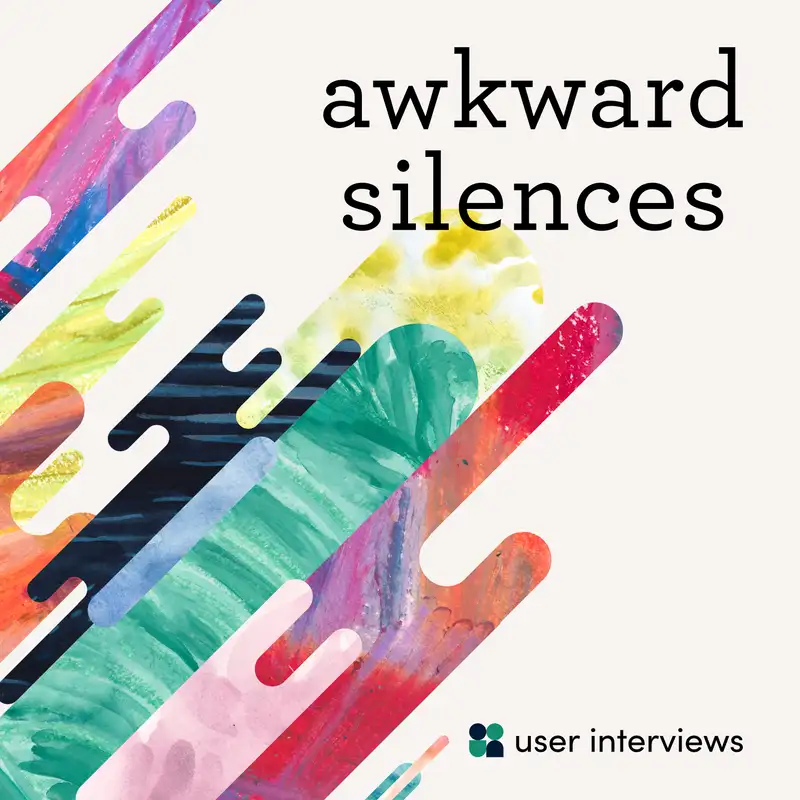
#75 - Debunking UX Research Myths with Zach Schendel of DoorDash
[00:00:00] Zach: We helped somebody. We helped somebody get somewhere faster or we helped somebody be more safe or something along those lines. There's lots of fun examples of things that you use every day that you have no idea that I worked on, or my team worked on at some company that I used to work at. it makes me really happy to go to sleep at night, knowing that you have gotten those benefits.
[00:00:21] Erin: Hello everybody. And welcome back to awkward silences. Today, we're here with Zach Schendel, the head of research at door dash. Today we're going to talk about diffusing UX research preconceptions. Should be a fun topic. Thanks for joining us Zach.
[00:00:56] Zach: Yeah. Thanks for welcoming me.
[00:00:58] Erin: We've got JH here too.
[00:00:59] JH: Yeah, I probably have a lot of these preconceptions in my head somewhere. So I'm excited to work through them.
[00:01:04] Erin: Awesome. Zach. So, so just to jump right in here, one of probably the most common UX research, adjacent preconceptions is about the faster horse, right? So, if we'd ask people what they wanted, you know, Henry Ford, they just would've said a faster horse ergo who needs research people don't know what they want.
Let's dig into it. What's wrong with that? Or what's not wrong with that. Is there some truth there?
[00:01:29] Zach: There is actually some truth there. I think if I did ask people what they would want, they would probably tell you that they wanted a faster horse, but I don't actually think that really is the point of research. I think the point of research is to show you the things that they want and then for you to be inspired by those things in order to make some sort of innovation that is going to move your space forward.
So if you're in research in the 1890s and someone says, I can't get from point A to B fast enough on my horse, then what you should take away from that is like, there's a white space opportunity here for something to help them out to solve that pain point. And it might not actually be a horse. It could be something else.
It could be a car and maybe the car is what you come up with. Maybe it isn't, maybe it's some other form of transportation. I don't know what you come up with, but the research is there to uncover the pain points. Not to tell you what the solution is.
[00:02:23] JH: Yeah. I mean, that makes a lot of sense. I think what people always miss about that is that you just hear the quote out of context, like just a faster horse. And it's like, because they need to get somewhere faster. Like it's a very reasonable thing when you actually take it that step further and understand what the underlying need is.
How do people do that though? Like, is it on the researchers to make sure that they're framing what they hear as the need? Is it for other people to be inspired and find innovations? Like where does that handoff or collaboration happen?
[00:02:49] Zach: I mean, I think both sides need to come together really on this one. So, usually where this quote is coming from is that a partner has potentially had challenges with research in the past. They may have limited patience with what research can do. Maybe they're biased a bit so they might pull the plug after research doesn't work the one-time that they tried to work with a researcher, but then, on the other side, you have researchers who might be a bit overzealous.
They want to matter. They want to make an impact or prove themselves in some kind of way. Maybe they have a chip on their shoulders, but it turns out that there's a bit of a flywheel where maybe a researcher isn't doing the greatest research and maybe their product partner isn't actually giving them the opportunity to do great research.
