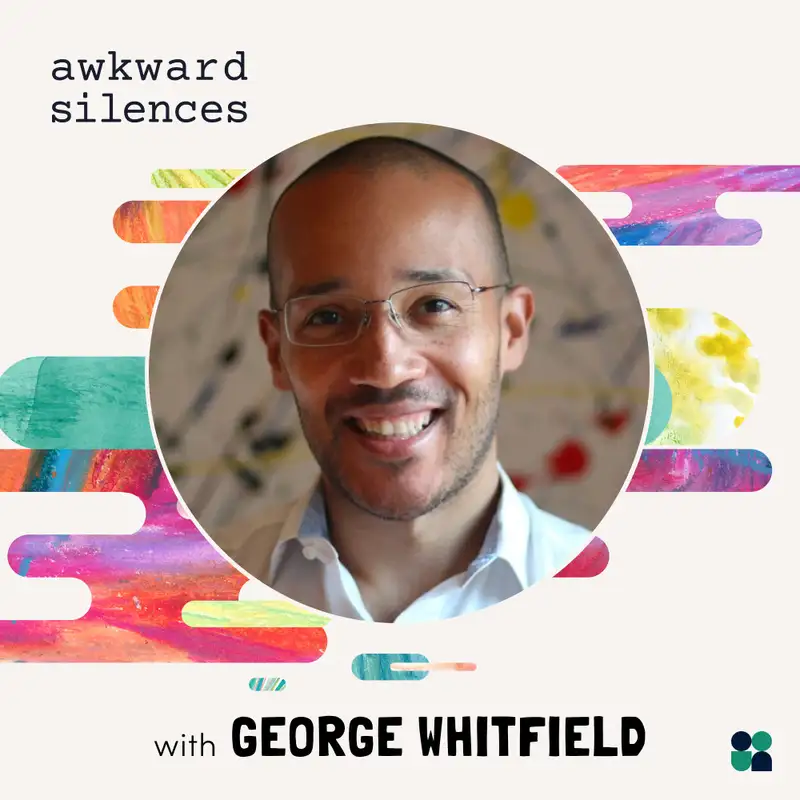
#143 - Harnessing AI For Better Insights with George Whitfield of MIT and FindOurView
George Whitfield [00:00:00]:
You can toss these like, really broad questions in there, but don't expect it to come up with anything that's like really earth shatteringly profound. It's just going to be like a happy go lucky little helper bot that's going to have some naive perspectives on things and some really very surprisingly well informed perspectives, but not necessarily making the right call from the expert judgment of a user researcher. So it's inspiration, not guidance or leadership, I would say.
Erin May [00:00:32]:
Hey, this is Erin May, and this is Carol Guest. And this is awkward silences.
Carol Guest [00:00:39]:
Awkward Silences is brought to you by.
Erin May [00:00:41]:
User interviews, the fastest way to recruit targeted, high quality participants for any kind of research. So this episode is going to be with George Whitfield, who's a founder and a lecturer and a PhD, and super knowledgeable about AI to do great qualitative analysis. I really enjoyed thinking about how you can actually sort of prompt better insights by kind of feeding the model with your hypotheses up front. So it's not just sort of spitting out random stuff it notices, but can actually help you to confirm or not confirm those hypotheses. So I think that's a really cool approach. Yeah.
Carol Guest [00:01:23]:
And one thing that I've been thinking about a lot in I've been a bit skeptical about using AI to do any interview synthesis because you'd think it would just spit out a bunch of not useful information to your point. But one of the points that he made was treating the AI like sort of a third person interview with you, the interviewer, and just the way you might synthesize the interview afterward with a notetaker or someone else on your team. You can use the AI summary also as another person to do synthesis. So I thought that was a nice way to think about bringing AI into the process, but not maybe wholly delegating the synthesis.
Erin May [00:01:55]:
Awesome. So this is a fun one. Hopefully it'll be good for all you who are doing qualitative research in your roles and check it out. Hello, everybody, and welcome back to awkward silences. Today we're here with George Whitfield. George is the entrepreneur in residence at the Martin Trust Center for MIT Entrepreneurship, lecturer at MIT Sloan School of Management, and the CEO and founder of FindOurView. We're very excited today to be talking about what we're always talking about these days, AI. But AI as applied to analysis of qualitative research, which we know a lot of you listening are thinking about.
Erin May [00:02:35]:
So thanks, George, for being our guest today.
George Whitfield [00:02:38]:
Thanks so much, Erin, for having me. It's a pleasure to be here.
Erin May [00:02:40]:
We've got Carol here, too.
George Whitfield [00:02:41]:
Carol as well. Great to be here with you, too.
