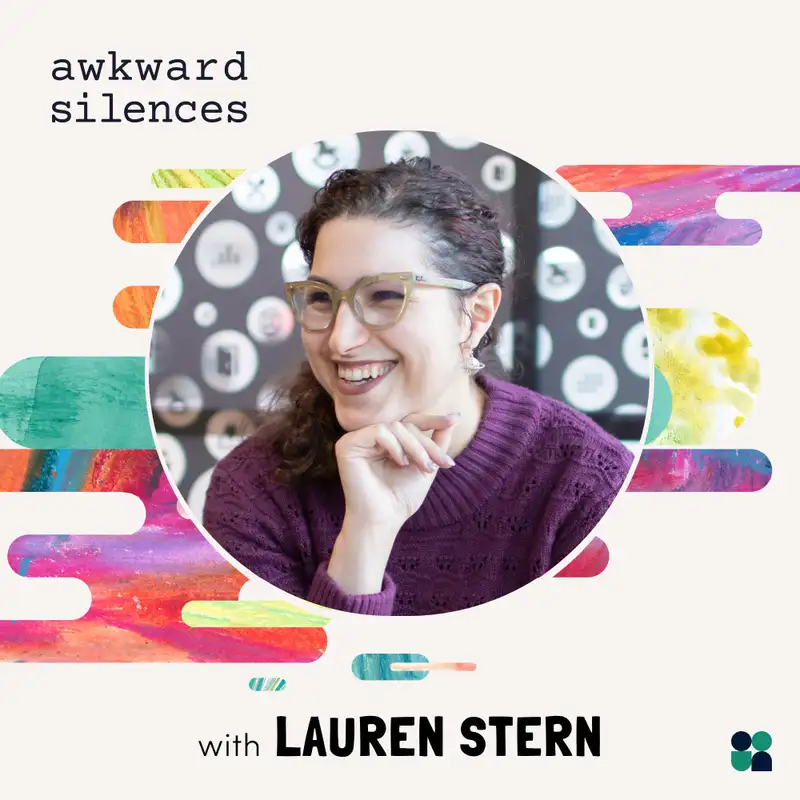
#144 - The Craft of Sample Sizes with Lauren Stern of WHOOP
Lauren Stern [00:00:00]:
I think that something I've seen a lot with more junior researchers on my teams has been being a little bit afraid to have a conversation around sample size. Like, it feels like something that either you're supposed to know as a researcher, like, you just should magically know what sample size you need, or that if you admit that you don't know that, you're going to get a lot of pushback from stakeholders, and then that becomes a whole other headache you have to solve. And so we just avoid the conversation altogether. And I wish that we talked more about sample size. That was why I was so excited to come on and do this. I think for researchers, it's really helpful to have the experience and the backing to know what resources are available to you to figure out the sample size, whether that's your personal experience, the experience of your team as a whole, the literature, calculators, there's lots of stuff out there. And at the same time, having these conversations can help then build the confidence to be able to go to a stakeholder and say, hey, I think the sample size that we need is X, but we have a little wiggle room. Does that number feel okay to you? Do you need it to be a lot higher or lower and engage in that in a way that's not going to spiral?
Erin May [00:01:07]:
Hey, this is Erin May.
Carol Guest [00:01:09]:
And this is Carol guest.
Erin May [00:01:10]:
And this is awkward silences.
Carol Guest [00:01:15]:
Awkward silences is brought to you by.
Erin May [00:01:17]:
User interviews, the fastest way to recruit targeted, high quality participants for any kind of research. Well, it was so great to have Lauren on to talk about sample sizes. I know we tease, like, you know, sample sizes is a nice, nerdy topic, but I love their nerdy topics. And in seriousness, what I love about this topic is that it's relevant to every single person doing research in every single study. You got to figure out how many people you're going to talk to or survey. And it's one of those kind of critical but easy to overlook pieces that can make a huge difference to the quality of your insight, to your budget, to the time it takes to complete the research. So I think it's pretty important and interesting.
Lauren Stern [00:02:03]:
Yeah.
Carol Guest [00:02:04]:
And it's great to hear Lauren dig in on all of the different methods that can contribute to different sample sizes and then also importantly, how to bring your stakeholder along with decisions you've made. So, yeah, really good conversation.
Erin May [00:02:15]:
Awesome. Hello, everybody, and welcome back to awkward silences. Today we're here with our special guest, Lauren Stern. Lauren is the director of WHOOP Labs at WHOOP. And today we're going to be talking about something probably all of you think about as part of just about every study. And if you're not, maybe you'll start doing that a little bit more, which is sample sizes. So I was looking for someone who could come join us to talk about sample sizes. And, Lauren, you were an enthusiastic hands up, so cannot wait to talk about sample sizes today.
Erin May [00:02:51]:
Thanks for joining us.
Lauren Stern [00:02:52]:
I'm so excited to be here. I love sample size. So happy to talk about it.
Erin May [00:02:56]:
Amazing. We've got Carol here, too. Hey, everyone.
Carol Guest [00:02:59]:
Glad to be here and excited to dig in on this topic.
Erin May [00:03:01]:
All right, so we are going to focus on sample sizes, as we've discussed already, Lauren, how did you develop an interest in knowledge of sample sizes?
