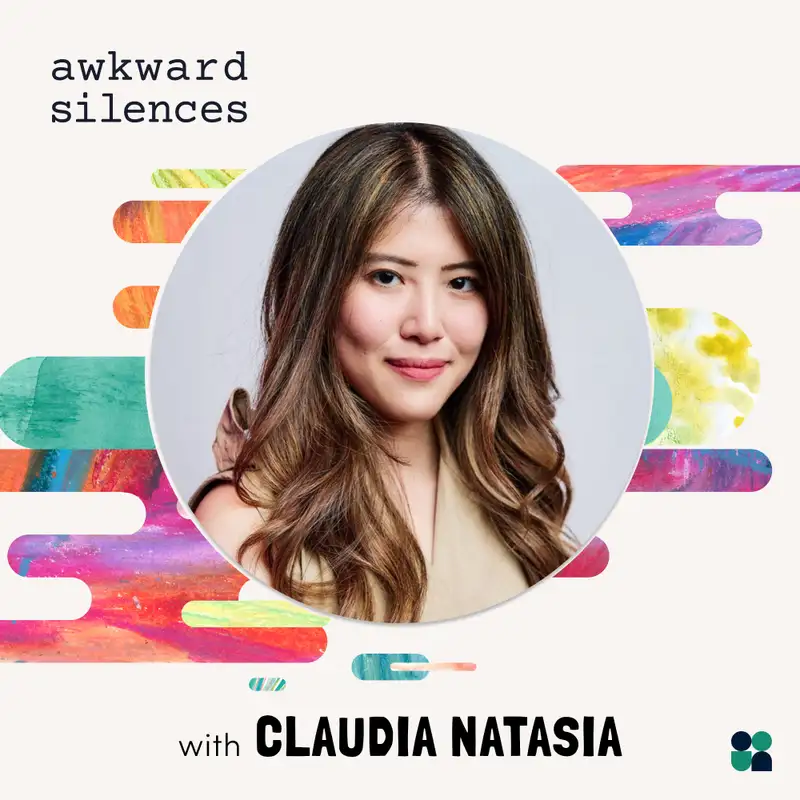
#148 - Connecting Research to Revenue with Claudia Natasia of Riley AI
Claudia Natasia [00:00:00]:
Don't be afraid to triangulate data. There's so many ways that you can do it. There's so many data sources that you have. The companies that are successful are the ones that are comfortable triangulating data and doing the hard work, because that's how you find the insights that other people can't find. If you're just relying on user research or if you're just relying on product analytics, most of your competitors are probably doing the same thing. So they're uncovering the same stuff that you are, they're talking to the same users and uncovering the same things that you are. But if you find a way to combine all of these insights together, distill meaningful signals, and come up with strategies that way, that's where you can actually differentiate and find what's meaningful that other competitors might have missed.
Erin May [00:00:41]:
Hey, this is Erin May.
Carol Guest [00:00:42]:
And this is Carol guest.
Erin May [00:00:44]:
And this is awkward silences.
Carol Guest [00:00:48]:
Awkward silences is brought to you by.
Erin May [00:00:50]:
User interviews, the fastest way to recruit targeted, high quality participants for any kind of research. Hello, everybody, and welcome back to awkward silences. Today we're here with Claudia Natasha, an expert on research, and has been thinking a ton about how to show the impact of research on your business. But today we're going to focus about very specifically, the impact of research on revenue, which is something obviously many, if not all businesses are trying to drive towards. So I think this will be a really useful and impactful episode for everyone listening. So thank you for joining us, Claudia.
Claudia Natasia [00:01:33]:
Of course. Excited to be here.
Erin May [00:01:35]:
Awesome. We got Carol here, too. Hey, everyone.
Carol Guest [00:01:37]:
Excited to dive into this topic that many of us are talking about these days.
Claudia Natasia [00:01:41]:
Right?
Erin May [00:01:41]:
So, Claudia, I know you've just closed around. You're the co founder and CEO at Riley AI, your new venture. So excited for that. Thinking a lot about revenue and in all aspects of your work life. Let's start from a high level and talk about researchers are all thinking about how to show the impact of the work of their research. When you think about research, often it can be a couple levels removed from revenue. So how do you start to just think qualitatively about making those connections?
Claudia Natasia [00:02:13]:
I agree with that statement that research tends to be a couple of steps removed from revenue. But at the end of the day, it's really helpful to always ground yourself in the understanding that you are part of the business. So very, so often I notice that research teams will isolate themselves, and it's usually it happens inadvertently because research teams tend to be under many layers of an organization under design, which is under product or sometimes under design, which is under engineering, which is under a larger organization. And so from a qualitative, high level perspective, the thing that researchers need to feel comfortable doing from the beginning is recognize that even if you are a smaller organization that's under different layers, that you are still, in fact, part of the business. And so because you're part of the business, you have to have the same amount of desire of gusto to learn about the OKRs that are driving the business. Not the product OKRs, but the high level of business OKRs that's driving your business's next raise for series B or if you're a public company, your revenue goal for this coming year. So making sure that at the beginning of each fiscal year as a research team, you understand those deeply, will set you up for success for everything else that you do, all of the planning that you do, all of the studies, all of the projects that you do in the coming year.
Erin May [00:03:28]:
Amazing. Yeah. So just having a kind of qualitative sense of how does the work we do fit into the revenue model, the growth model of the business, whether it be OKRs or KPI's, an operating model, you want to figure out how what you're doing is plugging into that revenue model.
Claudia Natasia [00:03:43]:
Exactly. Great.
Erin May [00:03:44]:
And then when it goes to kind of, you know, making the case for, you know, a project by project level or an annual plan, how do you think about tying that individual research work to that revenue model?