Carol Guest [00:02:45]:
Great to be here as well. And excited to talk more about AI and qualitative research.
George Whitfield [00:02:49]:
Awesome.
Erin May [00:02:50]:
Well, give us a little background, George. How did you get on this path and what made you start thinking about AI and using it to synthesize large volumes of qualitative data?
George Whitfield [00:03:00]:
Yeah, absolutely. Well, I've kind of been passionate about building software products ever since way back. It's what I studied at MIT software engineering and just enjoyed coding. And I had my first hand at trying to build a startup company in grad school because I saw buddies and mentors of mine who had done it, and I thought that, wow, this is a great way to impact the world. So I went into a couple of different software roles. I led a software team in an electric vehicles company for a number of years, launched a platform that scaled up. It's a SaaS platform to help people plan for their ends of life. And then I launched this new company that I have because I realized that there's this potential that we have when we're talking to each other online, when we're studying how people respond to us in large volumes.
George Whitfield [00:03:48]:
This potential to connect, that kind of gets diffused and lost as the numbers go up, as we talk to more and more people, we kind of lose that personal connection. And I wanted to find a way with technology to keep that fresh, to keep that really in context. And so I searched in a number of markets, and it wasn't user research initially, it was sort of consumer insights initially. We launched a product there that we sold to the New York Times and other media companies to help them understand consumers. But as we did more research of our own, we realized that this process itself really could be helped by AI. And furthermore, if we can help people who are doing research, we're going to impact so many more problems, so many more innovations that are coming out that are really trying to honor and understand what customers need, what markets need. So that was kind of the inspiration. And like I said, software and AI has always kind of been one of those tools in the arsenal that I've kind of been thinking about.
George Whitfield [00:04:42]:
How do we position that as we go forward? Awesome.
Erin May [00:04:45]:
Yeah, I'm sure it's very close to the hearts and minds of many of our listeners, but maybe you could tell us a little bit about what are some of the particular challenges when you get to lots of qualitative data, you've talked to lots of users, lots of customers, and you want to make sense of it, right. We often talk about how people should be talking to more users. They should be doing more research. But of course the purpose is to get insights. So what challenges come up when you're dealing with large quantities of qualitative data?
George Whitfield [00:05:15]:
Oh my gosh, so many challenges. Well, let's see. First you got to shake off the jitters and get out there and do the research. So if you're a user researcher, you've embraced that already and you're ready to get the tsunami of information that can come your way. But the funny thing is it takes some getting used to that sort of mode of operation, that sort of rapid iteration. And that's part of what we teach. When I'm teaching at MIT, we're teaching founders kind of to get in that rhythm and to say, hey, you really need to get first hand data, primary market research. So what do you do when you've now unleashed the wave of information and you've talked to customer after customer and you've got 10, 20, 50 interviews to try to make sense of? I mean, on the one hand, every interview you're trying to get the little incremental insight out of that and sort of carry that forward and not lose that and really be diligent about what's coming out of each one.
George Whitfield [00:06:08]:
So that sort of short cycle input or output is really important. And as you amass more and more interviews, then you've got this kind of emerging forest from the trees that is sort of coming together too, and then trying to put two and two together across 5100 data points and to find out what are those unexpected things that have emerged. So the challenge that I see kind of when you're in that rhythm, it's kind of both the short cycle and the longer cycle to get individual interviews kind of properly digested and put into an immediate perspective. But then as you get higher volumes to try to figure out what is the overall trend, what does it mean in terms of what we thought going into this study? And then how should we maybe shift strategy or shift perspective going forward?
Carol Guest [00:06:55]:
Often when we talk to researchers and members of our audience, we hear a lot about the amount of time that it takes also to synthesize all that data, often one of the longest parts in the research process. And so I'm sure people are broadly very excited about the idea of using AI to support that synthesis, but maybe have, maybe skeptical as well. So I'd love to hear more about where you have found so far that AI is really good and effective at supporting analysis and maybe where it doesn't do so well.
George Whitfield [00:07:21]:
Yeah, really excellent question. I think a lot has changed in the past two years, clearly, obviously, because let's say more than that. Like three to five years ago, we didn't really have the maturity of the language models that we have. And now it's just increasing at this crazy pace. It's just exponential on top of exponential. It's amazing. So the challenges a couple of years ago are different from what we face today. A couple of years ago it was like, well, it's not that great.