Lauren Stern [00:03:10]:
I would say that my passion for sample sizes comes from a couple of different areas. One is that I've historically been a mixed methods researcher, and I have, as a result of that, worked both on more quantitative projects where I was doing statistical analysis. I needed to be able to calculate significance and whether or not my sample size was high enough to be able to show the things that I was trying to prove or disprove. And at the same time, I've worked on very labor intensive studies that are more longitudinal, where we needed to be able to balance the number of participants that we included and the amount of work that had to go into that. I've also, as I've moved up and worked on more complicated logistically projects, had to balance things like how many prototypes do I have available that I can test with the sort of resources piece for what we want to offer for incentives versus how many people we can then support with our budget. So sample comes in in a bunch of different ways and has throughout my career. So I think it's really interesting to talk about. Love it.
Erin May [00:04:11]:
So it sounds like you have a breadth of different kinds of experience, and then you've got the sort of theoretical, what should our sample size be? Coming up against the realities of time and budget? And so finding those kind of real world compromises to get to your ideal sample size. All right, let's jump into it. When is the right time to start thinking about sample size? When you're planning your research as early.
Lauren Stern [00:04:34]:
As possible is the very glob response to that. The desired sample size has been part of my stakeholder onboarding for new research requests for a long time, and I find it really helpful to just have that conversation as soon as someone comes to me with a project that they want to do.
Carol Guest [00:04:48]:
And what does that conversation typically look like?
Erin May [00:04:50]:
Yeah.
Lauren Stern [00:04:51]:
So if it's a stakeholder that I already know, which most of the time it is, then what that conversation looks like is either they're using a form that we have. So at my last job at iRobot, we had a research request form that people could fill out. But quite honestly, most of the time that conversation is just me talking to someone and they're telling me like, hey, we kind of want to do this project, or we're trying to learn about this thing. And then I may in the background be pulling up a request form to fill out, but I'm asking them questions and we're kind of going back and forth about it. I found that forms tend to be a little bit intimidating for stakeholders. Like, they're not always sure if they're entering the right information or if they're putting, like, what if it changes? I don't want to tell you something and have it be wrong. And so as a result, if we just tell someone you have to fill out a form, we often don't get the request. So even if I'm using my own structured form in the background, I'm usually filling it out while I'm talking to them.
Lauren Stern [00:05:41]:
So that's a little aside. But how that conversation typically goes is I'll ask them what their research goals are. What are they trying to learn? What kinds of questions do they have? What questions go in the mod guide? It's what were you wondering? What do you want to know? What are we trying to answer with this? Do you have a hypothesis that we're trying to prove? Or is this more of just a, like, we want to get people's feedback on a topic kind of a thing? And then from there we go into what is the outcome of this research that you're looking for? So is this something where you need to make a decision and you're looking for something to support some sort of data to support that decision? Are you the one who makes that decision, or is this that you're trying to help someone above you, potentially or other people on the project make a decision and you're looking for data from me to help support that direction? Is this something where it's really early and explorative and you're just trying to figure out what your options are? And so kind of getting a sense really early from users is going to be valuable and whatever other things are, those are the examples that come to mind that we hear a lot, but there obviously can be other ones. So we talk through what the outcomes are and then we work backwards from there through the other aspects of the project. So if, for example, the research request is about getting feedback on a prototype, so we want to understand how people are using this new device in their homes or in their lives, then that tells me a lot of things about the follow up questions that I need to ask. I need to understand what access to that prototype I'm going to have. Is this something where it's fully functional, we can give it to people and they can take it home with them and we can see how it's working? Or is this something where we can fake it? If they come into the lab or into the office and we can try it out with them for a brief period of time, or does it not exist at all? And we're going to be doing a paper prototype or a figma that we need to walk them through, because how I run that study is going to change based on what resources are available, where we're at in the development cycle. And then additionally from there, we would be able to get into, okay, if we're going to use a real prototype, how many do you have? How many can I use versus if it's just a figma? And we're going to have people click through screens in an app, for example.
Lauren Stern [00:07:48]:
That doesn't impact my sample size at all. I can do that as many times as I want. We'd also potentially talk through budget. I've worked on teams where, as a researcher, I managed the budget for all of the research. I've also worked on teams where our stakeholders paid for research efforts. And so depending on who's paying for the study can impact how much budget you have. And then from there, we would go into what we would want participants to do in the study. And sometimes that's more me brainstorming a little bit live, and sometimes that's more stakeholders saying like, well, we really need them to exercise.
Lauren Stern [00:08:19]:
For example, we need to see them use this product in a bunch of different ways to understand if it's doing what we want. That's a very different ask for a participant than, hey, can you just look at some screens? And so how we structure that session is going to be different. Timeline is also something that we would talk about at this point in the conversation. When do you need this analysis by? Or when do you need this data by? That, again, is giving me information about how long I have to do the project and if it's a super intensive protocol and I need to run it one on one with participants, and I only have two weeks to do it. The amount of people that I can reasonably, as a researcher, get through that protocol and then analyze is going to be much lower as a starting point than if I were going to send a survey, for example, or if I had six weeks to do it. So there's a bunch of just logistical considerations, and I'm trying to hit as many of those as I can with my stakeholder. Other things that I could ask a stakeholder, but that, frankly, it's easier if I just know them or that I try to learn over time about the people that I work with. Are things like, is this person someone who responds better to quantitative information than qualitative? Is this the kind of decision that is very high stakes? And so if I come to them and say, we have a sample size of ten, here's what we heard, that's not going to feel like enough, even if it is technically, scientifically, it's not going to feel like enough.
