conflicting to our underlying instincts that we have as the builders, as the people that are, you know, tasked with understanding this reality. And so then the other part of it I would say is like, I would say it kind of builds on that. It's not just about the bias, but in particular just the belief that it's right and it's correct because there's a certain amount of kind of intellectual rigor that is, that I could see, that I would personally have fear that starts to just kind of get outsourced. And I think that's why teachers are afraid of ChatGPT. I don't know what percentage of the user base the ChatGPT is like high school kids and college kids, but I think it's a lot, and there's a good reason why they're concerned with that, because you're removing the underlying rigor that generated that outcome. And inevitably, over the next five to ten years, academic institutions are going to have to figure out how to play with these tools as opposed to against them, because they're going to be the arm and extension of what this rising generation of students is going to use to solve the problems that they have. But the fear is that if you never develop the underlying ability to write effectively or write prose, you kind of lose those benefits that you can gain as an individual. And I think there's probably a similar worry that could occur in this world of trying to understand qualitative problems or user base, is that we start to just kind of trust the output as opposed to really looking at it with rigorous eyes and using it as an aid to draw our own work that we're doing where we are still in charge and control, and then you start to kind of trust the output as valid, even though it oftentimes either could be factually incorrect or incredibly biased based on the model.
Erin - 00:33:49: Yeah, it's very interesting just thinking about that balance of how to teach fundamentals versus relying on machines. I'm just thinking about math and calculators versus having number sense and knowing how to add. What is that right balance, right? Because everyone's using a calculator or computer for all of that, but some baseline of number sense is obviously quite useful as well. And I think all of those calibrations will probably be changing as technology just changes and has more and more impact too.
Mike - 00:34:14: I think the calculator's a great example. I'm sure there was an uprising amongst grade school teachers, math teachers against the calculator.
Erin - 00:34:21: With our pencils in their long division. How dare. Yeah. A hundred percent.
JH - 00:34:25: But to your point about the sense and the fundamentals, like I think you can build off that, right? If you maybe don't know math super well and you're saying, "What's 12 times four?" and it comes back with like a hundred thousand, I think most people would still be like, "That feels off." You know what I mean? And so there is a little bit of like being able to calibrate and gut check it somehow. I think what I've seen in just like the beta features we've been using with grain is generally pretty dang good, but at least the way we are applying it is that every time it's generating some output that's representative of an idea or summation, it, at least on the principle of our design, has to link back. We are forcing the model to say, "cite your source." Like what point in the transcript did that come from? And then there's the ability to kind of tie it back and actually say, "Okay, maybe the question is summarized in these three sentences, but it's actually composed of 10 sentences in the transcript." Being able to preserve the relationship between the source material and then the summarization of that, I think is an important thing to kind of preserve, to be able to have a reality rooting and not just kind of this outsourcing of like, "I'm sure the calculator's right," because calculators, for example, have been around for a long, long time. We can trust them pretty regularly to be better at math operators than we are. But I think these applications of LLMs in a more qualitative capacity are not quite as trustworthy, and I think there is no actual assurance that they ever will be.
Mike - 00:34:42: I think what I've seen in just like the beta features we've been using with Grain is it's generally pretty dang good, but at least the way we are applying it is that every time it's generating some output that's representative of an idea or summation, it, at least on the principle of our design, has to link back. We are like forcing the model to say, "Cite your source." Like, what point in the transcript did that come from? And then there's that ability to kind of tie it back and actually, like, "Okay, maybe the question is summarized in these three sentences, but it's actually composed of ten sentences in the transcript." Being able to preserve the relationship between the source material and then the summarization of that, I think, is an important thing to kind of preserve, to be able to have a reality rooting and not just kind of this outsourcing of like, "I'm sure the calculator's right." Because calculators, for example, have gotten to have been there for a long, long time around. We can trust them pretty regularly to be better at math operators than we are. But I think these applications of LLMs in a more qualitative capacity are not quite as trustworthy, and I think there is no actual assurance that they ever will be fully there.
JH - 00:35:52: Right. And the math stuff is deterministic, right? Like there's a right answer, like when you do this operation, it's supposed to put this out. And if it's not, you can just say it's wrong. Whereas a lot of the large language model stuff is much more probabilistic and kind of subjective. Ask it this prompt, and there's actually not really a right answer. And so it's like, how right or truthy is this thing? And it's definitely a little messier.
Mike - 00:36:10: A hundred percent.
Erin - 00:36:10: Mike, what are you excited about in the future?
Mike - 00:36:12: I think I'm excited about the creative work that can be done with more powerful tools that can get rid of a lot of the busy work that I know I spend a ton of time doing, and I know a lot of people do. And that it really is an empowering, enabling thing for me as a creator of products and ideas that feels like I can just kind of do more and oftentimes with less. I would say that's probably the thing I get most excited about. And then I feel like when these tools are being applied in the right way upon solid fundamentals as we were talking about, you're probably making the ability to create better things that are more user-centric and user aligned, easier to do because the actual good practices become more democratized and ubiquitous, instead of kind of the same traps that founders or product builders or researchers or marketers or whoever it is, kind of fall down until they learn better from making mistakes. I feel like that is kind of exciting to me to be able to just spend more time in creative mode and less in monotonous, busy work that can be outsourced by my a hundred high schoolers.
Erin - 00:37:20: Nice, awesome
JH - 00:37:23: Yeah, totally. A lot of exciting stuff there.
Erin - 00:37:25: Well, thanks for being with us. Like JH said, we love Grain, happy customers, and excited for the future.
Mike - 00:37:30: Awesome. Thanks for having me. It's a lot of fun.
JH - 00:37:32: Cool, take care.
Erin - 00:37:35: Thanks for listening to Awkward Silences, brought to you by User Interviews.
JH - 00:37:41: Theme music by Fragile Gang.
Episode Video
Creators and Guests
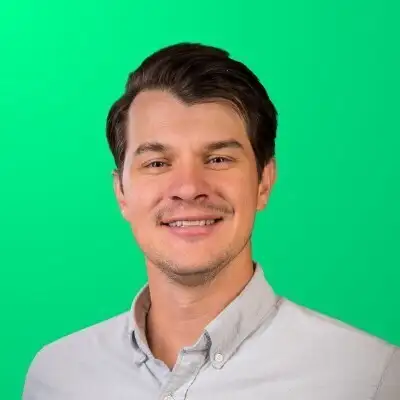