George Whitfield [00:07:49]:
You'll have some word clouds to kind of generally keyword match things. Or maybe there was just this start of semantic similarities so you can kind of figure out some topic modeling that started to get good. And then after that the sort of emerging challenge became, okay, how do we actually cut across context and not just words or topics, but actual sort of full descriptive context about when somebody said something that became a theme? How do we place that in the surrounding themes? What's the relationship to other themes that came out of this? But now I think there's a new type of challenge. So we've got basically language models that are very good at identifying context, can give you this semantic similarity. You can kind of identify themes, clustering, that kind of thing. But if you're trying to figure out what to do with that, there's also problems of making sure the model is itself accurate and isn't hallucinating. So let's say you've got some data, you're going toss it into chat GPT or something like that, right? One of these language models, and then you want to ask it to kind of help you figure out what are some key takeaways from that or what are some key outputs from that. For one thing, it might hallucinate.
George Whitfield [00:08:56]:
So we have to be careful about that. There need to be safeguards against that. But also another challenge that I see in a lot of tools that are now kind of sort of building that into the workflow. Building in like key takeaways from your interview is that it doesn't yet have all the time, sort of the agency that you brought to the interview. So whatever key takeaway pops out might be out of context from what you intended, right? It's going to say, okay, well, here's some key takeaways. And you'll look at that and be like, well, sure, that's generally what we talked about, but I've got an agenda here. I'm trying to test these specific hypotheses. I'm trying to understand what my customers need, and this is not capturing that yet.
George Whitfield [00:09:31]:
I can't just pop those out of my transcript, my interview, and then have that be the result of my research. So we tried to take it a step further, but like we said, I'm a founder at a company called find our view, and we basically inject into the process the intentions of the interview, the hypotheses you're testing, and explicitly state those. So that runs through the transcripts with AI, so that what comes out are not generic key takeaways, but are validations or invalidations on your hypotheses. And to address that hallucination problem, we then also pull quotes as evidence from the transcripts and bookmark those in the transcripts, too. So now you've got, okay, specific research agendas that were satisfied and then specific points of evidence, put a rationale on that, explaining, try to address sort of explainability around it, tell it why it validated that, and then finally put it all together to sort of see the forest. There's this approach then of just having all the interviews kind of summarized so you know what was validated or not. So how many percentage of customers are validating something or what percentage, maybe, of interviews didn't test that hypothesis, and you need to go back and reiterate or retarget your next interview. So those are both the challenges that I see today and also how, in our case, we're aiming to tackle that.
Erin May [00:10:45]:
Awesome sort of baking in the kind of prompt engineering right into it in terms of don't just give me some information, but really do it this way. I have an agenda here. Let's save everybody some time. And don't give me your generic. You don't know what I know. I'm going to tell you what I know, and then you're going to work for me and really help me find some actionable insights. We'll work together on this, you and me. The robot.
George Whitfield [00:11:09]:
Yeah, that's exactly right, Erin. And I'm glad you mentioned prompt engineering, too. That's another really important part to consider. Rather than just kind of diving in and just letting it, the model, have its way with the data, we need to make sure we set it up with the appropriate assumptions in the context of user research. So that's another aspect of it, like having a really good set of instructions that sort of takes a step by step approach, asking it to check itself, asking it to. Sometimes you could even ask it to assume a certain set of standards to apply to the data to. So there's different ways of approaching that to try to get it to produce a better result.
Carol Guest [00:11:44]:
When I think about using AI to do something like synthesize an interview that I've done, think about some of the interviews that I've done recently. I think my fear in doing so is that I would end up doing the work twice. Right? Like, I would feel like I both had to interpret the interview myself just to make sure that I picked up on directly what people were saying, as well as some other key needs that maybe they didn't say. And then I would also have to go and look at the AI and make sure that it was correctly categorizing some of the insights. So I just wonder, how do you think about the human in the loop and how to make sure that the human is supporting the process as opposed to maybe double checking the process or doing it twice?
George Whitfield [00:12:21]:
Oh, yeah, excellent question. I think we always need to have human in the loop. It's never a hands free process, at least what I always would recommend in terms of using these types of tools. So basically, firstly, going into it, expect that you're still going to be looking through the data, but when you have some AI to work with, I would recommend that as a jumping point or a draft or a preliminary analysis of something. So at best, I would say expect to look over this tool's shoulder and just sort of like check through and make sure that what you anticipated is coming through. And there's certainly a transition time where when you first start to adopt a tool, where you're going to want to be kind of a bit more of a micromanager on that tool. And I actually use that word intentionally in the sense that the way we treat these tools, I would recommend treating them actually as sort of integrated in the workflow, as if you were sort of managing a person doing that job, in a sense, because there are some really interesting similarities between how the tools work and how people work. It's like they're approximations.