Lauren Stern [00:09:40]:
So we can start to play that game a little bit. So having some of that context is helpful. And so sometimes I already know that context or I know the people well enough to have a sense of that, and sometimes I'll ask about it.
Carol Guest [00:09:50]:
It's a lot of information. It sounds like what you're saying is, I think there's a set of information that you might want to know in order to make the best decision or to help someone else make the best decision. And then there's a set of information that's maybe somewhat more related to constraints. So budget, timeline, what's realistic? Is that a fair way to characterize it, or do you think about it in another way?
Lauren Stern [00:10:11]:
No, I think that's a great summary. The one thing I would add to that is that there's also a piece that's trying to understand any of the background that might change my strategy for how I approach the project. So if we know, for example, this is something where quantitative data is going to be really important, I want to think about how to make sure I'm including that so that the work doesn't get ignored and is able to have the impact that we want.
Erin May [00:10:36]:
And it sounds like this whole sort of intake process, this planning process, is quite fluid and dynamic in terms of all these different pieces, could potentially move around a little bit, right? So, for example, the number of participants we're going to want in our research is, tell me if I'm wrong, highly related to the method we're going to use. We're not going to do 1000 user interviews, but we very well might survey 1000 people. And so as you kind of go through this process of asking questions and thinking about impact, time constraints, et cetera, do you find that you're often moving multiple pieces of the study design together, including the sample size?
Lauren Stern [00:11:15]:
Yes, absolutely. The other piece of this is if we know that we're, say, looking at, or we have some research questions about information that we ask almost every participant when we do research, like a warm up question, then that might be something where an individual sample for that kind of research, if it's user interviews, maybe we're only going to talk to ten people. But if I look back at the last six months where we've asked everybody if they have any pets in their house now, instead of having ten people in that sample, I can up my sample number for that piece of information a lot.
Carol Guest [00:11:50]:
I'd love to spend some more time on this topic of, I think, leaving aside sort of maybe the constraint side of things, I imagine there's a difference between the sample you need to you as a researcher, be confident in the patterns. Like this idea of convergence, a bunch of different people are saying similar things versus what you might need to convince a stakeholder. So how do you think about balancing those two things? I imagine there's a tension where some stakeholders might need a lot more than you, as a researcher, think is necessary.
Lauren Stern [00:12:20]:
Yes. I actually have had a recent stakeholder where this was like an ongoing conversation that we had almost every time we talked. I think that there's a couple of different ways that I've approached this. One is I've tried to just pull literature and try to make the case with stakeholders who, and to be fair, I work with a lot of scientists, and so they are people who respond well to published research. So being able to show them there is research that shows that there is a drop off when we're usability testing in the number of issues that we'll find after six or seven participants. So if we test with 20 people, because you like the number 20, that's not necessarily the best use of our time. Like, here, let me show you the journal articles. So that's one approach that I've taken.
Lauren Stern [00:13:06]:
The other is having them sit in on sessions. Honestly, when somebody is in the room and hears the same thing three times in a row, it's much easier for them. Or it's at least, I think, more palatable to say, like, okay, we got it. We've heard this. We can move on. The other thing that I've approached with some of those conversations is there is a research methodology. I guess that I really love called triangulation, where you use multiple studies to approach the same question that are all helping to give you the final answer. So we might do a small scale set of user interviews with ten people to get a good spread of demographics, but we want to understand a couple of key questions about this product.
Lauren Stern [00:13:48]:
And then we might also do a survey to 2000 people where we're asking, we take the learnings from our user interviews and we say, now we're going to ask structured questions that can go out to 2000 people to try and capture whether there's anything that we missed or whether there are any big themes that we wouldn't have seen at that small sample size, whether there's like a significant difference in responses based on gender or based on where they live in the country or something like that. And then we might take all of that information together and do a third follow up study, either with a slightly larger sample size where now we're going longitudinal and we're looking at similar questions, but over time and putting people into a diary study. Or we might do a follow up survey internationally because we're realizing that location is really important. That piece we can kind of decide as we go, but we're pulling in multiple data sources at different sample sizes to help make sure that we're covering all our bases, basically. Yeah.
Erin May [00:14:43]:
When you talk about triangulation and sort of multiple methods over a period of time, how much are you planning, your sample sizes and your methods upfront early on, versus. We're 50% confident it's going to look like this. And then we're going to revisit at these checkpoints and really figure it out as we go. I imagine it varies depending on the nature of the study. But how much are you trying to get that locked in kind of up front?
Lauren Stern [00:15:08]:
I'm trying to get it locked in up front as much as I can, because every time we have to change the sample size, it adds cost and it adds time. And so for most of my stakeholders, I tend to work in very fast paced environments. If I tell them the project is going to cost $1,000 and it's going to take us two weeks, and then we decide after week one that actually we need way more participants than we thought we did, now I have to go back to them and we have to negotiate raising the cost. We have to say, actually, this is going to take four weeks instead of two weeks. And in many cases that means that we miss the decision point. So my research can have more impact if I can plan as much as possible at the beginning. It's not always possible, but for the most part, and I think some of this comes with time and experience, too, you start to have sort of a running rolodex of how many people you really need to do different kinds of projects that you run a lot, and then you can start with that and build from there, whether that feels like enough or you need more or you need less based on what the specifics of that project are. So you're not starting from scratch every single time.