Sometimes a product partner might say, Hey, sure. Let's do some research. But, I need to see it in two weeks and I have, I'm pretty dug in on my opinion, so good luck trying to change my opinion. Right? So ultimately I think both sides need to really think about what the shortcomings and what the positives of research are.
And it's not perfect. It's not trying to predict the future. I think that's a fool's errand. I think really what research is trying to do is to increase your chances of success. And so anything research can do to help you as a partner, like be more successful then that is successful research.
I have a couple examples if you want to go through them.
[00:04:12] Erin: We love examples, let's hear them.
[00:04:13] JH: Yeah, Yeah. I was gonna say, it sounds like this is common, right? People show up with a feature request like, I want this. But it's maybe not the exact right way to go about it. What, yeah. What are some of those?
[00:04:22] Zach: Yeah. So like, you know, the quotes that we just talked about, like, if you ask people,what they wanted, so the wanted I think is the key term in there we asked people what they wanted a lot at Netflix. Usually at the end of qualitative interviews, it'd be on some topic at the end and be like, oh, is there anything else you want to tell us?
And there were some tried and true responses we would get. One of the things that people would often say was. If you could just give me an alphabetized list of every single movie and TV show that you have available, then I could go through it and I can find the things that I would want to watch. You see this play out on the web.
You often see articles come out and say like, Look, I've got all the secret codes for Netflix or these secret categories. And really all those are just web addresses that are associated with some combination of tags, like sci fi movies from the 1980s or a strong female lead, you know, whatever movie.
[00:05:18] JH: I just learned about these. They're cool, but probably not used very often.
[00:05:22] Zach: They're not secret codes though.
[00:05:24] JH: Yeah.
Yeah.
[00:05:24] Zach: Yeah. So what you find here is that really that's not the answer to the solution. It's a terrible answer to the solution, to their problem, in fact, because. There's kid shows. Okay. You're not five years old. Some things don't have whatever language you speak in subtitles or dubbing.
So you can't even watch it. A lot of the genres you don't even like. And so giving someone unfettered access and some sort of organized list A to Z isn't actually going to help, and obviously algorithms are going to be a superior experience to that. But what the goal is in research isn't to listen to that, it isn't to say like, oh, that's the idea.
Let's go give them this list. The idea is to say, what's the need behind that statement and the need behind that statement in this case is I don't trust you. I don't trust that you're giving me the good stuff. You're hiding the good stuff from me. Give me everything so I can choose for myself. And so what you need to hear there is the lack of trust.
And what you need to do is create product solutions that solve that lack of trust. Not just give them verbatim what they ask.
[00:06:25] Erin: Yeah, really important research skill, right. Is like hearing what people are really saying. And I'm curious, like, do you have any that comes from experience? I think right, how do you do a good job of inferring what someone really means without being, I don't know, like patronizing, like, like reading too much into.
Do you know what I'm saying? Like, like how do you get the right level of inference versus assumption, I guess.
[00:06:49] Zach: Yeah, that's a really good point. Another example that I think will illustrate that is we were working on parental controls at Netflix. They exist. You might not know about it. That's a bit of a spoiler alert. But you can set them and, you know, you can blacklist shows or you can pin protect profiles for your kids and stuff.
And you know, you survey parents and you sit in rooms with parents and you ask them questions about this, and everyone loves it. Everyone wants to use it. Because of course, like everyone wants to protect their kids. So, you think to yourself after this research, Hey, like this is going to be amazing.
It's going to be the most popular feature we've ever created in the history of innovation. But as a researcher, you need to know what you're hearing your people are telling you like, this is it. This is gold. I'm so excited to use this. I'm going to save all of my children. But if you, you need to think about other types of research methods that can give you a better view into how they might actually behave were you to launch something like that.
And there's competitive products out there. There's other products that have parental controls on them. You can talk to people who use them and don't use them. You can look up how popular apps are like screen time or things along those lines.
The popularity of these things is minimal. And we did our due diligence. We looked around and talked to people, and got some behavioral data on usage of these competitive apps. And so we were able to through the conversations with parents know that there's a lot of passion there but through actual behavior looking at the competitive set to temper that excitement down.
And as researchers, we didn't go and say, Hey, everyone's going to use this. We said, everybody wants this, but not that many people are actually going to use this, but it's definitely the right thing to do, particularly for the parents that do need it. For some government regulations that require these features in some countries and other really big benefits.
It's the right thing to do. And so we launched the feature, not, you know, 75% of parents do not use the feature. Right. I mean, it basically, you ended up at about where we expected to end up, but I think it's about a bit in this example, it's a bit about triangulation. It's a bit about knowing what your limits are, a bit about knowing like who you're talking to and how they might be expecting you to react with themselves, to react in this context.
[00:09:11] JH: And so in that example, like to make sure that you're leveling with the stakeholders and things like that, are you kind of like bringing in some of this sentiment, like along the way, or did you do the methodology in such a way where you were able to kind of preempt it? Because it seems like you lose a lot of trust if you go out, you do this research, you ask people to predict their future behavior.
They tell you that they're going to be, you know, super well-meaning and do all the right things for their kids. Then they don't. And if the research had misled the, you know, the product manager, whoever, then you damaged that relationship. So is that something you were able to avoid in this situation?