George Whitfield [00:13:25]:
They're not guaranteed to be able to give you the right answer or even the same answer every time, but they can be trained to recognize certain types of patterns and carry out things with instructions. And every once in a while, they might think they saw something and then make something else up. Right. And we call that hallucination. Right. So when you're first adopting it, I would say watch much more closely, kind of micromanaging what it's coming out with. But then as you start to see and confirm, okay, this is consistent with my thinking. This is sort of indeed what I was expecting based on my own analysis.
George Whitfield [00:13:57]:
And that could even mean like in the beginning, the very beginning, just still doing the manual analysis and then just comparing. I recommend that. And that's how we also often pilot this as well. Once you get comfortable, then you can kind of start to back off a little bit but still keep an eye on it. And whatever system you use too, it should have something that allows you to correct it too in real time so that you can hop in and edit, annotate, put comments on there so that it's not just this system sort of running on top of its own AI and carrying and propagating that without any human intervention.
Erin May [00:14:27]:
Does the correcting then make the AI? Obviously this will vary by tool, but does it then make it smarter over time? Or is it sort of incumbent on me to just get better at learning what the tool is good at and what it's not good at over time and how to best use it?
George Whitfield [00:14:42]:
Yeah, for tools that I've seen and including ours, it would be a longer cycle of learning. I think it'll speed up over time as different tools kind of mature and they're able to kind of adapt in real time. But sort of the assumption going into any new tool should be that it's not going to correct for me automatically. It's not going to necessarily adapt unless it's explicitly saying this is a new feature we have. It's going to do this thing right. But tools like, let's say you're hopping into just large language models like chat, GPT or Claude or BaRt or any of these that you might be using, it's going to remember context in a single conversation. It's not going to, as you have many conversations, many points of synthesis, get better at synthesizing your data. You're going to have to refeed it, reprompt it, reprime it for that exercise.
George Whitfield [00:15:34]:
And those companies that run those, indeed, they will say we're making our models better, but they're making everyone's models better. Right. And that takes a long time. So that's still, I think, an opportunity for other companies who are kind of more specialized to try to shorter cycle, get that to adapt on a per researcher basis.
Erin May [00:15:52]:
Yeah, absolutely.
Carol Guest [00:15:55]:
That's a good.
Erin May [00:15:55]:
Oh, go ahead, Carol.
Carol Guest [00:15:56]:
Oh, I was just going to say that you mentioned about treating the AI like an employee or heard, you know, treat the AI like an intern, where you need to monitor the work. And I think one of the things you mentioned that your tool find, our view does that seems particularly valuable is that you can see the direct quotes so that you can look through each of the quotes and see if it actually does apply to the hypothesis as you mentioned, and then maybe also realize that there were a few other quotes that were relevant but didn't show up. Right. So that's sort of like a way of checking the work is by going to the ground truth of the direct quotes, I guess. I wonder if you have any other thoughts on ways that you can ground truth the work as you're going through it.
George Whitfield [00:16:33]:
Yeah. Excellent. So I think one way I would say is try to go through this as quickly as possible or as, as possible after the interview. Like, well, it's fresh in your mind. And I think this is the rule of thumb. In general, I would say, for a synthesis, is to just let the back of the interview go jump into it. Now that we've got AI that can kind of crank through that in the matter of minutes, it now becomes something where you could have it there alongside your fresh recollections. And that'll just make it that much easier for you to go through the data and to catch those things that are missing, other things you can do.
George Whitfield [00:17:06]:
Well, this kind of does zoom out a little bit into tactics for user research, but I would say take a buddy, not just an AI budy, but a real buddy, right. And check each other's biases, each other's assumptions. I'd always say have one person doing the talking, one person kind of observing, and maybe like taking some notes or something like that. But when you put AI in the mix, then now you're both checking that and you're both kind of having the gut reaction and seeing where it landed. And maybe the third thing I would say is engage a broader discussion. So not just with you and your individual budy, but once you've got some results, try to put that to other stakeholders and kind of make sure that it's correctly aligning with what they are hearing from other angles. And so it's important to bring that data into the broader conversation. And I think that's where we see the more traditional tools help quite a, they're sort of designed to sort of do that because they have shareability, or you can make a page that kind of puts a digest together that's kind of a little mini report or something like that.
George Whitfield [00:18:03]:
Our angle on that was, it's interesting, although the reports in theory, that should make it shareable and easy to go through, we did hear from a lot of people in our own interviews that often they'll write reports that nobody ends up reading. It's just kind of getting filed away somewhere. And nevertheless, it's really important to get people's feedback. So idea we had was, hey, let's build a little chat interface so that, let's say you're in slack. You can kind of interrogate some of the results and talk about them that way. So it's going to draw upon your user research that you got all those results that you synthesize that are in the platform. It'll then bring that into slack. So you can now sort of add this little chat bot and say, hey, what did we learn in this last study? And it'll tell you that, but then your colleagues can then jump in there too and talk to it, talk with you and try to understand what's going on.