Erin May [00:16:19]:
Let's dig into some details. So you gave a bunch of kind of high level things you are thinking through and the if then statement of forms running through your head to figure out what these number of participants should be. Should we start with qual or quant? Where would you like to jump in?
Lauren Stern [00:16:34]:
Let's start with qual.
Erin May [00:16:35]:
Let's start with qual. Okay, so qualitative sample size is the subject of lots of sort of, I don't know, folklore, mystery. What should my number be? Is it always five? You mentioned stakeholders are saying five. What's the right number? Okay, so let's jump in. How do you figure how many participants you need for a qualitative study?
Lauren Stern [00:16:54]:
It's a great question. I think I'm going to push on it a little bit and say it's not just a qualitative study, it's what kind of qualitative study? I think if we're talking about usability testing specifically, there is a lot of literature on how many people you need for a usability test. So I feel pretty confident when we're working with stakeholders and trying to explain to them, like somewhere between five and seven, we're going to catch most of the issues, at least the big, really severe ones. That's probably enough. We also, in a lot of projects I really love, write methodology, which is where you have actually a smaller sample size. So you would only run like three sessions, and then you would look at what issues were found, make changes to the design, and then do additional sessions, alternating back and forth. Like three participants look at the design and iterate. Three participants look at the design and iterate.
Lauren Stern [00:17:43]:
And there's a whole tracking process that you do to make sure that if you don't fix something, you catch that and you're able to go back and deal with it. But that, again, has a lot of literature and research behind it to say that that's okay because you're building your sample over time, that that's an acceptable sort of approach. So for usability testing. I'm going to say that's generally easy. We have some soft rules, I'll call them to get us started for other kinds of qualitative research, things like just user interviews, exploration. If we're doing discovery research where it's super early, codesign sessions, diary studies, product testing, all those kinds of things, it gets way squishier. And what I find to be helpful is that I'll look at what type of user we're trying to talk to. So, for example, if we have a really targeted, we need to talk to our youngest user group, and we need to only talk to people who go to scheduled workout classes, then the type of person that I'm looking for is already pretty narrow.
Lauren Stern [00:18:46]:
So the group itself can be a little bit smaller. If we want to talk to anybody who takes a spin class, for example, and this isn't a real example because I haven't been at WHOOP long enough to run any research, but it's a good one. Then if we're talking to anybody who takes a workout class, that could be a huge range of ages, it could be a huge range of other demographics, race, gender, all those kinds of things where they live. And so the sample size that I would like to have to ensure that I'm catching people who represent a bunch of those different groups automatically needs to be a little bit bigger. And so that can give me like a starting point. And then from there I can look at those constraints. I can also look at whether or not I can only talk to one person at a time. Or is this something where maybe I can talk to multiple people, do sort of a group situation that makes it a little easier to have a bigger sample size so I can start playing with my methodology to accommodate that, too.
Erin May [00:19:41]:
So we've got our five to seven, or you mentioned the right studies where maybe it's three plus three plus three. You've got some notion for a usability test when we're going more discovery earlier on, we know it's probably going to be a bigger number, but how big is going to depend on a lot of other factors.
Lauren Stern [00:19:57]:
Yeah. And I think this is one of those places where within your organization, you'll start to find what's a sample size that feels comfortable to my stakeholders, that I know isn't going to get me a lot of gasps and horror that I only talk to six people kind of a thing. And also for you personally, for the types of timelines you work on, what is a reasonable number of participants for you to work with in that time frame and do analysis for. I think a lot of people, including me, have been on teams of one. And so you're trying to figure out sort of how much can I handle to get this project done. I find that for most product focused research, where I'm talking to people who already, either already own a product that I'm researching or I'm giving them some sort of prototype and having them test it out, that like ten to 15 is my sweet spot for what lets me hit multiple demographics and also be small enough that I can manage that sample effectively and get really in depth with them. For other kinds of projects where I'm doing, like, I need more diversity or I need to talk to people. For example, before I left iRobot, I was working on a project around product names.
Lauren Stern [00:21:02]:
And so we were doing word association and word meaning and then taking that into how people shop for products to see how, if at all, the names play into that. So there was a lot to those sessions. It was stuff that was about how we think and people think differently. So we automatically wanted a bigger sample and it was international, so we were trying to hit people across a lot of different countries. And so for that project, I needed a much larger sample, even though it was qual, just to ensure that I had a good representative group for each of those different factors. And so for that project, we pulled in other researchers, and I worked with contractors in other countries to help, and we did group sessions which allowed it to be a bigger sample size.
Erin May [00:21:42]:
Awkward interruption this episode of Awkward silences, like every episode of Awkward Silences, is brought to you by user interviews.
Carol Guest [00:21:50]:
We know that finding participants for research is hard. User interviews is the fastest way to recruit targeted, high quality participants for any kind of research. We're not a testing platform. Instead, we're fully focused on making sure you can get just in time insights for your product development, business strategy, marketing and more.