Claudia Natasia [00:03:57]:
It really depends on the types of revenue goals that you're trying to achieve. So let me talk about two in particular. That's very common. If you are a newer startup, or even if you're in an older company or a more mature company and you are working on a relatively newer product, then your goal would be to acquire as much customers. And so your goal would essentially be to drive new ARR, new revenue from acquiring customers. And knowing that goal means that you have to figure out research studies, discovery, let's consider it maybe like discovery research studies to figure out how to get newer Personas to try your product. So in that sense, as I'm discussing this for a lot of you're already probably thinking about, huh, then I'd want to do maybe Persona discovery. I'd want to talk to as many users as possible, see if I could segment these users into particular groups that are more likely to use the product and see what they would love about this product experience.
Claudia Natasia [00:04:56]:
So knowing that what's needed right now for this newer company or this newer product is acquisition, allows you to frame your study in a way that's most impactful for that. Now for let's say a more mature company, or let's say it's a product that you've launched and you're trying to get people to come back more because for some reason, after the first 90 days of using it, they don't come back. That's a separate problem. At that point, you'd know that you'd want to focus maybe on issues of retention. Why are people not returning? A lot of you might start with usability studies to just rule out if there are problems on the experience that definitely needs fixing. Or you might do some discovery interviews, focusing the questions more. So on what are you currently doing to fulfill the pain point right now that you thought that that product would fulfill, but these users are in fact not trying to fulfill or fix that pain point on that product. So it really depends on the lifecycle of the business and the goals that you want to achieve.
Carol Guest [00:05:53]:
When you think about the lifecycle of different businesses, I imagine you mentioned acquisition with a new company and retention. I imagine modernization is a piece of it as well. Are there a few different sort of broad categories that you think are most common that a researcher can align themselves to?
Claudia Natasia [00:06:07]:
Yeah, so I like to personally align. I've worked in companies from seed stage to public, and I like to personally align myself with the stage of fundraising for these companies. And stage of fundraising is pretty telling. So if you are a seed to series B company, and I know with AI tools, we can talk a little bit about that. Now, fundraising for AI is really crazy, so it makes the lines a little bit more blur. But for Seed and Series A, Series B, you are right now at more of the exploratory, trying to get as many customers trying to understand your market. So you'd want to focus more of your studies on acquisition and discovering how to drive stronger acquisition if you're a more mature company, and usually if your company already has 50 million AR or above that, you tend to be more in the growth phase. And so in the growth phase you'd want to focus more on retention, lifetime value driving, ensuring that there is no churn, ensuring that you can retain your users while also understanding newer markets or newer types of users that you can expand to beyond your core.
Claudia Natasia [00:07:14]:
So I would say it gets a little bit more complicated at later stages, but that's a nice, easy way to parse out the types of studies and the types of goals that you'd care about.
Erin May [00:07:23]:
Awesome. Awesome. Claudia. Yeah. I think because research is going to look so different in every company, it's a fundamentally difficult question. But what we're getting is the start of a framework, which is the first thing I hear you saying is you really want to be thinking about the impact of your research on revenue in the planning stage, when you're planning the research you're going to do, versus trying to retrofit after you've done the research. Oh, gee, how did this impact revenue? Right. That's going to be the first thing to maybe set you up for success.
Erin May [00:07:52]:
And the second is that a good kind of heuristic might be to think about what stage is my company in terms of fundraising, in terms of revenue, and how might that map to a focus on acquisition or retention? And then, of course, you want to layer that with what you know about the strategic priorities of the business. Right. Is it more acquisition or retention? Just kind of depending on what's going on in the business itself?
Claudia Natasia [00:08:16]:
Yes, exactly. Great.
Erin May [00:08:18]:
Great. You mentioned AI is kind of a passing comment, but is it worth taking a brief detour into AI, given that you're running an AI business and it's so top of mind for everyone right now?
Claudia Natasia [00:08:28]:
Yeah, yeah. Of course. As some of you might be familiar, just to talk about Riley AI very quickly, I started Riley AI a few months ago, and it's something that I've wanted to do for a while now. The idea actually came up when I was having a call with my research team two jobs ago, and I was in line for a cheesecake factory down the street, and everyone was arguing in the call, and my husband was there. And I remember telling my husband, everyone's arguing about our business goal right now. We need to drive LTV as a business. And there's so many different insights we can use. The data science team analyze some product analytics metrics.
Claudia Natasia [00:09:05]:
The research team analyzed and did some topic modeling of user research studies. 80 different interviews, and they all have different ideas for how to drive LTV, and we can't seem to come into an agreement, and it's been a month. In a month. You could already build a solution at this point. And so I thought one researcher, actually, his name, spec Millay, I like to mention him because he combined all of the insights together into one model, which ultimately generated a strategy and a direction. And I thought, if there's a way we can bring this to everyone, where instead of spending hours trying to wrangle disparate sources of data, trying to build your own topic model, if there's a way that we can automate all of this data wrangling and recommend strategies that will significantly help ensure that all teams are aligned and can move towards the same direction. And so that's what Riley AI does. Essentially, we combine all of your disparate sources of data.
Claudia Natasia [00:10:00]:
We produce strategy recommendations that learn and grow with you over time. And I really think I always get this question in different conferences or podcasts. Will AI replace researchers? Absolutely not. At the end of the day, as researchers, product managers, we're all still responsible for collecting the data, for understanding our users deeply. What AI will do is help be our second brain. Almost like I drive a Tesla, so almost like the map or the auto drive in the Tesla that tells me, or that there might be a car coming, it helps forecast better, and I'm really excited for the potential of AI to just make us get better at forecasting.
Erin May [00:10:36]:
Fantastic. Fantastic. So that's actually a perfect segue into the thing I wanted to talk about next, which is to talk about sources of data that you might use to tell that story about the impact of research on revenue. So I know you're a big fan, like a lot of researchers on triangulation, but maybe we could break apart some of these different kinds of data that you might use in your toolkit to talk about research and revenue.