Erin May [00:18:49]:
Awkward interruption this episode of Awkward Silences, like every episode of Awkward Silences, is brought to you by user interviews.
Carol Guest [00:18:57]:
We know that finding participants for research is hard. User interviews is the fastest way to recruit targeted, high quality participants for any kind of research. We're not a testing platform. Instead, we're fully focused on making sure you can get just in time insights for your product development, business strategy, marketing and more.
Erin May [00:19:15]:
Go to useernerviews.com awkward to get your first three participants free.
Carol Guest [00:19:20]:
So it sounds like part of, I'm just thinking about this workflow. It sounds like part of what you're describing is the common process where right after an interview you grab a buddy who is there, you do a quick synthesis, take notes, do a snapshot, however you do it, and share that out with maybe your relevant team. You're saying at that moment in time, that might be a good point to first run the transcript through an AI, get the summary notes from the AI, just to double check that it's tracking the insights that you're tracking, same as the way you're checking with your buddy that they saw the same things that you did in the interview. And that way when you get to the later, more broad summary of all of the separate interviews, you can at least be confident that on an individual interview you saw things the same way. And then the sort of summary you might see the same way. Is that sort of the process you're describing?
George Whitfield [00:20:04]:
Yeah, that's spot on. And I think I'll just add one more thing, which is it'll save you rather some time when you get to that big sort of forest of data. But I would also suggest even as you're talking with your budy, like, start the analysis right there whenever it lands let that sort of impact your discussion too, because if you've built that sort of trust in the tool that it's going to be able to produce those results, then that's an opportunity immediately for you and your colleague to both speed up the time that it'll take you to kind of tag the relevant things and just make sure that you're catching the important points. But it also sometimes can catch things that you just wouldn't have had time to find, too. And by having that at the table when you're doing the synthesis too, it'll just kind of deepen and enrich the discussions that you're having in the moment so that that becomes part of the digest that you then send out. So we really do recommend having it as early as possible in the synthesis process so that everything that you sort of go through and discuss and then share out is enriched from it.
Erin May [00:21:02]:
George, what are some research methodologies? Use cases where this becomes really useful? Of course, any research with qualitative data you could imagine could be relevant here. But is it more kind of study by study, session by session? Are you going across larger data sets across multiple studies? Where does this work?
George Whitfield [00:21:22]:
Well, so the way we frame it is really study by study. That's actually what we call it on the platform. So you define a study, it's a group of interviews, and imagine you've got a cohort of customers that you're going to interview for some particular reason. And there's a variety of reasons, just like you said. So it could be, let's say you're a founder. Well, you're going to do studies where you're going to interview a group of end users or potential end users to try to test a market need or something like that. Right? So you might have ten people that you reached out to, you booked interviews, and now you're going to do them back to back over the course of a week or so. So that would be like a study, but there's so many other use cases.
George Whitfield [00:21:59]:
So let's say you now are a little bit more advanced in terms of the stage of the company and you've got a product and now you want to do some user testing. Well, if you're sitting there doing the testing in a live format where you're interviewing them and asking questions and getting responses, that's again an opportunity to test those hypotheses going into that. And as you're talking through them, anything that's verbally being confirmed or disconfirmed, you can again run through the platform and analyze so it's both useful for kind of a founder or early stage sort of product lead who's sort of investigating new markets, doing market research for the go to market strategy, which then impacts product design. So the second round on top of that, we often would say is make a high level product specification so that you can then bring that back to the customer. Ask them about that. What do you like about this? What needs to be added? What didn't you like? So that's sort of in the product design phase. But then for product management, it's really once you've got a working product and you're trying to make it better and you're thinking about what's the next features to release. You need to stay on the pulse of customer needs and to be talking to them.
George Whitfield [00:23:02]:
Right. So again, have your interview transcript ready to go there, your transcription tool, whatever you're using, and take transcript and then synthesize on the back of that. It's something that across those different contexts, obviously scaling up even further, you've got user research teams that are dedicated to drive those types of insights in a variety of contexts and it's immediately relevant to them as well.
Erin May [00:23:23]:
So you've given some tips already as we've gone to sort of review the work early of this more junior in their career employee robot that we're employing here. What are some of your top tips just in terms of being really successful with using AI and recognizing that the technology is evolving really quickly. So those tips might change two months from now, but what are you generally seeing makes people successful or not successful working with AI and analysis?