[00:09:39] Zach: Yeah so, I mean, just so you know, a lot of the examples I'm giving you are not just things that I've done. There's a lot of credit that goes to a lot of other people on research teams that I've worked with. I didn't actually run this project, but the kids researcher that I worked with at the time went straight to the competitive set.
I mean, she had done enough projects to know. When it comes to kids, parents are always trying to say the right thing, or I do this for my kids. I want to keep them safe and all this kind of stuff, but their behaviors sometimes don't actually match the words that they say.
So we started with, we doubt that many people will use this and then we went to, but it's the right thing to do. And here's what we should do in order to make it successful for the people that will use it.
[00:10:19] Erin: Yeah, that's great. So, what I'm hearing in both examples is like, it's not an invalid question to say, what do you want? But different scenarios, different kinds of problems, different kinds of solutions require a different filter on what you do with those answers.
[00:10:35] Zach: Yeah. So there's ways of getting around, asking these direct questions. A lot of there's a lot of indirect methods that you can use to get a sense of what actually would work. And by that you're removing a lot of the bias that comes in when you try to ask people what they want, and they try to give you the answer that they expect to hear.
I'll talk about one, one example that also worked really well at Netflix. We had a homepage that was a templatized experience. So some rows that you'll have heard of like continue watching my lists, popular on Netflix, trending now and there's genres and all those sorts of things.
They used to be in the exact same order for everyone in the entire world. And at some point, one of the algorithms product managers thought to themselves, but people are all over the place and there's a lot of irrelevant content that's being shown to people or our members that's way high up on the page.
And really people are making most of their choices from the top of their page, because I mean, people are kind of lazy. And so what if we tried to pack more relevant stuff up into the higher part of the page, but that would require you to personalize the homepage. It would require you essentially to change up the order of the rows for pretty much everyone when we have spent years creating this templatized experience.
And so the question, the research question was, am I going to make a bunch of people angry by doing it? And my answer was in my mind was I could go and I could ask people like, Hey, do you want us to do this? Here's the benefits. I'll move more stuff up to the top of the page. So you don't have to scroll as much and you'll find more relevant stuff, or I could keep it the way it is.
And it will be templatized. You'll know which rows are there, and you'll know where to go to find those rows. And if anything's not in those rows, you'll have to go to search, to find. And I did that on purpose because I knew it was a bad research idea. I asked them specifically those two questions, and of course everyone said, like, I just want you to keep it exactly the same.
And I want to be able to find the rows. However, I chose to do an implicit method in parallel to that research to find the real answer. And the implicit method was really simple. I just gave them a list of rows and I said, okay, put these in order. You've had the same Netflix homepage for years, put them in order from number one to number, whatever.
And they were terrible at it. They bombed it. They could, they maybe got like two rows, right. And then they just failed on the rest. It was just random guessing at that point. And so my conclusion based on that, Was that it actually doesn't matter. They're going to tell you that it matters because people want to keep things familiar.
And but they don't have this mental model, this mysterious mental model of how exactly to find something and how quickly, you know, they can quickly go to this row because they know it's in the sixth position. It just doesn't exist. And so they changed it. They made the change and you know, it was a huge engagement win, and whether or not people want to admit that they want it or not.
It's absolutely a better experience for them. It's much easier for them to find content that's relevant to them when those rows are personalized in order to.
[00:13:48] JH: It's a fascinating example. I like that implicit testing as well. I was going to ask how testing and doing research on algorithms feels challenging because some of it is like, You just like to interact with it and see if you prefer the recommendations and stuff. So that's such an interesting space.
Is some of it that people just lean so much on like the physical world for their mental models? Like the idea of like every time I walk into the CVS, the rows like the, you know, the aisles and moving around, feels just jarring or like, you know, dissociative I'm like, where am I? Well, if you went in and like, I need diapers and the diapers right at the front, and then I need cold medicine where the front like, probably would be pretty nice.
Right. So it's like, it feels counter-intuitive. I'm just curious if that's like part of it or maybe not?
[00:14:24] Zach: Yeah, no, I've heard this. This is there's many contexts where what you're talking about is critical. So I think the one that always gets brought up is grocery stores. If you bury the staples, like milk and eggs and stuff like that in the back of the store, then they have to walk through aisles to get there.
So on the way they'll buy stuff, right. There's a lot of truth to that, to be honest. And I've heard a lot of examples in the digital world where that is actually true. But what's interesting about this is that you have to make that balance.
Like you think of it as a balance of what we call netflix destination versus discovery. So I know exactly what I want. I'm going to go there. I'm going to find it. Same thing's true with DoorDash. Like I know I'm going to order a burrito at Chipotle tonight. So when I go to DoorDash, I'll just show me the burrito from Chipotle and let me reorder it and walk away.
The problem is A. You don't know exactly when people are in those types of moments. And when they're like inspire me, or I want to try something new tonight, or I have friends over and they don't like Chipotle, they have an allergy or something. So can you mix it up that nobody eats meat tonight.
So like, we're, I'm going to be vegetarian or we're gonna order vegetarian. Like you, you can't predict very well using algorithms, you can try, like you can get okay at it, but you're never going to know with a hundred percent certainty the state of the person when they walk into these experiences.