Erin May [00:22:07]:
Go to useernerviews.com awkward to get your first three participants free.
Carol Guest [00:22:14]:
You mentioned that within qualitative or within the non usability qualitative user interview diary studies, et cetera, that different methodologies might have different sample sizes. Are there any that stand out as particularly needing larger sample sizes? Smaller?
Lauren Stern [00:22:29]:
How do they differ? So for me, I would say that if I'm doing one on one interviews, that's probably in my slightly larger group in terms of qualitative studies, because we want to hear from a bunch of different people that represent different demographics. And you can't just talk to one or two people in a particular group and then be like, this represents this whole group. So I want to aim for, like, four, at least for any particular segment. And one on one interviews, especially if I can do them remotely, are not overly challenging to run. Like, they're usually low time intensity, easy to schedule, easy to find people who will participate. So my sample can be a little bit higher, and then depending on the number of groups I want represented, anywhere from, like, ten to 20. If I'm doing something like a diary study, where it's over time, there's a lot more operations, labor, essentially, that goes into that, because you're doing a lot of participant communication, you're doing a lot of follow ups with them, you're going through a lot of data points because people are sending you a lot of information over the course of that project. And so in those cases, I often want to scale down so that I'm not overwhelmed by data.
Lauren Stern [00:23:39]:
I recently did a diary study that had 40 participants, which was big, and that was because we were trying to hit three different marketing segments within our organization, and we needed them to be located all over the country. I came in on the second day after the project had kicked off, and I had 200 entries in the diary study, and we were on day two of like a six week project. It just gets really overwhelming. So in those cases, if it's appropriate, I'll scale back to, like, eight ish participants. I've gone higher, obviously, but that's usually my starting point.
Erin May [00:24:13]:
Do you factor in drop off? I know with diary studies or longitudinal, sometimes folks won't make it, you might start off overwhelmed with data and then end up, where'd everybody go? How much sort of drop off do you factor in for the different types of studies?
Lauren Stern [00:24:27]:
Great question for anything where it's scheduled one on one, I don't typically factor in for very much drop off because in my experience, if we're compensating at market rate and it's something people are excited about, especially if they're already a product owner, they want to give you feedback. And so typically we might have someone no show or cancel, but it's pretty low. And so if we're aiming for eight interviews and we get seven, I'm not overly concerned about it for the most part. So I maybe factor up a little bit, but not much. We also tend to find that we can reschedule people or pull in subs pretty easily. So if I'm aiming for, and that's easier with smaller sample sizes. So if I'm aiming for eight people and I have someone cancel. Finding one more person is a lot easier than trying to find ten more people because I lost 20 for diary studies, I do factor in for a higher drop off rate if it's a study where they're participating but they don't have any product.
Lauren Stern [00:25:21]:
If I've given someone a product, there is an expectation that they will complete that because they have to send the product back to us. And I have, especially pre COVID when we were doing everything in person, driven to people's houses to pick stuff up from them because they didn't return it. It happens. So I don't worry as much about it in those cases, just because they have to sign some pretty serious stuff that they'll give us back the prototypes. But if we don't have anything, if they can just drop off and disappear into the ether, then I'll plan for typically a 5% drop off. It also, again, comes down to compensation. In my experience, you have a much higher drop off rate if you're not compensating people particularly well, if you're compensating them highly and they're motivated to get that compensation, then they tend to want to complete the study.
Erin May [00:26:07]:
Makes sense. You talked a bit about one of the ways you think about the sample is the different methods. What's my stakeholder comfort going to be like? Are they going to feel like these results are credible for you? Coming from the academic side of things where it's like, no, these results are meaningful. From a qualitative, what are you looking for? Because I think this is less sort of understood maybe than quantitative.
Lauren Stern [00:26:30]:
Yeah, I think there's a couple of things. One is what I'm looking for in terms of overall confidence, a, it's very different than when we talk about quantitative work. And I think this is something that I've had to talk to stakeholders about a lot because especially for people who do have an academic background, there's this kind of implicit association between saying we have high confidence in something and saying something is statistically significant. And when we're talking about qualitative work, we're not calculating statistical significance. That's not what this is. And so I've kind of, in many cases, had to have that conversation with stakeholders and say, hey, we're not looking to extrapolate out to a mass population, we're not calculating a p value, we're not looking at statistical significance, which means that how we calculate confidence is very different and we'll kind of walk through that. The other thing I've actually had happen is I've had stakeholders say, okay, well, if you're only talking to six people, then you're not looking at any sort of generalizations. Thus, I don't want you to do analysis.
Lauren Stern [00:27:30]:
I just want you to give me the raw data on every single one of those people. And we're going to go take every single one of their feedback individually because every person matters, because it's not generalizable at that small sample size, which also doesn't work for a number of reasons, the main one being we're not generalizing. But you do see themes at small samples. If you're going really deep and you're doing effective activities in your research to get at what you're trying to learn, you will start to see themes. You will. As we affinitize our data, like things do bubble to the surface. If they don't, that's a really good reason to increase your sample size. But often that they do, and so you do want to focus on that also, we have limited resources.