George Whitfield [00:23:51]:
I think first and foremost, knowing that this is all really new, there's a lot of potential, but it's certainly not perfect yet. And we can't just out of hand trust the results for a very new system that we have. So I always recommend there are many different ways you can use AI. And actually outside of research, we often think about this as being just a source of generating new content that also can impact the research process. So you can use it to get inspiration, you can use it to, let's say you have an interview script, you could use it to critique the script or to maybe get even a rough draft on the script. But don't just blindly trust what's coming out of it. We have to self critique as it's offering its counterpoint as well. So I would say use it for inspiration, use it for sort of assistance, but I wouldn't say give it the reins yet because there is still a lot of experimentation in this field, a lot of progress to be made still at the forefront of this.
George Whitfield [00:24:50]:
And it's something where we need to trust the experts, the user researchers who are in charge of the process, who have the expertise, the perspective and the experience to really be able to make the right decisions in the right context. AI, if anything, should be an accelerant, it should be an assistant. It should be something that is empowering every member of that research team to make the right calls and to sort of offload some of the more routine aspects of the work that they're doing.
Carol Guest [00:25:15]:
And then on the flip side, we'd love to hear any thoughts you have about where people can go wrong in using AI for analysis. You mentioned at the beginning that not having a structured hypothesis can lead to sort of a generic summary that might not be so useful. Anything else come to mind in terms of where people can go wrong?
George Whitfield [00:25:31]:
Yeah, I think we touched on this briefly, but hallucination is a big problem. Let's say you were to, again, toss this into one of those large language models and ask it to pick up some results without then going back and verifying that each and every one of the recommendations or observations that it made is correct. There is a chance that it could hallucinate. And even if you're trying to give it a really good prompt to make it not hallucinate, it just happens. Right. And it's never 100% accurate in that regard. So you have to add extra checks and balances and safeguards. And you might imagine that being something where, well, now I've got to go back and look through and check everything one by one by one again.
George Whitfield [00:26:10]:
But indeed, even that process can be automated if we're taking a research framework to the whole exercise. It's just that these big platforms, they're used for everything and they're not necessarily optimized for user research specifically. So it's not going to give you that sense of reliability that all the points are indeed validated and verified. And it's funny, I could even toss something into, I've had it before where I was tossing something into Bing and I'm chatting with Bing and then it gave me some references and it said, oh, and this is the citation of that. And then I tried to click on it and the link didn't work. And I said, can you give me that link again? And then I tried clicking and it didn't happen. Right, so it's literally hallucinating the reference. Right.
George Whitfield [00:26:50]:
So we've got to get under the hood and check it and so those are some of the more engineering related tasks that user research tools and companies like what we built would take into account to try to ensure the accuracy of things. Other places where you can go wrong. I would say getting inspired by AI can help getting like, let's say you wanted to just get some suggested ideas. What might I test? Or what might be the hypotheses in this area, or what segments might exist in the market. Right? You can toss these really broad questions in there, but don't expect it to come up with anything that's really earth shatteringly profound. It's just going to be like a happy go lucky little helper bot that's going to have some naive perspectives on things and some really very surprisingly well informed perspectives, but not necessarily making the right call from the expert judgment of a user researcher. So it's inspiration, not guidance or leadership. I would say keep it out of.
Erin May [00:27:48]:
The leadership role and might be decent at answering what is average person, not that that exists. Think about what. Because that's sort of what.
George Whitfield [00:27:55]:
It's exactly.
Erin May [00:27:56]:
Not the smartest kid in the class, necessarily, but useful and helpful.
Carol Guest [00:28:00]:
I was just going to add, I imagine it might be, we've talked about in user research, sometimes we'll talk about sort of like the hierarchy of user needs, where it might be they're stating a feature directly versus stating a need that they have versus a member of a segment that has a type of need. As you work your way up, I imagine it's probably very good at the, this is a specific feature they have a lot of feedback on and increasingly less good at the why do they care about that area? Or why does that specific group of people specifically care about that problem?
George Whitfield [00:28:28]:
Yeah, I think that's right. Because to answer that question, you need so much more context on the behaviors of your customers, the demographics, the psychographics, the relationships that you've built with them and prior conversations you've had. That's all what you're considering, to try to infer that. And over time, we can try to put more and more in there, but there's still something missing there. There's still the perspective, the judgments that you're bringing in there to be included. And I don't see any tool today filling that need at all. So I think that's where the role of the research or the responsibility of the people who are leading those research efforts comes into play.
Erin May [00:29:06]:
Anything out there that can analyze body language? I know you hear that a lot, right? Researchers, when they're speaking with the participant, you're looking at the words that are being said. That's the sentiment analysis, the language models. Right, the words. But there are a lot of visual cues happening as well and a lot of research. Is there anyone attempting or successfully analyzing those kinds of cues as well, or is that kind of. That's the researcher's job for now, yeah.