And so the right thing to do for a short-term and long-term win is to give people easy access to the things that they're likely to want, but also expose them to things that they could want in the future, but it's a careful balance. You don't want to overdo the difficulty like a grocery store might to get to the staples.
You want to bring those things up, you know, in reasonable places that you can access fairly quickly. If that's what you're looking for, maybe you jump through a couple hoops. Maybe you say, oh, what's new. Oh, there's this new restaurant. There's this new category. Oh, DoorDash has flowers, it sells flowers now.
And on Netflix, it's like, oh, this is the new movie that's out this weekend. That actually looks pretty cool. And if you don't expose them to that type of stuff, then they won't ever expand the ways that they use or engage with the experience. And so I think of those as planting seeds for future wins that are beneficial to both companies like a door dash or Netflix, and also to members.
Because they try something new and they like it, or they watch a new movie and they really like it and they get more value out of what the service that they've subscribed to, or the service that they're ordering from. And so the retention increases.
[00:16:59] Erin: Now I'm thinking of grocery stores, dark patterns, putting the milk in the back of the store. Cool. Let's talk about another conception folks have, might have some value. It might have some problems here. This one, a lot, some variety of. You only talked to eight people, you only talked to five people, you only surveyed a thousand people, your N is off.
You know, we need more people. What's the truth there? What's the problem there? How do you react to that?
[00:17:26] Zach: That's, this is a great one. This is a bit pessimistic, but in my experience, I generally only hear this one when the research doesn't match the preconceptions of, or the expectations of what we will find in the research prior to doing so, for example, on the survey side. Kind of the only times I ever hear anybody bring up response bias is when they were hoping for a different answer.
It's a bit of a crutch. But as researchers, we always have response bias, right? The same thing goes for praise. This is a good one too. The only the most of the time that I hear or I've ever heard either myself or my team get praised is when the research that they did like helped the person be more successful in ways that were kind of similar to what they were going to do, but maybe there's some big tweaks and big impacts from the research, but it's not like it's not a big leap to go from where they ended up and where they started from.
But the researchers are always trying to do great research. Like it would be great if there was praise for like, that was great research, like technically amazing. You got an amazing answer. I really believe it. But I'm not going to do it. And it goes against everything that I say, but it doesn't mean it's not great research.
So all of those things are looped into a single bucket that doesn't match my worldview and I'm gonna find, I'm gonna make some comment to like to try to make my worldview more accurate. And the worldview that you're presenting is less accurate. I'll give you a good example.
And this is going to go against the eight things, right? So like you only talked to eight people. In my opinion, sometimes you really only need to talk to one person. I think people might fall into problems with qualitative research where they try to turn it into quantitative research. They try to say things like, oh, most of the people I talked to like this or seven out of eight really thought this person was better than this version.
That's not really what qualitative research is for. That's what quantitative research is for. What you're looking for in qualitative research, it's that emotion, it's the clear I'm being drawn to something or it's that like, oh, that's so obvious. I should do that thing. So I'll give you an example.
There was a team that was doing signup flow research at Netflix, and it was just on general signup flow feedback. And they were just asking people to sign up for the whole experience. One person. In that research they got to the page where they had to enter their payment details. And it said something like enter your credit card.
And then they said, well, I don't have a credit card. Why can't I enter my debit card? And I think at the time you could actually enter your debit card. But like they didn't know, it wasn't clear to them. So they were like, oh, I'm not going to sign up. I don't have a credit card. And so they ran this test and based on that insight, they ran a test where they just added two words, credit or debit card to the signup flow.
And it was a big win. A lot more people signed up, they made more money like that. Those are the kinds of sparks of insights that you can get from doing research that they don't require consensus. They don't require you to get eight out of eight people like this thing. It is an insight that is clearly obvious and clearly could make an impact.
[00:20:48] Erin: So I imagine we could talk about this for a very long time, but sometimes an N of one is enough for a test like that. And sometimes like it isn't right. It's particularly to your point, you're not necessarily looking for seven or eight, but you're looking for some affinity, some trends, some themes.
Right. And so, what do you think about how many people do we need to talk to, to answer the research question we want to answer.
[00:21:52] Zach: Well, I mean, you have to design the research to answer the question. So if you really are looking for affinity, if you're really looking for opportunity size and things along those lines size the problem. Most often you should lean towards quantitative methods rather than qualitative methods, but researchers can fall into traps there too, where let's say a, a business partner stakeholder or whatever wants to know why something happens. Like, why aren't you doing it? Or what can we do to get you to sign up for this? Or what problems do you have with this thing? And they'll have a list of ideas of why they think that thing is happening. You may, you will too, because you've done a bunch of research in the past on unrelated topics and you can come up with some hypotheses.
And so then you go and you structure a survey and you ask them questions, like, why did you do this? Or why did you skip this step or whatever it is. And then you list off five or six ideas that you think might be the right ideas. You give them none of the above. You give them all of the above and that's it.