Lauren Stern [00:28:10]:
We can't do everything, so we have to prioritize. And if you just take everyone as exactly the same, you can't prioritize any feedback. So often what I've done in that case is go through with stakeholders and almost have the opposite conversation where we talk about starting to see themes, starting to see commonalities between people, identifying with them often, or sometimes I'll bring it to them. Where people are unique, where our participants are unique from one another. This one has pets, this one doesn't. This one has kids, this one doesn't. So when they're giving feedback about those specific aspects of their lives, yeah, maybe we do want to place a little more emphasis on that because we only have one person to represent that. On the other side, everyone in the study lives in a single family home.
Lauren Stern [00:28:53]:
So if they give us a piece of feedback about living in a single family home, we're going to look at themes there. We're not going to just look at one person because of the sample size. So I've had to approach it in both directions. But I think being able to talk about, even if you're not doing like a mathematical calculation of confidence, being able to provide something to say from the research we've done in the past, I've worked here for four years and we always run samples like this and we tend to see x, y and z outcomes. Or looking at the academic literature, this is how sample size is calculated. And I'm following best practices, being able to point to something to show why you got to the sample size you got to is really valuable with stakeholders who are unsure.
Carol Guest [00:29:34]:
I'd love to sort of jump in the room with you on some of these stakeholder conversations. Do you have specific language that you use to differentiate confidence in sort of a predictive sense, like the statistical significance versus trends you tend to see in studies? I wonder if there's a way that you differentiate for people who are particularly caught up on the statistical significance element.
Lauren Stern [00:29:56]:
Yeah. So I'll be totally honest. For people who get really caught up on statistical significance, if we're talking about qualitative work, I try to just push them as far away from it as possible because I think it's distracting. That already is a red flag to me that they're going to look at my analysis and the outcomes that we're recommending from this research with a lens that I don't find productive because they're looking at it as a numbers game. And this is not a numbers game. This is about empathy at its core. So I'll try to just pull. We'll dial back that as much as possible with people where they're not necessarily saying, like, oh, statistical significance, but they just want to understand, is this enough people? Is this, like, I have to go make a big decision based on your recommendations.
Lauren Stern [00:30:38]:
We're changing the design. I just want to know that you're supporting me, that this is actually helpful in those cases. I have occasionally used a confidence calculator. I think Qualtrics has one. I've used theirs for quant research, a whole bunch. There's also a formula for excel that you can get as, like a macro. So I've used things like that to give them some level of confidence. Those cases, though, often don't get to that level, because at those moments, I can say, okay, last year, we made a decision to add a button to the app.
Lauren Stern [00:31:09]:
Like, we changed the button in the app, and when we did that, we'd usability tested it with 25 people, and it had a really good response after we launched. So we're doing a sample size of 20. I think we can feel confident about that. And usually just being able to give a specific example that the stakeholder is familiar with is more powerful than trying to show them numbers.
Erin May [00:31:29]:
All right, should we talk about Quant?
Lauren Stern [00:31:30]:
Sure, let's do it.
Erin May [00:31:32]:
Okay, so, Quant. I'm thinking about numbers, larger numbers. Is there a number that makes something quant?
Lauren Stern [00:31:39]:
Great question. I've thought a lot about this. To me, there are two things that make a study quantitative. One can be a large sample size. If I am sending a survey to 2000 people, for example, the way that I build that survey is very different than a survey that I'm sending to 100 people, for example, I may have a lot fewer open ended text questions because I am not going to sit there and read 2000 responses. For the most part, if I'm only sending a survey to 100 people, I can ask them why on every single question, and maybe I shouldn't for other reasons, like how long it'll take them to fill it out. But I could read all those responses. So how I'm structuring the survey becomes very different.
Lauren Stern [00:32:20]:
Thus it is less about going deep and it is more about going broad. So at any point that we're talking about going broad and looking at trends and looking at kind of a general population, to me that's quant research. So that's one piece of that is sample size. The other piece of that can be that we're doing some sort of hypothesis testing. We're looking for relationships in our data, which means that we're going to be doing some sort of statistical analysis. And anytime we get into using math as part of a project, to me that now we're in quant, can you.
Erin May [00:32:51]:
Have a survey that is both qual and quant at the same time and analyzing different parts of it in different ways, or you pretty much know up front, like the purpose of this particular survey or this particular method, maybe even within a larger triangulated study, is a quant versus a qual.
Lauren Stern [00:33:09]:
That's a really good question. I think that from an analysis method perspective, absolutely. I mix qual and quant from what is the goal of the research perspective? For me, it's usually firmly one or the other. So if the questions I'm trying to answer are looking at, we're going broad, not deep, we're looking at broad population trends. Even if I'm doing some qual analysis and doing open response questions and analyzing those in a qualitative manner, it's still generally a quant study. And the qual research is adding additional depth to my analysis and the types of insights that I'm able to offer my stakeholders at the end. Whereas if my focus is going really deep, is on building empathy, is on really digging in on understanding humans at a granular level, that's a qual study. Even if I'm at a large sample size, even if I'm using multiple choice questions or other sort of more quantitative.
Erin May [00:34:04]:
Methods, when I think of quant, I think of surveys. What other methods are in the mix. What else should I be thinking about?