Claudia Natasia [00:10:59]:
Yeah. So there are a couple of data sources that I've personally found very valuable in the past. The first I'd like to talk about is actually clusterings of user behavior. And the reason why I call it clustering of user behavior is we as researchers do so many different user research interviews. Right? Like there could be typical thing is like, let's say you do 20 interviews for a product, then you do 20 other interviews for a different product out of all of the interviews that you do in a year, what my research teams and PM's have done in the past is try to find commonalities in behavior across all of the interviews. So even if the interviews are done in isolation, if we look back at the end of every quarter and see all of the different interviews, we can usually see clusters of behavior still form. And there's data science methods to do that. So running a topic model, for example, to see the different cultures of behavior, and a good example of this is actually, we discovered in two companies ago that sales reps actually love being coached live.
Claudia Natasia [00:12:05]:
When they do a sales call, they like getting little tips on a chat bar on the side, or they were really trying to envision what this future would look like. But essentially, if you're on a sales call, you want your manager to be able to coach you live as you do your pitch and say that, hey, Claudia, maybe your voice needs to be a bit louder or use this content right now. And we discovered that through this movement in behavior from all of the interviews that we did, and that ultimately led to the creation of a sales coaching product, which was really powerful for that company. So clustering of behavior is really important as a data source, and that comes from user interviews. One other data source that's really important also is competitive arbitrage. So that comes from essentially competitive analysis, but with intention. And the intention isn't just to see what your competitors are doing, because of all you do is copy what your competitors are doing, you will only get a small percentage of the market share that already exists. It's really seeing like having the contrarian thinking to see, okay, all competitors are moving towards this direction, but what exactly is the pain point that they're trying to solve, and are they solving it correctly? And usually what my teams discovered is all of the competitors are moving towards this direction, but they're not necessarily.
Claudia Natasia [00:13:18]:
So this is the pain point. Maybe there's a different way to solve the pain point, and we solve the pain point using the different way, and that's where we usually land with gold.
Carol Guest [00:13:28]:
Going to this clusters of user behavior. One, I'm just thinking about, one, what it means, and two, how one might apply it. Maybe if they don't have a full data science team. So in terms of what it means, are you describing that there might be. You have a whole lot of user feedback, and you might be grouping that user feedback by theme? Or is there something else going on, too, where you're also saying users like this tend to have this type of feedback? Can you say more about that?
Claudia Natasia [00:13:51]:
Yeah, of course. It's actually the former, Carol. So it's grouping feedback based on certain themes, and there's two ways to do it, the most common ways to do it qualitatively, which I know a lot of us probably have done one way or another. That's the ideation method of trying to cluster different feedback into one larger theme. Now, there's actually quite a bit of. There's a paper that maybe I'll forward to everyone here at a separate time, but there's a paper that shows that that's extremely inaccurate and not replicable. It is good for ideation and good for discussion, but if you rely on that fully, it shouldn't be the full solution to everything. And so a good way to do it from the data science perspective is actually to create a topic model.
Claudia Natasia [00:14:33]:
And an example of a topic model is k means cluster model, where you're clustering all of these texts that are quite similar to each other. And it follows trigonometry theory of euclidean distance. Sorry, I'm just throwing a bunch of terms, but we can dig deep on this a little bit later. I get really excited when I talk about data science, everyone. But essentially what we're trying to do is find the distance between each text that are closest or most similar to each other. And if the texts are very close and similar to each other, then we cluster them into one topic. So it's what we are doing with our whiteboarding, with our. With post it notes, but in a more tech specific data science method.
Claudia Natasia [00:15:15]:
Got it.
Carol Guest [00:15:15]:
So it's sort of like we do affinity mapping. We look at a bunch of insights from maybe a small set of interviews, and we do some lightweight affinity mapping. That might be the low tech version.
Claudia Natasia [00:15:23]:
Exactly.
Carol Guest [00:15:24]:
There's a data science version, this topic model, where you're looking at similarity among terms.
Claudia Natasia [00:15:28]:
Exactly.
Carol Guest [00:15:29]:
And part of what you were saying was, you can now scale this to.
Claudia Natasia [00:15:32]:
A lot more research.
Carol Guest [00:15:33]:
Is that a big.
Claudia Natasia [00:15:34]:
Exactly. And that learns and grows with your terminology also. So that's why I'm personally passionate about building what we build in Riley AI, because I remembered at five stars, we use user interviews a lot. We have all of these amazing notes and insights. And my dream back then was to have a way to analyze this insight at scale without necessarily having to code my topic model manually. And combining all of these texts in a way that fits the language of five stars. Because there are certain terminologies that are more common for a company relative to another. Right.
Claudia Natasia [00:16:07]:
Like, we all have our own nomenclature, our own colloquial language of talking about our products within the company.
Erin May [00:16:14]:
Amazing. And part of what I hear you saying with some of the triangulation and looking at the movement, the sales example is great. Cause it's easy to imagine how finding a lift in your sales related software will have an impact on revenue for other organizations. Right. But you're talking really about, like, skating to where the puck is going. Is that the sports metaphor? Right. Of it's not what is happening, but how are things changing? How are things moving? And how can we use those insights to drive our revenue driving innovation? Is that a fair way of summarizing?
Claudia Natasia [00:16:43]:
Yes, absolutely.
Erin May [00:16:45]:
Awesome. Awesome. So these are some of the data sources that we can use to really run impactful research. Are there other sources that you use in terms of measuring the impact of research? Or thinking about how to measure and share that.
