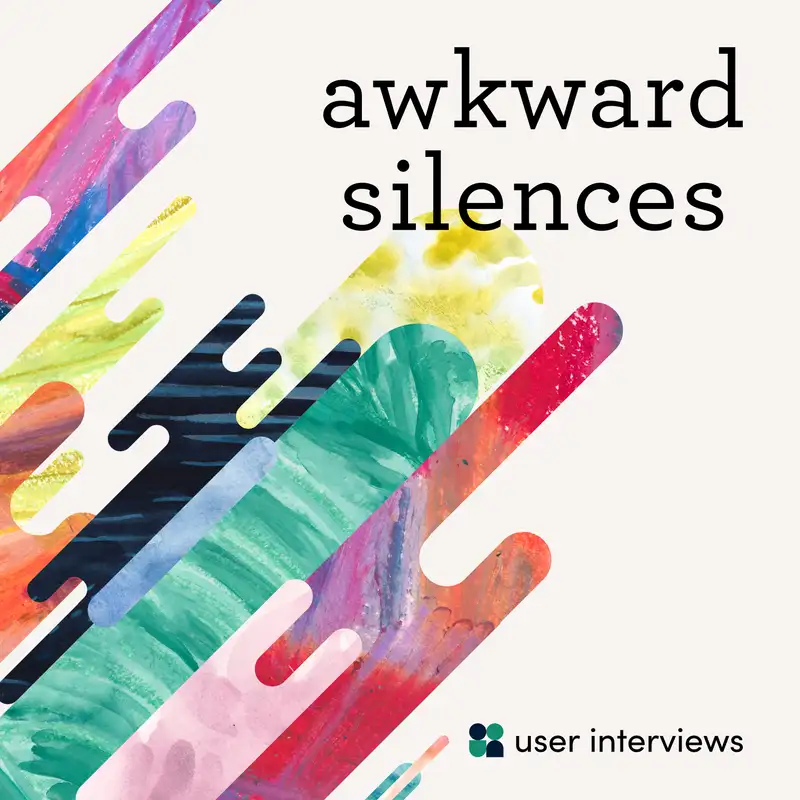
#51 - Uncharted Territory: AI & User Research with Hana Nagel
Erin: [00:00:43] Hello everybody. And welcome back to awkward silences. Today. We're here with Hana Nagel. She is a design researcher at element AI. And today we're going to jump into a new topic for us. A I so are going to talk about artificial intelligence and the unique role user research can play. When, learning about artificial intelligence and in particular, how we can think about AI and the ethical implications and how user research can help us to build moral, ethical products.
So thank you, Hannah, for joining us to talk about this very interesting topic.
Hana: [00:01:26] Yeah. Thank you for having me.
Erin: [00:01:28] Got JH here too.
JH: [00:01:31] Yeah. I'm somewhere between embracing and being ready for our robot overlords and like wearing a tin hat. So I'm figure out where on that spectrum. I actually am. I think this would be a good combo.
Hana: [00:01:40] Sliding scale.
JH: [00:01:42] Yeah.
Erin: [00:01:43] Always. So let's jump in user research in AI. We want to talk about some of the moral challenges that can present themselves. When we think about, letting those, are they chaotic good or neutral evil, or whatever sort of robots we're dealing with. Let's talk about what some of those challenges are and how user research can, can help us to make sense of that.
Hana: [00:02:10] Perfect. so one thing that I would say, when we're thinking about this concept is, Always the starting point for me is defining the ethics, of what like a responsible artificial intelligence system might be. and when we're looking at these systems, I find it helpful to think about operationalizing those ethics.
Rather than a philosophical discussion about morality. So how can we actually put, into practice what it means to have kind of a responsible, ethical, fair system? I think one of the kind of initial challenges in this space is that the kind of standards and the best practices are very much still being developed.
And because the technology has been moving so fast, it's been a collaboration between, between private enterprise, a civic sector, governments, et cetera. and then academia is of course putting out a lot of work around that as well. so coordinating those efforts to understand, from those different viewpoints, what do we actually mean when we're talking?
Think about an ethical system.
Erin: [00:03:13] how do you use your research? AI? I know this is not like a monolithic thing, But what are practically, what are some of the things you do to figure out what the, what a good experience is going to be when it comes to this pretty. Nebulous big data, We think on the one hand and these, I think we need robots, To have something tangible to point to, but how do you, what are some of the methods and tools that you're using and your work as a design researcher?