That's your question. And so what you it's like an input output situation. What you put into that is what you're going to get out. So clearly one of those answers is going to be the most popular answer because that's how surveys work. And so you think to yourself, well, that's the biggest problem we should go and we should solve that problem.
there's a big issue with going down that path. If you actually haven't done the work to find out what the problem set actually entails at the extremes and you haven't created a set of options. That is inclusive of all of the things that people might run into or all the reasons why they do or don't do something then you're fooling yourself thinking that you're going to get a good answer by just jumping straight to an opportunity sizing.
[00:23:35] JH: That seems like such a tough one to avoid because the thing you lead with right, is that other than just like knowing it's the best practice and doing the right thing cause people usually have internalized some possible wise, some probably valid off user feedback or other observations. Some completely just from their own perspective.
Right. And made up. And so is that just a situation where the researcher needs to be the expert and say like, Hey, we're going to do a round of qual and get like really flush out the spectrum of options here, then we'll size it. Cause it just seems like there's it's I could just imagine business partners.
There's like the momentum just building like, come on, we already got five of the possible reasons. Like we'll just throw them down there and see what happens.
[00:24:11] Zach: So do it the right way. That's exactly what you mean. I, you know, it doesn't take that long to have a few conversations with people and understand, you know, people who are using a feature, people who have never used a feature and just find out like, why are you using it? Why are you not using it? And then use that to create your question in your survey and send it out.
You had said a couple extra days, right? Maybe an extra week, depending on if you have translations, you gotta do it in a different country. I don't know what the constraints are but the benefit that you get from that is that. You have a higher chance of being successful because you're going after the right problem.
You're not being fooled into thinking that your worldview is the worldview of everyone. That's going to be using your product
[00:24:52] JH: On the, On the N of one thing, just to follow up there. Like, so the example you gave was a great one, right? And it makes a lot of sense. You hear it and you're like, oh, this is, yeah, this is an obvious insight. Is this just on the researcher? Or the people involved in this project to just have some amount of like product sense and judgment, to be able to kind of like point to those.
And that's just, and that's just part of being good at the job, or is that something you can develop? Like, you know, cause that person probably said, you know, a dozen other interesting things in that call, but that one was like the one that moved the needle, like how do you do that part?
[00:25:21] Zach: Yeah. I mean, there's a bunch of things that you need to be really good at to be a great researcher or a more impactful researcher. Technical skills. We're talking a lot about methodology and choices, and we've also talked a lot about partner relationships here. And, you know, when they come to you they're biased.
They don't want to work with you. I don't know. Maybe they're excited about working for you. Maybe you confirmed what they thought. I don't know what it is, but those are two for sure. Technical skills partner relationship but underlying all of that is a strong business foundation because what a mistake I see often made is that researchers will over-rotate to the needs of people that they're doing research.
And they'll forget that they're in a business, right? And really what researchers need to do is they need to understand the business context of pretty much, what is the business trying to do. And you need to also think about what the needs of the customers are and what your goal should be to be the most successful is to find out where those two things overlap.
So, I'll give you an example on DoorDash. We just launched a product feature called double dash. If you've ordered something on DoorDash recently you may have seen a bit of a notification pop up after you ordered. And it says like, Hey, you live near a seven 11, we'll pick up something for you on the way home with no additional delivery fee and think about the benefits of that from all of the audience's perspective and door dash from the consumer's perspective, it's great because they're not the one going out to get the food, but if they were, they might stop somewhere and pick up a milk or pick up some eggs or something, or get some ice cream or maybe get a Coke or get a six pack of beer.
I don't know what they want to do, you know, something for their kids lunch the next day or a project who knows what it is like they'll stop and pick something up. So there's this convenience aspect for customers and it's no additional fee or barely any additional time from a dashers perspective, you now have increased.
The amount of money that's being spent overall. So there's the potential there for more tips. And then from a merchant perspective you've now created an access point for an entity like a seven 11, or like a Walgreens or some convenience when someone actually needs to go there. When someone actually needs that thing.
So then they get in on the game and they, and as a business from the door dash perspective, we are now increasing awareness of other categories outside of our bread and butter, which is restaurants. So it's like a win. It's a huge innovation, a huge opportunity. And so as a researcher, what we need to uncover.
Hey people aren't using I'm not, I'm making this up. I don't have an actual example on this one, but let's say in a research project you heard, Hey, I'm not actually going to use DoorDash because I need a bunch of other things. And all you're doing is delivering from this one place. And so I'm going to go and do all of my errands at once.
And while I'm out, I'm going to pick up that thing that I would have ordered under. Yeah. Right. So that's the need in your mind. That's a need, but then you think in the business context, Hey, this is a great way to introduce new categories. Hey, this is a great way to increase the amount of money that a Dasher earns, et cetera.
So you can see how those benefits start over.
[00:28:49] Erin: Yeah. And it's back to the sort of discovery versus. Putting up front, what we know you want is kind of a dilemma or the different ways of finding things where, you know, I imagine if you're looking for that burrito from Chipotle a and we're just like, no, 7 11, 7, 11. It's like, well, That's not great. experience but putting it at the right time makes a ton of
[00:29:07] JH: That's great. Yeah, that's a great name too. It sounds like a Nickelodeon show or something like double dash.
[00:29:11] Zach: Yeah.
[00:29:12] JH: It's fun.
[00:29:14] Erin: A lot of alliteration.