Claudia Natasia [00:16:58]:
Yeah, very early on, I've always really relied on integrating research, product analytics, and I was quite fortunate to have product analytics part of my organization in a few jobs. This makes it significantly easier because one of the feedback that I received from a lot of people whenever I do talks is it's really hard to get my product analytics team engaged. One person actually, a few weeks ago told me that they've tried slacking a product analytics team member and got ignored. And so we can talk a little bit about tips on how we can get product analytics team engaged after this. Back to the question. Having knowledge of product analytics earlier on is extremely helpful. And there's this one arm of product analytics called telemetry that actually tracks conversion and user behavior across a platform, which is something that I believe every researcher should know and the reason why it's basically like a live usability study. You're able to track from the moment Aaron enters a website, what Aaron does after, and then what causes Aaron to drop off, what causes Aaron to stay, and that adds additional insight to your user research studies.
Claudia Natasia [00:18:03]:
And so I rely deeply on telemetry. I also rely deeply on North Star metrics that ultimately drives research. So something like lifetime value LTB, or let's say monthly active user time it takes for users to complete the first task of a particular product, like checking out of a ecommerce site. Those are important metrics that I ground every single research practice and every single product on.
Erin May [00:18:28]:
Right. And that's a great tie back to what you were talking about earlier, where often you have research teams embedded in product teams. Maybe that's embedded in an engineering team. And now revenue is over here and I'm over here. But hopefully the product team has done some good thinking about how do we map our work to revenue. And the North Star metric might be one way that plays out so you can kind of orient toward that, knowing that that's going to impact revenue, right?
Claudia Natasia [00:18:52]:
Absolutely.
Erin May [00:18:54]:
Awesome. Any other tools or types of analytics you like to use in your research.
Claudia Natasia [00:18:58]:
And revenue work tools in particular? One thing that I absolutely, really, really love is actually amplitude. And the reason why using amplitude is very good at scale is because, especially if you're not a data scientist, or you're a PM that's extremely busy with other things, or you're a researcher that's extremely busy with other things, you want a tool that helps you get what you need very quickly. And I think amplitude is a good, easy step to get there. And then if you want to do more of the complicated stuff like run your own models, then you can always download the data, but at least the first few makes it extremely easy for you to do what you want. Yeah, and beyond that, I would encourage everyone here if you do want to explore data science methods a little bit to download rstudio. Everyone always feels like if they want to do data science, they have to download python and attend boot camps and everything. That's such a high barrier to entry. If it takes you two months to learn, you'll probably lose interest in the first few weeks because we're all busy with other things as well.
Claudia Natasia [00:19:56]:
R makes it extremely easy. You can download dummy data and start playing with it. It's very guided as well. There's so many libraries and blogs that you can read to help you understand how to run your first model. At the end of the day, even if you do use tools to help you run the analysis. For example, Riley AI, you'd want to be able to understand what goes in the analysis yourself as well. Reading up on a lot of this is helpful.
Erin May [00:20:20]:
Amazing. Yeah, and up for folks. We've got people who are listening that are researchers, uxers, product managers, designers, research ops. So you know how someone in a different role that is, or adjacent to research, might think differently about how their work is impacting revenue. Any tips or guidance on how you might vary how you think about it depending on what your specific role is?
Claudia Natasia [00:20:45]:
Yeah, I'll start with researchers and then we can talk about PM research ops and design. So with researchers, you are essentially at the forefront of discovery, and so you are responsible for discovering the problems that will ultimately lead to solutions that drive impact. And how I would encourage researchers is as you're thinking about the types of studies, the types of generative work that you want to do, the types of evaluative work that you want to do, that's important. Back to the first thing I said in this discussion. Find the most impactful revenue metrics for your business right now. And if you are in a private company that's usually on a deck, someone would share it during a town hall. Usually the CRO or the CEO would share it during a town hall. So pay attention to what those goals are and ground your study in those goals.
Claudia Natasia [00:21:36]:
And even if it's a stretch, that's completely fine. Stretch yourself to actually figure out. Let's say the goal is to drive fifty k a new ARR. How the heck would this research study drive fifty k a new ARR? It might not necessarily directly drive fifty k a new ARR. But you can discover optimizations or new products that can lead to contributing to that fifty k a new ARR. So I'd always ground yourselves in those metrics. And if you're at a really, really large company, read the ten k. So read the financial reports that are all public.
Claudia Natasia [00:22:06]:
The first part of the financial report is always the business goal that meta or Google or whoever wants to achieve. And that's where you can even ground your small team or your smaller projects on these larger goals and actually impress the people that you work with, that you are tied to overall making the business a success. So focus all of your discoveries on those goals. For researchers, let's talk about PM's. PM's are usually a little bit more informed on what the business goals are just from the nature of the role. So at that point you'd want to make sure that the strategies, because you are essentially the bridge between all of these different teams, you'd want to make sure that all of the strategies that you create aligns again with those business goals and that you bring in the right types of input to align with those business goals. I think some of the most successful PM's that I've had the pleasure of working with usually are able to marry all of the insights together in a way that's meaningful, not in a ten page deck with a bunch of different inputs, but in a way that ties directly to ultimately the business goal that you want to achieve. And over time, also creates a learn and grow environment where six months ago we learned from implementing this product and we discovered that it in fact did not drive MAU.
Claudia Natasia [00:23:19]:
These are the patterns of why it doesn't drive MAU. And this is why we're doing something differently now. So for PM's, it's pattern recognition as well, through insights for design. I think the one thing that is extremely, as I'm thinking about the designers that are very the designers that really made an impression on me with how they utilize insights is all of us has the ability to affect change in our organization, but designers are the makers. And so how I've seen designers interact with insights is the most powerful way is running studies where they do live edits of design. And the reason why I gave a very specific example for this is I think back to one designer who was testing out this particular product experience and had a team of designers as users gave feedback that quickly worked on updates on the design. So on the same session they would show updates of the design and continue to iterate live with the user. So creating this culture of community where we're gathering insights live and making quick implementation is one way that design can have their own impact as well and ultimately drive stronger community, which does drive stronger lifetime value.