Hana: [00:03:45] Yeah. Great question. so the first thing I would want to, just differentiate something that I heard you both say, we're talking about robots. I want to differentiate between kind of the software and the hardware element here, which can go together. Of course, when we're looking at, Maybe, in home devices, or there are some actual, robots that are in hotels, for example, that will bring, deliver food to rooms, et cetera.
and then we also have this kind of like software, the model component, which has its own unique set of challenges. and I'm going to focus more on kind of the model side or the software side of artificial intelligence.
so I would say in terms of the differences and the challenges of researching, AIS, as opposed to maybe other kinds of software services, One thing that we're looking at that as similar, of course it's the kind of the needs and the pain points and the challenges, of our users.
and so we are using, methods like observational interviews, What kind of semi structured interviews and design the feedback or usability testing sessions, in the enterprise space. At least a lot of the folks that we are working with are already using some sort of enterprise system that is using, AI.
And so they do have some level of familiarity with it. How to use a new system? I think one of the, one of the challenges of AI, is that it can be hard to, it's hard for users or for humans really, to imagine. Using something that doesn't yet exist. and so when you are presenting a new tool, and asking, trying to get a sense of, what their comfort level might be with it, how they might have, unintended or unexpected uses of it, it can be challenging to get that kind of insights when the product isn't fully built and doesn't have all the data behind it.
I think one of the famous examples is when. they were going to launch the iPhone and Apple hired this external marketing company to send out this massive survey. And, they're asking people like, would you like a device? So that was like a camera and also, music player, it could also access the internet and people were like, yeah, I don't you'll really see the need for that.
That doesn't really seem helpful. it's really hard to situate ourselves in an environment where we're thinking about a completely new tool. and it's also challenging for people to really understand the impact on their day to day life of. How this tool might, go about collecting and using data.
And I think that's a big part of these ethical systems and we're thinking about it. if we're trying to get a sense of, for example, would you be interested, you said, in a tool that monitors the environment in your house and adjust the temperature accordingly and then, maybe lowers your electricity bill.
And that sounds great. When you're explaining that part of that is maybe monitoring personal health, and tracking those records herds as well. That might be too far for some people, and getting people to, fully understand like the breadth and depth of the data inputs that are needed in order to get the data outputs that they want, can be challenging to do.
JH: [00:06:43] Yeah, it seems in addition to like your point about, it's hard to imagine, using this recommender model or something and liking that it's giving you a good song recommendations or something it's hard to, for people to picture that it seems hard for people to also recall. If they enjoyed their interaction or something with, I'm just thinking like recommending stuff.
if you're asking me about Netflix and you're like, Hey, the last time you were on Netflix, did you like the record? I'd be like, I don't know. Maybe like how do you even know when people are able to interact with a model? How do you engage with them to get the right insights about like their experience with it and whether there were issues ethical or otherwise.
Hana: [00:07:19] I'm going to go back to one of those challenges of data collection, when we're thinking about our metrics for an ethical or responsible system, and trying to define those metrics and like the process for achieving those metrics, and how we're going to validate those targets are achieved.
Wrapped up in that is this concept of transparency and explainability and accountability. and these are essentially ways of showing a human user, how the model, how and why a model kind of reached a decision? there's a couple of different kinds of explainability. And there are some they're in different and levels of maturity in terms of how accurately, a model can explain to a human user, how and why, an event was reached.
and I think part of that is, to go back to this recommendation question, How can we explain to our users why something was recommended, and show them what might've happened with different pieces of data were collected or what pieces of data that's being combined with in order to make, better or different decisions.
Erin: [00:08:24] what is the ethical imperative around transparency? Because similar sort of to some of the security things we talk about there is this maybe trade off between. Just tell me what I need to know and that's it. Cause I'm trying to get something done and it's not going to be usable. If I have to read, your 30 page training manual on how you train right.
The software to do whatever it's doing. And maybe I don't really care on the other hand, depending on the sort of software it is. maybe I really care how, so this decision was reached and I need to know if I trust it or not. If I can. mess with the inputs myself, to tweak the algorithm and what's happening there to make it really work for my use case.
And I imagine for different use cases and different users, there's quite a variety on that spectrum in terms of how much do I want or need to know about what's really happening here. So how do you navigate all of that?
Hana: [00:09:24] Yeah. And I think that navigation is really a challenge that as in industry, we're really starting to confront. and I think that, users who have different types of these systems, are also starting to really be confronted with what is their level of comfort in what kind of data has been collected and how it's being used.