Lauren Stern [00:34:11]:
So, my background before I got into UXR is in psychology, and I did a lot of behavioral studies which look like a survey. If you're a participant, like you're answering some questions in a survey format. But on the back end, that's very different research than a traditional survey, because what we're looking at is decisions that you made throughout the courses at research. And this is something I've actually done as a UXR, if we're trying to get a sense of. So, one of my favorite projects I've ever run was looking at robot personality and whether or not people assign a personality to their robot, and what, if any, impact that has on other things that they think about their robot. So, for example, if you name your robot, are you more likely to be satisfied with it? Are you more likely to think that it does a good job at cleaning your home? And so that was a behavioral project where we asked some questions in a survey format, and then what we were looking at was any statistically significant relationships between the attitudinal data that you provided and a binary. Did you name your robot or not? Or behaviors that you reported? So I guess this is a way to say, maybe they're all surveys, but what a survey actually means in terms of the study design and analysis is many different things. They're not all surveys, but for a participant, you're just answering questions on a screen.
Lauren Stern [00:35:36]:
You can also get into quant studies that are in person or in other methods where you're doing interviews. But usually that requires either a lot of people to do the interviews or building in other methods to kind of get you to the point where you have enough data.
Erin May [00:35:52]:
Does naming your robot make you like them more?
Lauren Stern [00:35:55]:
So naming itself doesn't. But we did see trends between if you anthropomorphize your robot, if you felt that your robot kind of had a personality and you had assigned that sort of relationship to it, you were more likely to think that your robot did a good job.
Erin May [00:36:08]:
Just feels relevant to the world we're all living into. All right, cool. Okay, so how many participants do you.
Lauren Stern [00:36:15]:
Need for your survey? It depends which is everyone's favorite answer. So this is where we get into, like, Qualtrics has a really good calculator for this. It depends on the types of analysis you're going to do. If you're going to look at descriptive statistics, meaning you're going to look at averages, you're going to look at how many people said something that requires a lower sample size than if you want to do hypothesis testing, if you want to look at relationships, if you want to do clustering. So anything where you're doing hypothesis testing. In psychology, I was taught as a general rule that you should never do statistical analysis if you have less than 100 people in a group. Meaning if you want to look at the relationship between two groups, you need at least 100 people in both of those groups. So we're talking about a sample size of hundreds to get to a point where you can run like an ANOVA or a t test.
Lauren Stern [00:37:03]:
If we're talking about something like clustering, where you're not sure what you're looking for, you're just going to send it out and then look at the trends. When it comes back, you need an even higher sample. So I would start at 1000 for that, for example. But for getting into the specifics of like, is getting 150 enough, or do I really need 500? That's where I would lean on a calculator, because I don't know that off the top of my head.
Erin May [00:37:29]:
Something. Tip of the tongue, Carol, trying to think about.
Carol Guest [00:37:33]:
Yeah, I guess I was thinking of examples of examples of research that fall in between these two, not quite qual. And some examples that come to mind are, I think, mixed method studies, which we've talked about a little bit, triangulation, which you mentioned. So for something that falls in between, where you might want to apply a quantitative lens, but it's mostly qualitative. So a user interview where you're asking someone to rate something on a scale, and maybe you ask multiple people to rate things on a scale, how do.
Lauren Stern [00:38:04]:
You think about sample size there? That's a great question. I've approached this in two ways. One is I like rating questions, scale questions, as a conversation starter in qualitative sessions, especially in interviews, because sometimes it's hard for people to articulate how they're feeling about something, especially if we're asking them to break down an experience that they just had, like do this task. Now tell me all about the task that's really hard for some people. And so giving them something to latch onto is valuable, and so we have them rate it and then we ask why, and we have them explain. And that gives them that little ladder up in those cases, for the most part, I just don't show the numbers. Like, the point of that question is not to get a sample. That lets me tell you something about how many people found this difficult.
Lauren Stern [00:38:49]:
What I'm trying to do is get a deeper insight into their experience. And so I may say most participants found this challenging as part of my readout, but I'm not breaking that down into like, six people said that this was very difficult, and three people said that it was somewhat difficult. One person said it was very easy. It's not important to the conversation. So in that framework, I just don't provide numbers. There are other times where we're asking that question because we need a little bit of oomph to explain. I sometimes call that the your baby is ugly conversation. If we have, say, a very challenging design that we're trying to convince a stakeholder, like we really need to change, having that little bit of quantitative information can be really valuable to just drive the point home.
Lauren Stern [00:39:33]:
And so in those cases, I will typically aim for a larger sample size instead of six. Maybe we go for ten, for example. And then also I may or may not show the numbers. So then we get into the realm of like, I'm going to show you a chart and I'm going to show you the proportion of our participants and who said that this was easy versus difficult. But I'm not going to say seven people said that this was easy and six people said that this was difficult or whatever the case may be. I may show percentages and just not break it down to individual counts. It becomes a little bit of a judgment call based on the story that I'm trying to tell and what feels truthful to the data, while balancing how my stakeholders are going to react to the information that I'm giving them.
Carol Guest [00:40:14]:
So if you're wanting to do some descriptive statistics, as you described it, of total or average number of people who rated this a certain way, then you're probably not going to get it from a small sample in a user interview, maybe a better fit for a survey. Whereas that user interview question you're saying is essentially, it looks like Quant on the surface, but really it's driving a qualitative goal, which is to better understand the user.