Claudia Natasia [00:24:31]:
And with research Ops, one of my favorite roles is the most important thing about research Ops is you are essentially a little bit similar to the PM, the keeper of all of these different types of insights. So any ways you can figure out the signals that matter for a particular project? I would encourage research Ops actually to make it a responsibility for you all to understand financial metrics, financial objectives, business objectives, and to also understand what competitors are doing. A good example of how you can do this is read VC reports. Where are all of the investment dollars going in this year, especially tied to your business industry? And by understanding that, then you'd know how to push or encourage all of the teams that you support to focus on certain areas, to focus on certain insights, to focus on certain projects, to meet person a, person b, to influence exec A or exec B. Amazing.
Erin May [00:25:23]:
And as you know, each of these.
Carol Guest [00:25:24]:
Functions has sort of different strengths, right, in terms of what they're bringing. Research can ask deep questions and dive deep with all types of different users. PM often has more of the business context design, bringing a lot of implementation and solutioning. Any thoughts on how these different teams can work together collectively to. I guess I should say all of them have an eye toward impacting top line metrics. Thoughts on how the team can work together to triangulate on most important problems to solve, especially those that drive revenue.
Claudia Natasia [00:25:51]:
The most practical way to do this is to make sure that you all have a forum or you can have these types of discussion and someone always needs to start it. And a forum is not a meeting once a week, because I feel like when it's a meeting once a week, people usually come excited. But then if it's a live discussion, there's always one or two people who don't feel comfortable speaking up. So then that creates that culture moving forward. How I've seen this work, companies after companies, something as simple as a slack channel that's specific to a roundtable of cross functional peers that care very deeply about insights. And in these roundtables, I've had researchers and PM's start posting something first, or even automating, sharing a particular insight. I think user interviews does something like this where we can share insights on slack. Yeah, absolutely.
Erin May [00:26:43]:
Yeah.
Claudia Natasia [00:26:43]:
So making that part of the culture and then tagging people like, let's say a particularly exciting insight from user interviews is shared on a Slack channel. How I've driven the skills. I would tag a particular PM from a different team and the PM would start discussing it and bring in the PMM. And so it just needs to start from somewhere. If you all don't have it in your current organization, I'd encourage you to start it. And that's how you get people excited to collaborate with the insights and to bring different voices and data together.
Erin May [00:27:15]:
Awkward interruption this episode of Awkward Silences, like every episode of Awkward Silences, is brought to you by user interviews.
Carol Guest [00:27:22]:
We know that finding participants for research is hard. User interviews is the fastest way to recruit targeted, high quality participants for any kind of research. We're not a testing platform. Instead, we're fully focused on making sure you can get just in time insights for your product development, business strategy, marketing and more.
Erin May [00:27:40]:
Go to useriterviews.com awkward to get your first three participants free. Claudia, it struck me when you were talking about the designer that these are the makers, the creators. They're really taking insights and turning them into reality. At least the first critical step in that each person might be playing multiple roles in this product development lifecycle. But just to kind of summarize what we've talked about so far, at least part of it you're talking about, okay, you got to understand, what are the top line metrics that map to revenue that the business is focused on right now that can come from those decks that are being presented in all hands is a great resource that you mentioned. But you want to have that in mind when you're selecting your research projects. Your research projects are then going to yield insights, hopefully that help you get closer to, you know, being able to push those metrics forward, those revenue related metrics. And then there's this special part that happens with the designer, right? Which is, okay, here's the insight.
Erin May [00:28:36]:
What am I going to do with it? How am I going to turn that user need into something that fits with that business need? And that's kind of the magic of realizing revenue actually happening, right? And then round and round it goes. We have tons of questions from our audience, and I think it would be great to jump into some of them. Let's do it. All right, so first question. I hear this a lot, right? So research, particularly discovery research, strategic research, the development that results from it can take a while. You could start a research project one year. We're developing for years into the future. Any tips on folks there? The revenue realized from a research effort might be long into the future and how to kind of talk about those sorts of projects.
Claudia Natasia [00:29:24]:
I'll use the example of marketing as a function that actually shares similar characteristics to research. But does this so well. When you run a marketing campaign or an advertising campaign, it takes a year or six months, minimum to actually see that campaign have an effect. I used to work on advertising products, and that was one of my first jobs out of college. And I remember launching something, and I was like, oh, my gosh, nothing's working. And the thing that my boss at that time told me is know, like, chill out, Claudia. Like, this takes time. And so if marketers can feel comfortable with spending millions of dollars on campaigns while waiting to have an impact, then researchers should feel comfortable as well.
Claudia Natasia [00:30:05]:
And I think the reason that marketers feel comfortable is they've built a practice that allows them to have continuous conversation as their campaigns are achieving their goals. And so tips to do that is, while you're undertaking a research project, if you know that it won't have immediate impact, start socializing, even from the beginning of the research project, the impact that you hope to achieve and how long it'll take to achieve that impact. So the hope is like, let's say you're tying this research project to driving 20% of the ARR of the company, the annual recurring revenue. Then you'd say, I envision that the ARR will be contingent on launching these three products, and they'll take seven months. And so setting that expectation from the beginning helps. The second tip is, as those seven months happen, as you run research studies that ties to that ARR, I'd also recommend sending routine updates to execs, the influencers in the company, the people part of the project about the insights, and tie it specifically to, let's say you discover one of the insights is users interacting in a particular behavior, interacting with a particular product that you hypothesize will drive the array. Highlight that as an insight to the ARR goal. And so, yeah, just to recap, setting expectations from the beginning on the goals that you want to serve and then sending routine updates to keep the influencers and the execs informed on exactly how you're achieving.