[00:29:16] JH: Yeah.
[00:29:16] Erin: Another one of these kinds of preconceptions that come up in UX research, people can articulate what is good and what is bad. So I guess there are a couple of things going on there, like A. The ability to clearly or articulate you know, a sort of a preference. And then, you know, these sort of binary good and bad.
So what's right or wrong with that one?
[00:29:36] Zach: Yeah, so I called one of my social psychologist friends this weekend and asked him specifically. This one. And I want to explain to you what he told me. Because it's really interesting. He described it as like, people are pretty fickle. People are pretty lazy and they're not really aware of it, but it's actually to their benefit because if your brain really took in all of the possible signals, it could throughout the day, you would never be able to function.
You would never be able to concentrate. The task that you need to concentrate on your brain is constantly filtering out things that it deems to be unnecessary or not helpful. And so like your brain is essentially making choices for you, and you're not consciously aware of those choices that your brain is making for you.
And so to expect people to be able to consciously come up with some reason or justification for. Why they like something or what's good. And what's bad. You're asking for a lot of filtering. You're asking for a lot of like information that they believe to be true, but isn't actually true to pop up in those answers.
I, there's a couple examples that we talked through this weekend where there's this classic eye witness memory study that Elizabeth Loftus did where she asked or she created a questionnaire and asked people after they watched a video of a car accident, how fast the cars were going when they blank.
And the blank was different levels of extreme like bumps smashed, hit. I don't know. I don't remember the exact words, but essentially the way that the participants estimated the speed was influenced by how strong that word was. They were going at slower speeds when they bumped, but faster speeds when they smashed together.
For example, Um, another example that we've talked through this weekend was there was a study that somebody set up where there was a confederate of the researcher walking around the parking garage with a flyer and the confederate without would either throw the flyer on the ground or they would keep it in their hand.
And an unsuspecting participant would walk to their car and that same flyer would be on their windshield. And when they saw the person dropped the flyer 54% of those people also threw the flyer on the ground of their car. But if they sell them, keep it, then 32% of people threw the flyer on the ground.
So you can see in these examples, just how easy it is to manipulate people's behaviors in ways that you're not even aware of.
[00:32:22] Erin: And to manipulate their impressions, right? Like how they perceive reality.
[00:32:28] Zach: That's exactly right. So I'll give you an example from Unilever. I used to work on skincare at Unilever, and we were interested in premium ness, like creating a premium lotion experience. And we had this hypothesis that a lot of quote unquote, what is premium is fairly smoke and mirrors. And so to test that out, we took an actual premium lotion that we knew objectively it was expensive to make, we had made it ourselves and it had all the kinds of that stuff that you would associate with being premium, it was silky smooth, fast absorbing it. Wasn't sticky. It wasn't greasy. It left your skin feeling amazing, et cetera. And we took a regular cheap everyday water-based lotion that you took longer to absorb.
Maybe it was more draggy, draggy being the opposite of silky and in like sensory terms. And we took two packages. They were, they looked exactly the same. They were just white packages. And on those packages, we put different labels. That was the key. The label’s a really nice, white, smooth, premium feeling, soft label, and a label that you might find on something you used in the shower.
Like it, it has had way more grip. It felt and looked and was a bit shiny and a bit cheaper, but they were, everything was white. And they couldn't see the lotion, right. Because it's in the package and we just said, okay, what's premium? And it's all driven by. It was entirely driven by the packaging. Like you could fool people into saying like this super cheap lotion was amazing in premium just by putting it into this really nice feeling packaging. It didn't even look good.
And there was no brand associated with it. There's no like history or memory or any of these other bias signals being built into it. And so after I did that, it was more of a multi-sensory research project. After we did this project, it just dawned on me like you, there are so many things that can get in the way of you getting the actual right answer.
And a lot of the things that you're trying to do, it's not about actually doing the thing, it's about creating the perception of it.
[00:34:31] Erin: Well, what you're saying is interesting too, because like, if you're trying to learn which lotion, the actual content people prefer, that's one thing. But if you're trying to learn how to sell them lotion, then that insight is a real insight. Right? Cause it's the label that maybe disproportionally matters at least to get them to pick it up off the shelf.
[00:34:49] Zach: Yeah, that's exactly right. And if you can add on, you know, whatever the premium brand is onto that on top of that, that's even right. Right. I worked on anti-aging products too. You know, it's really hard to make people look younger, particularly. I mean, biologically it's very difficult and we're talking changes on the edges here, like really small percentages of changes and really the best way to look younger is just to take a time machine back to when you were younger, and wear sunscreen.
Like that's, that, that would be the best way to look younger, but. You know, but you have to create these experiences where you get people excited about the prospect of looking younger around pretty small changes that are definitely perceptible to the human eye, but they're not massive changes. You're not losing 10 years off of your life.
[00:35:41] JH: There's an element here, right? When you're talking about like all the social science stuff and all these biases, and to your point of all the shortcuts we take to navigate a day and all our habits and behaviors and triggers and cues is I can imagine especially researchers often being some of the more like ethical and academic minded people in an organization.