I referenced like, And nest, as a system that is collecting data inside the home in order to make decisions about temperature. we've also been seeing that systems, like Alexa or Google that are, recording within the home. and what. Some folks are not realizing is that there is a human in the loop there.
so there are folks, I forgot the percentage, it is a small percentage of these recordings, but there is a percentage of recordings which are actually sent to human transcribers. and that is to just keep the model, on track and make sure that it's being accurate. and so there are.
And a certain percentage of conversations that is small, but there are a certain percentage conversations that are real human is listening to. and maybe you were just talking about, do you need to order more milk? but maybe you were having a very intimate and heated discussion with a partner about something that is very private.
and that yeah, information has been reported and no longer belongs to you. That data does not belong to you anymore. And there's no privacy for that. A lot of folks, I think whether they don't work in tech necessarily, but even some in, in this industry, like we don't always think about what our comfort levels are.
and what that trade off is, what level of, ease or kind of optimization are we willing to, Get in order to give access to our own data, whether that's our body temperature or conversations in the home that we might want to be private. what is our level, in navigating that and understanding the impact.
JH: [00:11:51] It feels so tough because it feels like it's one of those things it's often like it's okay until it isn't right. I don't mind you recording me. Cause I like being able to add stuff to my grocery lists really easily and play music in the kitchen and stuff until like you have a fight or something in your house and you're like, I didn't want that recorded.
So like, How do you get people to think about it, it holistically to understand like where their comfort level is, so that they can have more of a input or control over that.
Hana: [00:12:15] I think that this part of it, the input and the control, this is something that a lot of work needs to be done in our own industry, to define those metrics, and define those features. so when we're thinking about transparency, explainability, accountability, based on our principles that we are putting into practice in this, to what extent can we show our users in an explainable way, in an understandable way, what those trade offs are?
a lot of these models and systems are very complicated and convoluted. They have a lot of moving parts. there's a lot of teams that are working on these products. some of them are consultants, some of them are outsourced. and so the accountability is really spread out over a wide network, and pinpointing where to start.
I think is, so part of this process of like, how do we define and operationalize an ethical system? I think there's a couple kind of big examples over the last year or two. when we look at, for example, the Boeing 2019 crash, or a couple of years ago, the Uber, automated vehicle that crashed into a cyclist.
and these are some really great examples of how we need to start thinking about accountability as part of this answer to who is responsible for explaining to users. What information has been collected in order to get an output and what control the user has over different parts of a system.
Erin: [00:13:42] The stakes of bad usability. And some of the cases you just mentioned are really high adding a huge ethical component to making sure not only do users know how to use these tools and softwares powered with AI, but that they actually in fact do that and know. Where the metaphorical robot capabilities begin and ends.
and I think that's really interesting when you think about right human computer interaction, it's this whole new level of that, of. what exactly am I as a human responsible for now? What exactly actually are you the computer responsible for? And if everybody just throws their hands up, what am I the creator of the computer responsible for?
Are there starting to be standards? You talked about some of the standards in terms of ethical outputs. We can strive toward transparency and security and so on. are there starting to be standards in terms of who is accountable?
Hana: [00:14:42] I think that we've a way to go before those standards are implemented in kind of a government space where regulatory things.
There's a really great paper by Madeline Claire Eilish. She wrote a paper called moral crumple zones, cautionary tales, and human computer interaction. and one of the things I love about this paper, which actually references that Uber crash is, who is responsible and what is the level of control that we are giving to humans versus systems.
and from the kind of company standpoint, one of the things that is put forward in this paper is that. The human operators are the human in the loop. They're being put into what she brings the moral crumple zone. So in a car, a crumple zone, is that area that kind of absorbs the cracks. Gosh. and we know that, and an argument that she's putting forward is that, the responsibility for the impact of these systems is being, perhaps unfairly put onto the human in the loop, the human operators.
when it might be who best to go back and take a look at the ways in which the system, is actually the system at large, is actually responsible for a lot of these, buildup of decisions and the humans really just the end of it. And one of those kinds of regulatory challenges is this systems are built by a lot of people.
we have, the execs that are signing off on things. We have the managers, we have, the people who are actually coding and designing it, in the case of the Boeing crash, for example, some of that code was built internally. Some of it was outsourced to consultant and other areas. and so there's this.
Kind of convoluted sense of accountability, that it can be easiest to just say, Oh, the it's the pilot it's fault because they crashed the plane and it was the human monitor's fault in the car because he wasn't paying attention. but. What was the system doing to keep their attention? what was the system doing to show, updates and, statuses, et cetera.