Claudia Natasia [00:31:26]:
That helps with the entire journey.
Carol Guest [00:31:30]:
We have a related question here from someone here on the call, which is, how do you check back in on impact? So some of these do have a long running impact. Are there any processes that you've seen where teams are?
Erin May [00:31:40]:
Yeah.
Carol Guest [00:31:40]:
Checking that we actually had the impact that we expected of some of these launches or some of the research?
Claudia Natasia [00:31:45]:
Yeah, it depends on the type of product. And this is where it's both an art and it's mostly a science, but a little bit of an art as well, because you don't want to check too early where you abandon a product and says it's a failure. But it turns out there were certain user behaviors that users need to do before they end up liking a product. A good example is when Facebook launched Newsfeed a few years ago. I'm sure everyone saw that happen. And if you were like me, I complained and I wrote so many messages.
Erin May [00:32:13]:
To them.
Claudia Natasia [00:32:16]:
I actually got a job interview because of the messages. But that's a different story for a different day. So had Facebook abandoned Newsfeed, then they wouldn't have the platform that allowed them to build all of the amazing features that they have right now. And so it took a while for them to be comfortable with launching it, to pay attention to user feedback, but also ignore it in a sense of like, just wait a little bit for a little more. I think the best way to ensure that you are checking at the right timeframe is make that part of your hypothesis. So if you're launching a product, make that an active part of your hypothesis that it's going to take a month for this to really stick and then check back at that cadence. Or if it's a retention product, like if, you know, actually, let me, let me frame it this way back to the goals of acquisition and retention. If this is a new product and you're trying to acquire new users, the timeframe would usually be a little bit more.
Claudia Natasia [00:33:09]:
If it's a retention product or an optimization where you already have users and you're adding a new experience that they've asked for, or you are optimizing an existing experience, then you'd want to check in pretty quickly, maybe a week or two after launching, seeing if it's in fact driving the metric that you want to drive. And the third category of products I'll add is if it's a trigger product. A good example of a trigger product is an alerts or a notification system where it's very clear that it's supposed to trigger a particular action, like clicking on a CTA, checking the platform. If that action doesn't happen directly, then you know that what you're doing is really not landing with them.
Erin May [00:33:47]:
Awesome. Awesome. And to put a finer point on the retention example, you might have some leading and lagging indicators there, right where you're trying to move a behavior that you know is related to retention. And it might take you three months, six months, a year to see if that actually played out, particularly for, you know, an annually recurring kind of product. So that can be helpful too, to have your leading and your lagging indicators that you're watching.
Claudia Natasia [00:34:09]:
Yeah, exactly.
Erin May [00:34:11]:
Awesome. We've got a question here from Jen, who is asking a question from the agency side or consultant side. So I don't know if you've had agency experience, Claudia, but I'm sure you've worked with consultants. So when you're thinking about impact on revenue internally versus as a consultant, obviously those are kind of different scenarios. So any tips for someone who's coming in more as a consultant to sell in the value of research at all, or perhaps an expensive or long term project and how it might impact revenue?
Claudia Natasia [00:34:41]:
Yeah, when I've worked with consultants, what I've noticed is the projects tend to be more time bound. So when you agree to do a consulting project and feel free to follow up if this is not the case for you, and I'm happy to answer for your specific use case, but usually for consulting, it's more time bound. So when you join a company to consult, there's already a thesis that maybe the exec or the team that hires you needed help on. And because of that, two problems that often come is you're limited in the types of insights you can gather during that period of time. And to Carol's question earlier, also, you are also limited in being able to measure your impact. Right. Because at the end of the day, maybe you're already gone. At that point, your gig is already over and then the impact happens later.
Claudia Natasia [00:35:31]:
So usually when I've worked with consultants before, what I've encouraged them to do is focus more on gathering the secondary data that already exists. So start by figuring out what are the user research studies that they've done? What are the user research notes that the company has done? What are the product analytics that they have? And then starting there, before coming up with the new studies that I want to run, the new data that I want to collect, and then combining all of those together, it helps save some time from the beginning. And it also helps you understand that there is already, like, what is the ground zero of the company? What are the known knowns? And how can we build off from the known knowns to ultimately add value?
Carol Guest [00:36:15]:
Another question here from Agnes. You talked earlier about trying to connect your research, your investment, to business metrics. If you're working on sort of a secondary product, like Agnes mentions working on an analytics platform that then drives revenue. Any recommendations on how to do that? Sort of second step to revenue in a clear way?
Claudia Natasia [00:36:34]:
Yeah. Yeah, that's a really great question, Agnes. I worked on a product for. It was basically making kubernetes more efficient, and they had an entire research and data team to support that product. And it doesn't necessarily drive revenue, but what. What it does is drive efficiency, which ultimately improves the bottom line of a company. So revenue is not the only important metric for a company, especially now in this market. Anything related to operational efficiency, anything related to increasing profit margins and making it easier for sales to land deals, making it easier for engineers to do their job, those are important metrics as well.
Claudia Natasia [00:37:13]:
So I'd encourage you to tie the analytics products or the investments that you're making on other metrics that it might be helping with. For example, faster decision making or efficiency in decision making. And this, I'd like to extend this answer also to researchers who work on internal tools. What I've heard from a lot of researchers in the field that work on internal tools is there's a lot of fear that people won't pay attention to internal tools anymore because it's not driving revenue. And maybe that might lead to layoffs or unfortunate circumstances in the future. I think that's a completely valid fear. I think as researchers or product team members that work on internal tools, let's encourage each other to just be better at measuring all of the other different types of impact that we can possibly have, including operational efficiency. And these are metrics that could be measured as well.