Like does some of this stuff get a little uncomfortable, like, like, Hey you know, we're actually not going to improve the quality of our wine, but we want to slap nicer labels on it and mark it up so that we can sell it from. Do people ever like push back or feel icky? Like that's not the type of research I want to do.
I don't want to know how to sell people crap and like nicer bottles or are people generally, because it feels like there's real things you can unpack here that are valuable and worth knowing, but you can also take it to kind of the dark pattern category pretty quickly. I'd imagine too.
[00:36:24] Zach: I would, that's a really good question. I, you know, I've never worked in a company that's ever done any of these manipulative tricks ever. So like none of that stuff ever made it to fruition. Like I was talking about the perception of premium ness. At Unilever that's not something that I have ever seen happen in a company before.
I mean, I know that there's studies out there that if you dye white wine red and people think it's red wine or experts, can't tell the difference. I don't know. I don't know all the details of all of those studies. I think there's ways that you can take advantage of these things. I would just hope that people don't and I've, so I've actually, I've never been a part of it.
I've never seen it happen.
[00:36:59] JH: Cool. Well, that's encouraging to know. Yeah. And it feels like to your point, like if you deploy them correctly they're really powerful. Because people have trouble changing their behaviors or adopting new habits or products. And if it's something that can really help them and you can figure out how to break into that loop and get them to consider it.
That's, you know, that's a win-win like, as you were describing earlier.
[00:37:14] Zach: That's true. You can also do the opposite as well. I mean, you can take people that have bad habits and you can try to introduce good habits. I worked on heart health meals at Unilever. It was a project. It was under multiple names, but the whole idea of the project was to give people what we called, like a heart score or heart health score.
So they would know where they live, what their internal health was, not what they looked like externally. And then we could give them targets like how to decrease, like in a good way, make younger their heart health. That could be through exercise or whatever, but it could also be through products that Unilever offered some of the food brands that Unilever offered.
So you, there's definitely behavior change techniques and things that you can do to help people live better lives as well.
[00:38:01] Erin: Awesome. Uh, Okay. so here's another one. I think this one is maybe controversial, I guess all of these could be, but UX research equals qualitative, qual. True or false, it depends. What you think?
[00:38:16] Zach: I think it's well-meaning. I think the people who are saying this are generally like, you know, I'm part of the gang. I know. I know research too. And I just, I want to do something with you. Let's do some qual. It's not always a qual though. And I think that's a fairly obvious answer. A lot of the time what we actually end up doing is quant. Or it's a combination of qual and quant or it's something else maybe it's work.
Maybe we don't even need to do research. Maybe we'd go and talk to a data science team and we get behavioral data. Maybe we do a literature review. I don't know what it is. There's all sorts of ways that you can get decent answers. And so, I would like to banish let's do a qual from everyone's phrase and here are two alternatives.
You can say let's do some research or let's talk to the research team and figure it out. Right. So I think let's just take the easy route on this one. And let's just talk about quant methodology.
I'll give you an example. Also at Unilever. We were always trying to create like a new lotion I wanted, I want something great.
I want something that hits a white space essentially. So one way you could do that is you could whip up a few new formulations. You could go and talk to some people and have them try them and they can tell you if they like something and dislike something and you can run into some of those earlier problems we were talking about where, you know, seven of the eight people I've talked to thought this was the best one. So let's launch this one into the market.
You could go down that path. It's not a great idea though. With this, you can do some really cool stuff with quantitative research, and I'm going to talk for a couple minutes about landscape segmentation analysis. This is a really cool method that we did at Unilever, where you take something like a lotion and you break it down into its sensory areas.
So you score it on how sticky it is, how fast absorbing it is, how oily it is, how greasy it is, all sorts of things like that. Right? And then you find lotions that cover that sensory space. That's a multidimensional sensory space that covers different combinations of all of those attributes. And so you're doing the hard work to find a subset of stimuli that give anyone who tries this group of lotions, a sense of all of the dimensions in the extremes of all of those dimensions.
And you send those out, let's say like seven motions. You send them out to people. They use them for a week and they give you one score. They say how much they like it.
Like whatever point scale, 7.9 point scale. And then what you do is you take all of that data and you stick it into this landscape segmentation. And now. That shows you where clusters of people fall in relation to not just the lotions that you showed them, but all of the combinations of attributes that could make up future lotions that you didn't actually show them.
And so your goal with this analysis is to define the clusters of people that fall in a space where there isn't a competitive lotion where there isn't a Unilever lotion. It can be defined by a combination of attributes that you can use to create a new lotion to hit that white space. Right? So instead of saying, like, let's try these five and a qual it's, let's try this massive existing set that pushes the boundaries of what could be a lotion.
And then let's come up with a list of attributes that then describes what people actually want and turn that into a new lotion.
[00:41:40] JH: Nice. Yeah, that's cool. That, that feels like a type of technique where you definitely need somebody who's pretty well versed in that type of analysis. Right. Cause you're getting into some pretty sophisticated stuff in terms of the study design and. that it does feel like there's stuff like that where you just get the whole, I guess the landscape is a very literal word in that sense, you really do get a grasp of what the space is like and where you might want to concentrate future efforts and attention.