And what kind of responsibility does the code have, or does the company have, who produced that code? in terms of, inputs and outputs.
JH: [00:16:43] Not to go like full trolley problem. But I think the thing that makes this so complicated is if like humans driving cars without any eye AI involved at all right. Get into accidents and, there's really unfortunate outcomes there. And if we're able to say with some high degree of confidence that we could lower the accident rate by, adopting a lot more self-driving or assisted driving or whatever it is, there's, you can make a decent argument, That there's a moral imperative to pursue that because it could actually help us be safer in like some aggregate way. But I guess the part that like, maybe just feels challenging about that is if you do have all of these automated drivers out there and then a bug or an issue gets shipped, the ripple effect.
There can be enormous in a way that like not, everybody's gonna wake up tomorrow and be like a bad driver. and so I just don't like, how do you disconnect that part of it? Cause it seems like there that's. I think the argument that people that really are proponents of this stuff tend to make right.
Is that in aggregate, we maybe could be much safer in cars than we are today.
Hana: [00:17:41] So I think part of that is asking questions about how do we enable it to be safer, when we're looking at models that are being deployed and kind of these test environments, what is, how closely does that replicate a real life? Driving experience. you are on the road, for example, in this Uber crash case.
How often are you actually paying attention to literally everything going on the road? what is a human response time to a cyclist coming out in front of a car, as opposed to the model response time of a cycle is coming out in front of a car. When you are, driving a quote unquote regular car, it's, only happens I'm unfortunately that, cyclists will come out on either side.
and if you weren't looking in that direction, it's very easy to crash into a cyclist. so if we're just, continuing down this path, really asking companies to break down that argument and show in a range of situations in the dark, in the snow, when there are children fighting, when you.
Reach for a coffee. In what ways is the system accounting for that range of human behavior and still maintaining that kind of consistent output of safety?
Erin: [00:18:54] And this is one too, where it seems like user researchers have such a unique role to step in and. So user researchers don't get to just work on right. W whatever aspect of the project they want necessarily. but I imagine let's use the plane example. There's, dozens, hundreds, thousands of mini software releases and hardware decisions that go into making a plane like that.
I can't even fathom a lot. so it's not like when user researcher is just gonna, I'm gonna, own go ahead and own this. Clean release. But, it does seem like. you could be in a unique position to think less myopically and more bigger picture in terms of things that might go wrong.
On the other hand, that's a huge burden to put on someone to think through all those doomsday, worst case scenarios. And yeah, it's really interesting situation. We're in here with all this, to what you said early in the conversation. People can imagine something that's never existed before and that's happening.
Every day, all the time with AI in ways, big and small. and I think, not to get philosophical, Cause for being practical here. But, I don't have a question. I just think it's really
JH: [00:20:10] Yeah. Yeah. I have an idea maybe to try to get us in like to more concrete stuff is let's imagine, Aaron and I came up with some great idea that using a bunch of the sensors on a phone, the microphone and the step data on the gyroscope stuff. Like we think we have a way to blend all those things together to make really smart health predictions that can be helpful to users.
And, So we're going to consume all this personal data off their device. If they install our app. And we think we can give them really good recommendations about when they should see a doctor or whatever it may be. and we're like, all right, let's go start this company. What are some of the things we should do upfront, or like questions we should be asking ourselves to avoid like some of the lurking ethical concerns that are like, going down a path like that.
Like what are the things you would tell us to get ahead of, or to look out for?
Hana: [00:20:53] Yeah. So I think one of the initial steps will be to think about what features, what kind of ethical and responsible features of your system you are going to plan for. so what kind of, how would you define ethical, responsible system performance? what kind of explainability? the model, produce, How can you assess whether humans are understanding, the different, explainable options from the model, what kind of data is being collected and from what source, what kinds of biases might be built into that data collection process, that are going to maybe impact the output of that model, security, where is that data stored?
Who is it shared with? and how are users understanding, W what that data collection and storage kind of looks like. and then we have more of the operational side of the metrics. so re mentioning like the metrics for the feature is a process for achieving. how are you going to validate if those targets are achieved?
those are, I think some of the starting points that we would want to think about. one of the, one of the challenges I think, across the board is of course data, which is really like the, one of the fundamental areas of these models. humans, construct these frameworks for collecting data.
and so there are oftentimes issues with the data sets that have already been collected, and understanding the extent of the bias in that data. I think is one initial step as well. There was a British healthcare company. I think it was like a, it was like a medical chat company, which I'm forgetting the name of right now.