Erin May [00:38:09]:
Yeah, and I hear you saying a lot, Claudia, you don't have to force it, but even if you're several levels removed from revenue, the connection, maybe it is three or four, and that's okay. But draw it out. Like, literally, this relates to this relates to this. It's an input and output. Right. And it's good practice to have a sense of what those are. It doesn't have to be exactly right, but just a general idea.
Claudia Natasia [00:38:29]:
Right, exactly. And speaking the language, just being able to mention, I want to run the study because I'm confident that it has the potential to drive ARR. And this is why, I think, as researchers, because we are so trained for attribution in our head, it's like, oh, my gosh, we have to attribute every single thing. This has to drive this. We work with human behaviors and very, so often it's impossible to perfectly attribute everything. So it's okay to start leaning in and practicing saying all of these metrics, hypothesizing and. Yeah, and just to Erin's point, take the first step and just start talking about it.
Erin May [00:39:06]:
That can be a great way to dialogue and actually learn more and get better at this practice as well when you're working with more revenue focused teams, like, actually, I think about it more this way. Great. Now we're all speaking more the same language, so moving in the right direction.
Claudia Natasia [00:39:19]:
Yeah.
Erin May [00:39:20]:
Someone asked, I imagine this probably happens a lot that, you know, you've got a project, you're working with more junior stakeholders in the organization. Maybe it's scoped as a smaller project or for whatever reason, but you really, you know, start to believe there's value in a bigger sphere here. This could really impact the entire organization or something bigger than what I'm working on right now. Any tips to kind of take that project to a more senior audience to potentially get more budget, more buy in to make sure that that has as much impact as possible?
Claudia Natasia [00:39:53]:
My biggest, most tangible tip is actually to create an alert or a newsletter system that goes to the execs and goes to the influencers that you want to influence. And I know that this seems like it's very basic, but in every single company, my teams have always created what we call a skim, and it goes out weekly for smaller companies, sometimes biweekly. Once we did monthly, and people were like, bring it back to weekly. But essentially creating this summary of the high level insights and how it ties to the business objectives. So the format is usually start with a business objective that everyone cares about right now. And then all of the insights that tie towards that helps create this cadence of execs being interested in what you're working on, in the insights that you generate and helps you build a presence over time. It doesn't happen in a day. If you constantly provide good things, an exec will open their laptop in the morning, see that, and always find something that's interesting to look at.
Claudia Natasia [00:40:52]:
Maybe the CRO is only interested at something you send next week, but today the CTO is interested in a finding that you have, and it starts discourse that way. So I'd encourage everyone to do that. Just making sure you remain salient through creating these newsletters. And often what I hear is there's barriers to entry of like, oh my gosh, will they even read it? It's kind of scary to send an email. I don't think it is. It's, again, just taking the first step and taking that chance.
Erin May [00:41:17]:
Got it.
Carol Guest [00:41:17]:
So sort of this regular reporting on insights. We had a related question on are there annual metrics you should track for research? So thinking beyond a regular update. Is there something that you tend to summarize at a higher level?
Claudia Natasia [00:41:29]:
I tend to align the regular metrics to one business goal and one product goal that we want to achieve. So an example from my last company is a business goal was achieving a x percent growth rate. So the product and research and data science team aligned to achieving that x percent growth rate, and then the product goal that we had was tied to the growth rate. To achieve the x percent growth rate, we need to increase sharing of a particular product that we had by x percent. So we aligned all of the initiatives against that high level goal as the single most important thing that we need to do this particular year or this particular quarter. Now, the reason why I limited the team to those two goals is I've seen teams fall prey and to paying attention to 15 to 20 goals, and then at the end of the day, you just run into analysis paralysis. So I think I'd encourage everyone to really, like, evaluate on what matters and align yourself to just a few goals that everyone can live and breathe and remember every single day about. And beyond that, I'd also encourage, especially if you're research leaders or product leaders, to encourage having one or two additional goals that are not tied to the business, but more for your team.
Claudia Natasia [00:42:50]:
One product leader. Two years ago, after I have this community of people that talk about finance and research, in case anyone here is interested, also joining, it's just a slack community, and I still keep in touch with a lot of them. And this one person from a company in Southeast Asia actually reached out and saying that, hey, we took your advice and we implemented an actual business metric goal, but we also implemented a goal of everyone has to have one discussion each week talking about finance. And now he told me that they're actually doing really well. And actually, two of his researchers ended up talking at conferences in Southeast Asia about measuring the financial impact of research, which is amazing. So I'd encourage you all to have goals like that as well.
Erin May [00:43:33]:
I love that you can do a bartering system, right? Like on the business side, it's, you know, all right, you got to have a weekly conversation about customer insights and then vice versa and cross pollinate, because, you know, it's all one team trying to get to the same thing. Happy customers with making some money.
Claudia Natasia [00:43:48]:
Sure.
Erin May [00:43:49]:
Awesome. All right, let's see. So while you're talking about the finance and research, someone asked, and we can put this in the show notes, and if you come up with ideas later, we can add them as well. But folks want to know what your favorite resources are to learn about business and finance terminology as it relates to Ux.
Claudia Natasia [00:44:07]:
I'll link a corporate finance book that I know. It's not a textbook, I promise. Everyone might not necessarily like reading textbooks. They summarize the insights in a they summarize the top 15 most important financial metrics with sub metrics that fall under those metrics. And I feel like it's a good first step. Everyone in the community read that book and then we had a book club and it's a really good first step to just understanding the language. So that's the first thing that I'd encourage everyone to read. The second thing is reading VC reports.