George Whitfield [00:29:33]:
That'S such a good idea and question. I know of one company, not in the context of user research, but does analyze body language on Zoom and for the purpose of personal feedback, to give me advice as to sit up straight and try to make more eye contact with the camera or something like that. Right. But for the purpose of user research, I haven't seen that yet. It's certainly a fantastic idea, and it would be something that, as we grow, that's something that we would want to add as well to ours. And I think it's a very likely frontier that we'll see more and more user research tools adopting as they go forward.
Erin May [00:30:09]:
Yeah, I would think so. So one thing we've talked about is sort of like the etiquette of AI as it becomes more prominent in all of our various workflows. And so, for example, important to be mindful of hallucinations, bad facts and insights coming from the AI. But you've got your analysis that you feel pretty good about, that you've gone through and given that human check, and now you're doing your readout, any kind of sharing of these learnings with your stakeholders, do you share that AI helped put this together, was part of this loop at all? Is that kind of caveat necessary or welcome, or how are folks? Or do you recommend sharing that or not sharing that information as analysis gets shared?
George Whitfield [00:30:52]:
Yeah, that's a really good question. I think it does depend the response you might expect from that. It's like an internal cultural thing to the company, too. So I think we're going to see variation across industries. I think that typically, in terms of user research, we're expecting that user researchers, they've got a range of tools in their arsenal that are going to enable them to tackle that data and to get their heads around it and to digest it and get the most out of it. Right. So in that regard, I see it as a very good thing that they are aware and embracing and engaging with the latest and the most suitable tool to their needs. So I think what I would expect to be the case is that as you adopt a better tool, it should give you a boost, it should give you a bump.
George Whitfield [00:31:37]:
And the results coming out of that should come forward likely. We might expect it to come forward faster, potentially, or this is really the call of the user researcher to make. You might just be going deeper into the data, right. And just kind of going through more thoroughly and just discovering a lot more and more things, a lot of interesting results, right? So whatever the results are from using that tool, they're going to be embodied in some way in the work product. Either maybe you went faster or maybe you went deeper, or it's just really confident in that decision. So I would say it's your call whether or not to give it the stamp of assisted by AI, because it's something where the way I see it, it's like, of course you're using AI because you guys are just really savvy. You're going to use whatever is appropriate, whatever you make the call on. And if you do decide to put it sort of front and center, then I would do that kind of, if you wanted to maybe strategically invite other people to engage, too.
George Whitfield [00:32:29]:
I think that's kind of sort of the pull that I would see if that were something that you were considering doing. And the best example I could think of is what we do, which is having the AI actually in the output, attached to the output of the results so that you could chat with it. And that's something that's, I think, pretty new. It's something obviously we can chat one on one with a chat GPT, but having a bot that's ready to chat with your entire team and with the data to help you digest through it, that does kind of introduce the hey, AI is doing something here stamp. But also in doing so, hopefully opens up a broader discussion and productivity in terms of having everybody rally around that data and really start to internalize and feel like those results are results I can get behind. Because again, that's one of the biggest challenges that I heard. It's like you do a study, you have this amazing insight around it. How do you get people to appreciate the impact of it and having them to have this ability to get up close and personal and talk with it and to really relate with it gives them that opportunity.
Erin May [00:33:28]:
Yeah, I love how it kind of gives the research a second life or keeps it alive after the research is done. That interaction, it's very cool.
George Whitfield [00:33:35]:
Absolutely. Whatever the data source was, whatever the customers were, the users were that we were talking with, like to continue to carry forward their voice, I think is a really powerful thing. There might be amazing insights you had in the course of the conversation, and a lot of them got summarized, but then maybe some of them weren't summarized, but they were still really good. And having that still at your disposal can be a really powerful thing.
Erin May [00:33:58]:
So you've been thinking about AI and qualitative data for a while, first within kind of customer insights and more in a user research context recently, what are you excited about for the future? No one knows what's going to happen, but what are you starting to see that is exciting to you?
George Whitfield [00:34:12]:
Yeah. Wow, I think it's such an exciting space. One of the areas the frontiers is like with multimodal models now, we do have the potential to interpret different modes of communication and interaction so that it's not just analyzing a transcript. You can think about tone of voice and inflection, you could think about micro expressions and just like little subtle cues that could just help us understand and contextualize what was said in the research specifically. I think that my hope is that with this type of technology that we will finally have a tool that is able to sort of do justice to interpreting things in context the way that we might, such that as we scale up and grow to much larger sets of conversations, that we can have the help of the tool for us to get our heads around those data sets. So it speaks to user research one on one, but it speaks to so many other contexts of discourse and speaking with people that we really sort of lose that personal touch, that understanding of what was meant by the words that were said in that context. So I'm excited about applications for that, in public discourse, for social discourse. But staying on user research, I think that these models are just improving at an exponential rate.