And it would essentially, one of the screener questions would be like, what is your gender? And then yeah. Describe your symptoms. And it would tell you whether or not to go to a doctor or the emergency room, et cetera. and because, there's so much bias in medical care, systemically, one of the inputs, one of the outputs that we saw there was a deep, gender bias, where.
Men would, describe a certain set of character. We'll be told they're having a heart attack. whereas, female patients were told that they just needed to calm down, hysterical basically. and the only difference there was the gender marking. that's just one, One example of how biases, we're living in a system, a social system that has, deep systemic biases built into its operation.
and the ways in that's reflected in the data that we collect, has, a range of impacts. Now that we are, asking models to be responsible for some of the decisions that a person might make. we can't offload some of that responsible task to the model when it's consuming data that we create Doost as a biased system.
Erin: [00:23:30] Is it ethical for AI to try to overcompensate for human bias? So it's similar to the idea that. an AI enabled smart car can save lives and drive better than humans can drive. That sounds pretty good. we know that humans are subject to all sorts of cognitive biases to, accidental or intentional racism, sexism, et cetera.
And so those things, we see those and. In the training and the machine learning of et cetera. So if we say, okay, as a society, let's try to eradicate all of that human biases from, is that the right thing to do? And can that be done? And can you, is that somehow bad? And the unforeseen way that to suppose that there's a neutral position versus a biased one, and who would get to decide that.
Hana: [00:24:24] Yeah, that's a good question. I think on one hand, I would want to point out that not all biopsies are damaging or bad.
Erin: [00:24:32] Right.
Hana: [00:24:33] by simply means being a, slanted to one angle or another. and that sometimes those can be good and have a positive impact. so part of this work is determining what is it, the impact that we want to have, and is our current structure, getting us there, in terms of asking models too, Identifying account for those biases.
part of the work on our side is noting the biases that we have. the model can not necessarily. Identify biases that we're not aware of. either, I think when non AI example of this to try and, take a deep dive into this concept is that Boston orchestra example, and then I think the 1960s, I forget the name of his experimentation.
I've pulled it open before, but, essentially there was a challenge, where, orchestras, all were overwhelmingly male, and. That may or may not be a problem. So the first step is to say, do we want orchestras that are all male? Or do we want orchestra, Which are composed of the most talented players.
if answer B, then the next step is to assess whether or not, our data input. So like our applicants, are being assessed correctly. and so essentially what they did in that experiment was to, con. Bring in a series of kind of blind approaches. So first they put up that screen, so they couldn't see the applicant.
but there is still that auditory cue, of the, heels clicking on the stage. and once they, brought in a double blind layer of putting a carpet down, I think it was something like 35% more, Female applicants were brought in, which is actually quite a high amount because there's not that many new orchestra members being brought in every year.
so that's one example in a real life example of their goal was to have the best. Inputs possible. So the best players, because of their own bias, two words, probably white men. they were ending up with an orchestra, which was composed of, a bias, which actually didn't reflect their goal.
and so they had to start breaking down and identifying their own biases before they could, Kind of rearrange their data input in terms of like applicant stream. and that's something that we need to do as well when we're looking at models that are, doing a range of things, assessing credit, worthiness, determining where to roll out a feature.
all of those things are going to be, all of those models are going to be drawing from data, which is collected from biased systems. we're looking at things like credit worthiness, A lot of that has been shaped by racist practices. Yeah. I'm in both the United States and Canada around who was allowed to own homes, and who has been systemically denied access to that.
and when that's tied to things like, for example, Amazon rolling out their prime feature, and they were, using historical kind of zip code data, which really reflected. areas where blacks weren't allowed to own homes in America. so we're seeing this kind of consumer feature, That is based on seemingly like innocent quote unquote data.
But that data is actually really rooted in longstanding systemic biases and discrimination. and so we have to take a couple steps back and say, what, how would we define. Ethical here, how would we define, a nondiscriminatory approach? and then how does our own team play into this? How does our data sourcing play into this?
and then what can we do differently?
JH: [00:28:02] Yeah. There's so many layers. I feel like the thing that, for me, that makes the machine learning, model stuff, like giving them control over, who's qualified to be in the orchestra or whatever. Is not that like we're perfect. You just gave a bunch of awesome examples of how flawed humans are at these same things is that it feels like the risk of I'm a model or something like machine learning based for it to be like a runaway freight train where like it takes a bias and like really amplifies it or really goes nuts.