Claudia Natasia [00:44:39]:
So I brought this up earlier in the call. VC's usually launch industry reports where they talk about the product perspective discovery. VC's actually do their own user research as well. They call it due diligence, but it's essentially talking to users to see if a particular space is interesting for them to invest in. And so they write these reports almost like the perspective of a user researcher writing the reports, except they tie it to financial metrics. So it's a good way for you to practice linking both together. Reading VC reports from battery venture, sequoia from every single VC publishes reports. Actually the third way is also reading books about startups and the reason why books about startups is important.
Claudia Natasia [00:45:20]:
One of my favorite one, actually I have it here, is I'm sure some of you have seen this, it's zero to one by Peter Thiel. It's a really great book. And the reason why those are important is because it shows you how to implement all of the theories that you've learned from that corporate finance textbook, from the VC reports into something that's more tangible, using examples of how great startups were built through all of this.
Erin May [00:45:45]:
Great.
Carol Guest [00:45:46]:
I can't wait to link out to all these resources. So it sounds like we have a sort of a textbook, some information on how to think about business and finance within businesses, some ideas from venture firms or transfer venture firms, and then reading about startups. Yeah, so we'll be sure to link to all those in the show notes as well.
Claudia Natasia [00:46:02]:
Yeah, for sure. I'm going to switch gears a little.
Carol Guest [00:46:04]:
Bit, so we had a lot of questions about attributing the impact. You mentioned this slightly, but attributing the impact of research when looking at something that should drive impact, because, you know, often user research is working with a number of different members of the team and product managers, designers, engineers, etcetera. And how do we essentially, how does the research team get to take credit for their part and of the insights and the impact? So, any thoughts on that?
Claudia Natasia [00:46:28]:
That's a really great question. A metaphor, I guess. It's not really a metaphor. A connection that I want to draw is with advertising. When you work on advertising products, you tend to measure less hit, which is a person. Me could have been exposed to five different forms of ad to buy this zero to one book. It might be some magazine that I read, or it might be a billboard. But eventually, what advertisers attribute as the main impact is the last hit.
Claudia Natasia [00:46:57]:
So the Facebook ad that led you to buy the book, the Instagram ad that let you to buy the shoes, and I think with researchers it's the same thing. So you might have an impact on something. It might not be the last impact. The last impact would be the people responsible for actually shipping the product. But it doesn't mean your impact is less. It's just very easy for people to tie the impact to the last hit. So I'd encourage everyone to be part of that discourse, I think related to that whole newsletter suggestion that I made earlier. If you have a way or an avenue, whether it's the newsletter, whether it's creating.
Claudia Natasia [00:47:37]:
We talked about creating a community slack group earlier. These are ways that you can always remain part of the discussion and include it in the discussion. And therefore, even if you're not necessarily the last hit, you are seen as someone who contributes to the overall success of their product.
Erin May [00:47:53]:
Amazing. Amazing. Yes. We think about multi touch attribution all the time here in marketing. Great example. Let's wrap up. And there's so much we could talk about with research impact on revenue, and a lot we've tried to cover in a brief period of time. But maybe just some parting words of wisdom or inspiration to leave our listeners with.
Claudia Natasia [00:48:11]:
Claudia, the first thing is be courageous and start talking about research and finance, and be comfortable with talking about these maybe financial terminologies that you might not be familiar with. I think part of pushing our practice is being able to have these type of conversations. And if you don't start, you will never feel comfortable doing it. Usually what I've seen happen is not being able to start. So I'd encourage everyone to feel comfortable starting. And then the second thing is, don't be afraid to triangulate data. There's so many ways that you can do it. There's so many data sources that you have.
Claudia Natasia [00:48:48]:
The companies that are successful are the ones that are comfortable triangulating data and doing the hard work because that's how you find the insights that other people can't find. If you're just relying on user research or if you're just relying on product analytics, most of your competitors are probably doing the same thing. So they're uncovering the same stuff that you are, they're talking to the same users and uncovering the same things that you are. But if you find a way to combine all of these insights together, distill meaningful signals and come up with strategies that way, that's where you can actually differentiate and find what's meaningful that other competitors might have missed. So don't be afraid to be a little bit more creative with that.
Erin May [00:49:23]:
Awesome. Awesome. Yeah, Claudia, there's a lot of questions in chatter, in the chat about some of the interesting methods you mentioned about the cloud, the AI assisted analysis of data. I think we're gonna have to have you come back and do a webinar and get a little more into the details of some of that because there's definitely an appetite to learn more. So we'll share some more resources, getting a little more tactical with that, but be courageous. I think a great tip for everyone in every situation. So love that. And for folks not in the chat, you can find Claudia on LinkedIn at claudianatasha.
Erin May [00:49:55]:
That's n a t a s I a. Thanks for being with us Claudia. I really appreciate it.
Claudia Natasia [00:50:01]:
Thank you everyone, and please feel free to connect with me on LinkedIn. As you know, I love talking about this topic and answering any questions that you might have. So yeah, please feel free to follow up and reach out with any questions. Thank you so much to the user interviews team for having me here.
Erin May [00:50:14]:
Thank you. Thanks for listening to awkward silences brought to you by user interviews theme music by fragile gang hi there, awkward silences listener thanks for listening. If you like what you heard, we always appreciate a rating or review on your podcast app of choice.
Carol Guest [00:50:46]:
We'd also love to hear from you with feedback, guest topics or ideas so that we can improve your podcast listening experience. We're running a quick survey so you can share your thoughts on what you like about the show, which episodes you like best, which subjects you'd like to hear more about, which stuff you're sick of, and more just about you. The fans that have kept us on the air for the past five years.
Erin May [00:51:05]:
We know surveys usually suck. See a episode 21 with Erica hall for more on that, but this one's quick and useful, we promise. Thanks for helping us make this the best podcast it can be. You can find the survey link in the episode, description of any episode, or head on over to userinterviews.com awkward survey.