George Whitfield [00:35:33]:
And so the accuracy is going to go up, the speed is going to go up, and that little buddy assistant will get a little more helpful. And so I think being able to have sort of a more of a strategic guidance and to be able to trust it to do some of that work for us in the synthesis, should enable us as researchers to tackle so many more challenges or many more, let's say, cohorts that are bigger or problems that we thought maybe had a lot of nuance that just would have taken too long to go through. I think it's going to be really empowering for user researchers, and that excites me, because user research is the core of, I would say, any new tech innovation, any innovation that's going on, we need user research to be like the front lines of understanding customers. And if we can get AI systems that are helping them do their job faster and with greater confidence, that looks really great. For the future of innovation.
Erin May [00:36:29]:
Agreed. You're here. Awesome, George. Well, now it's time for our rapid fire section. So just a couple quick hit questions at the end here. What are a couple of resources that have been really helpful to you in your career that you think other folks might get some value out of?
George Whitfield [00:36:43]:
Yeah, sure.
Erin May [00:36:44]:
Books or articles or something you've written?
George Whitfield [00:36:46]:
Oh, yeah, absolutely. Well, this one is for, I think, all the founders or people working in like, a startup environment that's scaling, I would say, a book called Disciplined Entrepreneurship. It's written by a professor at MIT named Bill Olett, who is the head of the entrepreneurship center there at MIT, the Martin Trust Center. And it's the foundation of how we teach entrepreneurship at MIT. It's very structured and prioritized, and it's almost like, think of the engineering rigor that you have in sort of the tech part of the university. Well, that sort of level of rigor is applied now to building a business. And it's like, what types of hypotheses should I be testing at the beginning of the company versus after I've segmented the market versus after I've identified the customer need and then had the high level product specification? And it's sort of marching forward, testing ability for the market to have willingness to pay. As a startup founder, there's like a million things you could test, right? So that one really stages it out very systematically.
George Whitfield [00:37:45]:
There's another book coming out on the back of that, which is called venture creation tactics discipline entrepreneurship venture creation tactics. And that happens to be a class that I teach at MIT, books written by one of the other professors there by the name of Paul Cheek. And that is sort of taking entrepreneurship strategy fundamentals and now applying it to driving efficiency in operations. So if you're doing lots of primary market research, what types of approaches can you take? What types of tools might you use to try to speed up that process? Or your go to market strategy, or building digital assets, or hiring and product roadmapping. That's kind of what that is about. And I just give you one third book, which is really great for user research. I'm sure this is on your must read list. It's the mom test, right? Which is, as you're going out there to say, stay just like really scrappy, really nimble, and really open to being ready to engage in that user interview when the opportunity arises.
George Whitfield [00:38:38]:
And doing that in a way that's lightweight, it's not overwhelming, but it's still really intentional. And that all these resources, I think they play together really nicely because any good venture needs to be built on the back of strong user research.
Erin May [00:38:52]:
Awesome. Where can folks find you? Follow you? Engage with you online?
George Whitfield [00:38:57]:
Yeah, look me up on LinkedIn. That's probably the easiest George Whitfield my LinkedIn page there. You can search me there. You can also find my company at findourview.com find O U R view.com yeah, and hit me up. Always happy to chat, especially if you're sort of doing user research, just looking for input on a venture or just want to chat about startup life in general. Always happy to.
Erin May [00:39:23]:
Awesome. Thanks so much for joining us, George. This has been great.
George Whitfield [00:39:26]:
It's been a pleasure. Thank you. Erin thank you, Carol, thanks so much.
Erin May [00:39:36]:
Thanks for listening to awkward silences brought to you by user interviews theme music by Fragile Gangs Listener. Thanks for listening. If you like what you heard, we always appreciate a rating or review on your podcast app of choice.
Carol Guest [00:40:01]:
We'd also love to hear from you with feedback, guest topics or ideas so that we can improve your podcast listening experience. We're running a quick survey so you can share your thoughts on what you like about the show, which episodes you like best, which subjects you'd like to hear more about, which stuff you're sick of, and more just about you. The fans have kept us on the air for the past five years.
Erin May [00:40:20]:
We know surveys usually suck. See episode 21 with Erica hall for more on that. But this one's quick and useful, we promise. Thanks for helping us make this the best podcast it can be. You can find the survey link in the episode description of any episode, or head on over to useernerviews.com awkwardsurvey.