[00:42:01] Zach: yeah, that's actually that's right. It doesn't have to be that complicated. There's a lot of tried and true marketing techniques or marketing research, quant techniques, like a conjoint, discrete choice, a turf analysis that you could that are fairly straight forward, you know, survey techniques that you can get an answer within a couple of days that aren't directly asking people, you know, which one do you want?
They're giving people choices and they make choices. And then you do the complicated part of the analysis on the back end. That helps you to define what the optimal choice would be, the optimal combination of options together that you should launch on the market.
[00:42:38] Erin: When you genetically engineer the perfect lotion. Right. And I've got a couple on my desk and I'm thinking I need to apply some. But when you know, you're like, well, they like this characteristic and this, and there's these clusters and you put them together into the perfect lotion for this group of people.
Not for everybody. Does it like work or do you like actually that's a Frankenstein when you put it all together and actually they like all these characteristics, but together it's a mess. Like is there just salt to a perfect lotion? You know, and like, is there an MVP for a lotion? You just put it out there and see what happens and try it again.
[00:43:12] Zach: That's a really good question. You can find attributes that are really the main driver of the location of these groups, of people, these white space people. And so like, hey, we've got this one key attribute and that's the one that really defines this group and pushes these people away from what is currently being offered. How can we dial up that one attribute in our lotions to really move the people that want to be there down to that space?
[00:43:41] Erin: interesting.
[00:43:42] Zach: Yeah, I mean, the, another, the other example that I was going to talk through was around turf analysis, which is a fairly straightforward technique that you wouldn't use a qual for. I mean, you could use a qual for, if someone came to you and said like, Hey, I've got a $2 million budget to spend on TV advertising.
What should I spend it on? Where should I put it? You can do a fairly straightforward, easy study where you send a survey out to people and you ask those people to be people that are targeted for your brand. They can be people that you want to be using the brand, your brand, but they haven't started using it yet.
I mean, you can work on whoever the target is, but it's a fairly straightforward study where you just ask them, okay, I'm going to list off 10 TV shows and you you rate how interested you are in their shows on a scale of one to five or how likely you are to watch them, or some other attribute on a scale of one to five.
And then you take the data and you do all the work on the backend because ultimately you don't want to take your $2 million. And spend it all on a combination of three shows that everyone watches, even if they're the most popular shows, because you're basically just repeating your message to the same people three different times.
What you need to find is the combination of the right number and type of shows that will maximize the reach to which your advertisement gets out to in this population. So it's often not the top two most popular top three most popular shows in your list. Oftentimes it's the most popular one.
And then something that's entirely different from that one that really brings an additive audience. And that will come out of that analysis. So what I'm trying to say is like, in these cases, like, when you say, when you come to me and say, let's do a qual, how should I spend my marketing budget, or let's do a qual.
What kind of new lotion should we create? That you should come to us and say, like, let's do some research. I need to make a new lotion. Let's do some research, help me spend this marketing budget more effectively. And then we can work with you to define what that might look like.
[00:45:44] JH: Nice. This is a very stupid, observation, but I think there's something about this phrase. Just let's do some qual that sounds cool. So maybe we just need to be like let's do some re or something. Like maybe we just need to give it like a little more pizazz so that people are lean into
[00:45:54] Erin: Yeah. Yeah. I'm imagining a person who's really enlightened. They're like I'm open to qual,
[00:45:59] JH: It just sounds calming. Like qual has a nice, like
[00:46:01] Erin: Yeah, it does. It's soft. Yeah well, it's been really cool to hear about all your depth of experience across so many different research use cases and companies. Thanks so much for joining us.
[00:46:14] JH: Yeah. It's Super fun when it's examples. Everyone knows. Yeah.
[00:46:17] Zach: Yeah. Thanks a bunch for having me. I had a lot of fun thinking about all of these interesting experiences that I've run into over the last year.
[00:46:25] Erin: Yeah. Let me ask you a question I like to ask folks, which is just based on your experience, what do you love most about doing research? What do you love about UX research?
[00:46:34] Zach: Oh about re?
[00:46:36] Erin: Not qual.
[00:46:37] Zach: I, this is an easy one for me.
It's always been making some kind of difference or some kind of impact and seeing people or some population benefit from the change that research uncovered. Uh, So if you want to go all the way back to the beginning of the conversation, if I had done research, I had created a car instead of a faster horse, like I would be super proud of that.
And I would always know. We helped somebody. We helped somebody get somewhere faster or we helped somebody be more safe or something along those lines. There's lots of fun examples of things that you use everyday that you have no idea that I worked on, or my team worked on at some company that I used to work at. it makes me really happy to go to sleep at night, knowing that you have gotten those benefits.
Creators and Guests
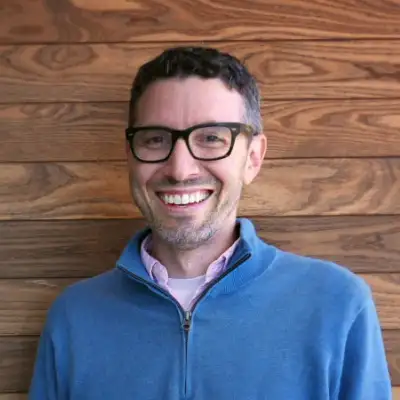