Just feels like at a different scale than maybe what humans could do. So like humans while we're very flawed for all the reasons you just pointed out. Are like maybe slower, both to fix things or make them worse. Whereas it feels like the machine making that distinction about who should be in the orchestra or whatever could like really accelerate it for good or worse.
does that seem like a fair concern?
Hana: [00:28:51] Yeah. I think one of the concerns there is just like our systems trust, where we're like, it's just the answer. It's not, it's not a biased answer and not understanding how the inputs are getting us today. Like one thing, for example, that I see a lot on Twitter, for example, in like the Twitter fights that happen is that you'll see people, Try and back up an argument, with, global warming, for example, by what they found in a Google result.
and there is it seems like a deep misunderstanding that the results that they're seeing are actually tailored to their own history and preferences. It's not the answer. It's an answer that reflects a range of inputs. and I think when we're looking at. how artificial intelligence is machine learning models are being implemented in a range of systems.
and we need to ask ourselves questions about, what were the inputs that really got us this output. and that's really the explainability portion. and the human interpret human interpretability. Pardon me to say, the ethical practices putting into practice when an ethical, unfair system is.
Erin: [00:29:56] So not to ask the where's the world going predict. Predicted question, but yeah. where do you think this is going? And it's the emerging fields are always, I dunno, it's really slow. And then all at once, right? we've been talking about the self driving car for a while now, and it seems to have slowed down.
And I don't know when we're all going to be not driving autonomous cars, but how are user researchers getting more verbal with figuring out how to work with AI technology? Is it. Because it's new and evolving, going to continue to be that way even more. So for the foreseeable future or.
Hana: [00:30:36] Yeah, I think one thing that I'm starting to see more of that as researchers, we're looking more at, the holistic sense of systemically, what does it take, to get a fair and accountable system in place? I think the. Car driving example is actually really great. where, five years ago, people were saying we're all gonna be in these, self driving cars.
and now it's looking more like it will be, Long haul trucks, for example, a part of that is what is the environment that allows that to be safer, and really breaking down, these cars are then driving on long stretches of highway where they don't need to navigate around a lot of different objects.
And so the safety there, is clear, Whereas, if you're in an urban environment where you're navigating around different cars and bicycles and traffic, that's maybe not an ideal environment for, for an automated car, or self driving car. and I think this kind of holistic sense, like what, not just are users, the individual user, but what are the characteristics of their environment that they operate in?
whether that's other people, other systems, either, habits, et cetera. What are the characteristics of that environment that we really need to account for? When we're thinking about building and researching these systems?
JH: [00:31:52] Yeah, your job seems hard. It seems really hard to juggle all this stuff. but it seems really important and it seems really interesting. So I'd imagine it's very satisfying, but, it seems so many different factors to juggle and balance all at once.
Hana: [00:32:05] Yeah, I think in my dream world, which I think will become a lived reality soon. Like this work will be shared among a range of expertise. so there will be design researchers, but really doing, close, collaborative work with other kinds of systems, specialists, social system specialists, et cetera.
as we flesh out those unknown unknowns and turn some of them into known unknowns, I think it would be taking, a range of collaborations for us to deliver these systems that have, an ethical and fair impact.
Erin: [00:32:34] Yeah. And so much of this is applicable to not just AI and we didn't really get into what is AI, but as software eats the world, everything seems to have a twinge of AI in it. these kinds of questions, ethical imperatives will become relevant. To use our researchers everywhere. And to your point to cross functional teams of well meaning humans who are dependent on technology increasingly.
Hana: [00:33:01] Yeah, I think a lot of our decisions, when we're using a certain piece of software, in order to enable decisions there, that output, that final decision is. Based on other decisions that are maybe out with two different models. so for example, you might use, Google maps to get to a place and then use a different application, to make another decision.
and so trying to look at that framework of applications, and understand, the inputs and outputs at different points that you want to focus on. I think is part of the, what are the challenges, but also one of the exciting things about this field,
JH: [00:33:37] Yeah, no, it seems like, there's so much excitement in terms of, if you're able to actually shift some of this stuff in the right direction, the impact and the scale is huge and super exciting and hopefully a real net positive.
Hana: [00:33:48] Yeah, I think so. I am really hopeful and I'm seeing a lot of, really, a lot of cool articles are being written. I think both from, use cases from, in practice and also academia. and I'm also seeing a lot of, collaboration between kind of, enterprise and, academia that I think is really important.
So it will be really cool to see where that goes as well.
Erin: [00:34:08] we'll be here waiting.
JH: [00:34:12] Yeah. hopefully this episode gets picked up by whatever algorithm recommendation and get up in that viral loop.