Lauren Stern [00:40:38]:
At that point, I would say yes. I think the one thing is we may want to frame it as quantitative if it's helpful for the conversation with stakeholders. Like depending on what we're doing with that data, some people respond better to numbers. And telling them that 60% of participants rated this task as very difficult is going to be meaningful in a way that no amount of user quotes or anything else is going to make the same point. So there's some amount of judgment call in there. But my way of approaching those kinds of ratings questions in an interview is very different than if I were asking a similar question at a sample size of 200, for example.
Erin May [00:41:14]:
Yeah, you talked about this a little bit before. And I think one of the themes that's been really interesting to me in this conversation is how much making an impact with stakeholders really factors in here to how you think about sample size and how you balance the truth of the research with the story you need to tell. Right. And what's the best way to position this? Not to make up a story, but to tell a cohesive story backed up by the objectivity of the data. Very interesting. So if I am talking about, I talk to ten people, a small sample. This is qualitative research. How often are you talking about percents of users who had a feeling you're doing that to show a theme?
Lauren Stern [00:42:00]:
Honestly, I often don't even frame it in percent. In that case, sometimes it's helpful. I find as like a kind of grounding conversation at the beginning of a readout, for example, to say, okay, as part of this study, we spoke to ten people. Of those, 8% like to work out outside and 88% like to work out in a class. And I am very bad at math off the top of my head. So whatever percentage is left, like, to work out in the gym alone, I might do something like that and then I can break it down if there's something specific that we want to get at. Like 50% were already product users and 50% were new to the product, like those kinds of things. Because I think that can be helpful for people to sort of picture where the feedback is going.
Lauren Stern [00:42:48]:
But I'll tend to stay away from percents when I'm talking about the themes because again, I'm trying to build empathy. And the numbers, to me, take away from the humanity.
Erin May [00:42:56]:
All right, what did we not cover? What are some just parting words of wisdom in terms of sample sizes, sampling, getting it right?
Lauren Stern [00:43:05]:
I think that something I've seen a lot with more junior researchers on my teams has been being a little bit afraid to have a conversation around sample size. It feels like something that either you're supposed to know as a researcher, you just should magically know what sample size you need, or that if you admit that you don't know that, you're going to get a lot of pushback from stakeholders and then that becomes a whole other headache you have to solve. And so we just avoid the conversation altogether. And I wish that we talked more about sample size. That was why I was so excited to come on and do this. I think for researchers, it's really helpful to have the experience and the backing to know what resources are available to you to figure out the sample size, whether that's your personal experience, the experience of your team as a whole, the literature calculators. There's lots of stuff out there. And at the same time, having these conversations can help then build the confidence to be able to go to a stakeholder and say, hey, I think the sample size that we need is x, but we have a little wiggle room.
Lauren Stern [00:44:04]:
Does that number feel okay to you? Do you need it to be a lot higher or lower? And engage in that in a way that's not going to spiral?
Erin May [00:44:11]:
All right, lightning rounds. Favorite interview question question to ask participants, not research question.
Lauren Stern [00:44:18]:
Sure. If you could wave a magic wand, what's something you'd change about? And then whatever the topic is, it's a classic. But I love it. It's a good one.
Carol Guest [00:44:25]:
What are two to three resources you recommend most to others?
Lauren Stern [00:44:29]:
I love the People nerds blog. I also will recommend the Reops community, which has a medium and also a slack channel. And then I think the other big one is Google Scholar. Like, go look at what's being published. We often don't because it feels, I think, very stuffy. But there's some really good stuff out there, and it's interesting to see what people are doing in an academic space.
Erin May [00:44:50]:
And where can folks follow you and meet you in person or however you like to engage with folks?
Lauren Stern [00:44:55]:
I'm on LinkedIn, but I will say I'm very bad at responding to LinkedIn messages. The easiest way to find me is to run into me in person. If you are in the Boston area and you want to come to Wooblabs and participate in research, we'd love to have you. I'm also going to be on a panel about ethnography at a learner's event in May that's happening at toast in Boston.
Erin May [00:45:15]:
Oh, exciting. Maybe we'll get Carol there. We just recorded an episode on going to events and how to navigate events, so that could be a fun one.
Lauren Stern [00:45:23]:
That would be awesome.
Carol Guest [00:45:24]:
Sounds great.
Erin May [00:45:25]:
Awesome. Well, thank you so much, Lauren. It's been very educational for me and I'm sure lots of our listeners.
Lauren Stern [00:45:30]:
Thanks for having me. It was really fun to get to nerd out about sample size. I appreciate the opportunity.
Erin May [00:45:35]:
Awesome. Thanks for listening to awkward silences brought to you by user interviews theme music by fragile gangs Listener. Thanks for listening. If you like what you heard, we always appreciate a rating or review on your podcast app of choice.
Carol Guest [00:46:07]:
We'd also love to hear from you with feedback, guest topics, or ideas so that we can improve your podcast listening experience. We're running a quick survey so you can share your thoughts on what you like about the show, which episodes you like best, which subjects you'd like to hear more about, which stuff you're sick of, and more just about you. The fans have kept us on the air for the past five years.
Erin May [00:46:26]:
We know surveys usually suck. See episode 21 with Erica hall for more on that. But this one's quick and useful, we promise. Thanks for helping us make this the best podcast it can be. You can find the survey link in the episode description of any episode, or head on over to userinterviews.com. Awkwardsurvey.